Traffic Light Recognition With High Dynamic Range Imaging and Deep Learning
IEEE Transactions on Intelligent Transportation Systems(2019)
摘要
Traffic light recognition (TLR) detects the traffic light from an image and then estimates the state of the light signal. TLR is important for autonomous vehicles because running against a red light could cause a deadly car accident. For a practical TLR system, computation time, varying illumination conditions, and false positives are three key challenges. In this paper, a novel real-time method is proposed to recognize a traffic light with high dynamic imaging and deep learning. In our approach, traffic light candidates are robustly detected from low exposure/dark frames and accurately classified using a deep neural network in consecutive high exposure/bright frames. This dual-channel mechanism can make full use of undistorted color and shape information in dark frames as well as the rich context in bright frames. In the dark channel, a non-parametric multi-color saliency model is proposed to simultaneously extract lights with different colors. A multiclass classifier with convolutional neural network (CNN) model is then adopted to reduce the number of false positives in the bright channel. The performance is further boosted by incorporating temporal trajectory tracking. In order to speed up the algorithm, a prior detection mask is generated to limit the potential search regions. Intensive experiments on a large dual-channel dataset show that the proposed approach outperforms the state-of-the-art real-time deep learning object detector, which could cause more false positives because it uses bright images only. The algorithm has been integrated into our autonomous vehicle and can work robustly on real roads.
更多查看译文
关键词
Image color analysis,Machine learning,Cameras,Lighting,Image recognition,Robustness,Histograms
AI 理解论文
溯源树
样例
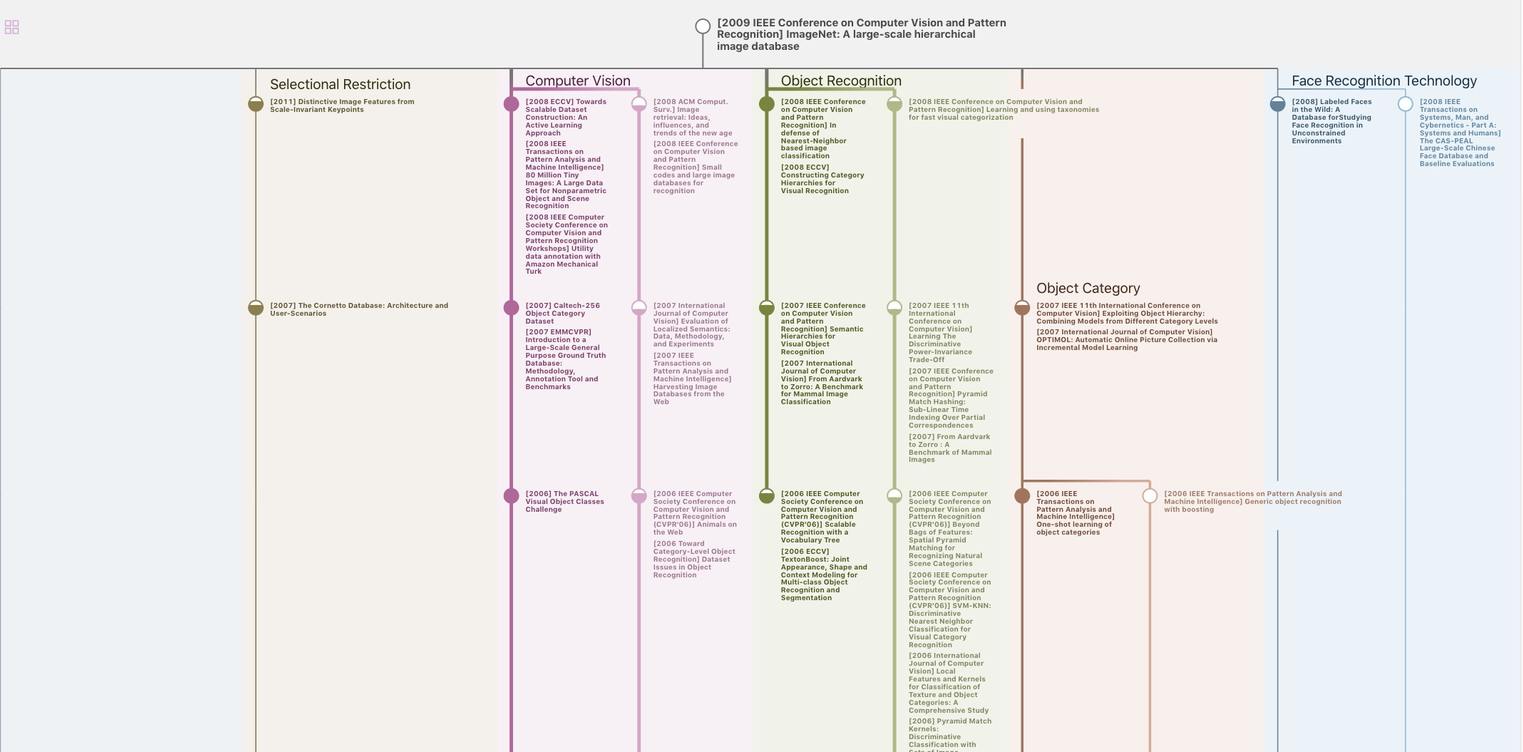
生成溯源树,研究论文发展脉络
Chat Paper
正在生成论文摘要