Incipient bearing fault diagnosis based on redundant dictionary pruning
2018 IEEE International Instrumentation and Measurement Technology Conference (I2MTC)(2018)
摘要
Incipient bearing fault detection has great significance for mechanical condition monitoring. One of the challenges is to extract the impulse features under high background noise. Recently, the sparse representation theory has made tremendous progress in removing noises and vibration feature extraction. The fault characteristics are adaptively extracted from the noisy vibration waveform by means of the dictionary learning methods. However, under strong noise, the learned dictionaries contain a number of noise atoms, which influence the reconstruction performance of the impulse features. In order to address this problem, we propose a redundant dictionary pruning method to suppress the atom noise. It applies Lilliefors hypothesis test to judge noise atoms after the dictionary learning procedure. The effectiveness and robustness of the proposed method are verified by the numerical simulations and the wind generator incipient bearing fault diagnosis.
更多查看译文
关键词
sparse representation,dictionary pruning,bearing fault diagnosis
AI 理解论文
溯源树
样例
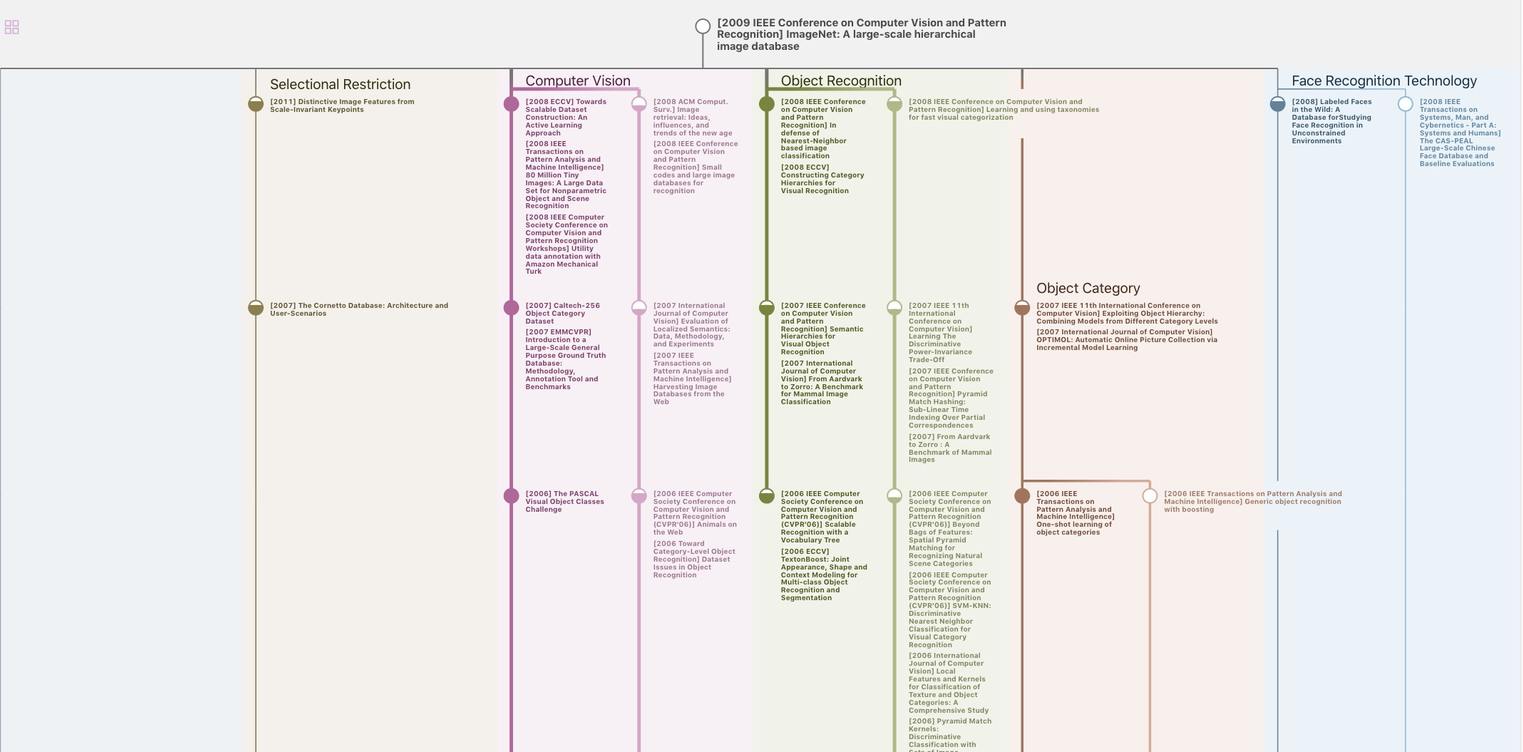
生成溯源树,研究论文发展脉络
Chat Paper
正在生成论文摘要