Convolutional structures and marginal statistics--a study based on K-nearest neighbours
Journal of Communication and Information Systems(2018)
摘要
This paper addresses statistical tricks found in deep convolutive neural networks. First, the most relevant statistical tricks are studied under the perspective of data scarcity, then one of them, directly related to convolution-like structures, is regarded as a random variable marginalization. The same kind of marginalization is implemented in an ensemble of K-nearest neighbours cells, where each cell yields scores instead of class labels. Scores are then combined to improve classification accuracy, as compared to a conventional K-nearest neighbours classifier in experiments with two emblematic datasets---MNIST and CIFAR-10. This improvement is regarded as evidence of the variable marginalization effect over performance, whereas it is discussed the potential for further lessons learned from deep neural networks to be transferred to KNN based classifiers, whose advantage is to allow for explainable artificial intelligence.
更多查看译文
关键词
convolutional structures,k-nearest
AI 理解论文
溯源树
样例
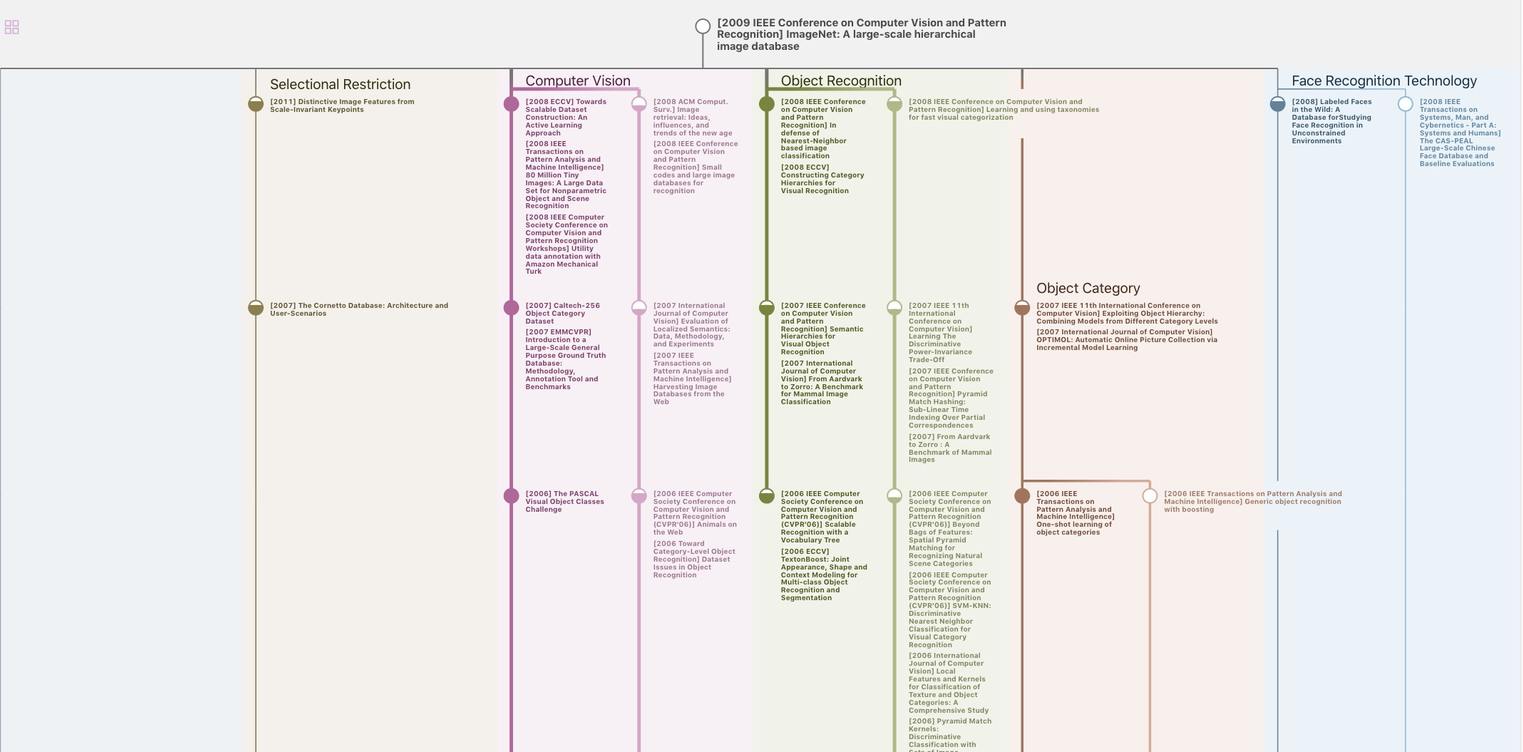
生成溯源树,研究论文发展脉络
Chat Paper
正在生成论文摘要