Efficient Near-Infrared Spectrum Detection in Nondestructive Wood Testing via Transfer Network Redesign
SENSORS(2024)
摘要
This study systematically developed a deep transfer network for near-infrared spectrum detection using convolutional neural network modules as key components. Through meticulous evaluation, specific modules and structures suitable for constructing the near-infrared spectrum detection model were identified, ensuring its effectiveness. This study extensively analyzed the basic network components and explored three unsupervised domain adaptation structures, highlighting their applications in the nondestructive testing of wood. Additionally, five transfer networks were strategically redesigned to substantially enhance their performance. The experimental results showed that the Conditional Domain Adversarial Network and Globalized Loss Optimization Transfer network outperformed the Direct Standardization, Piecewise Direct Standardization, and Spectral Space Transformation models. The coefficients of determination for the Conditional Domain Adversarial Network and Globalized Loss Optimization Transfer network are 82.11% and 83.59%, respectively, with root mean square error prediction values of 12.237 and 11.582, respectively. These achievements represent considerable advancements toward the practical implementation of an efficient and reliable near-infrared spectrum detection system using a deep transfer network.
更多查看译文
关键词
CNN,domain adaptation,near-infrared detection,nondestructive testing,transfer learning,wood material analysis
AI 理解论文
溯源树
样例
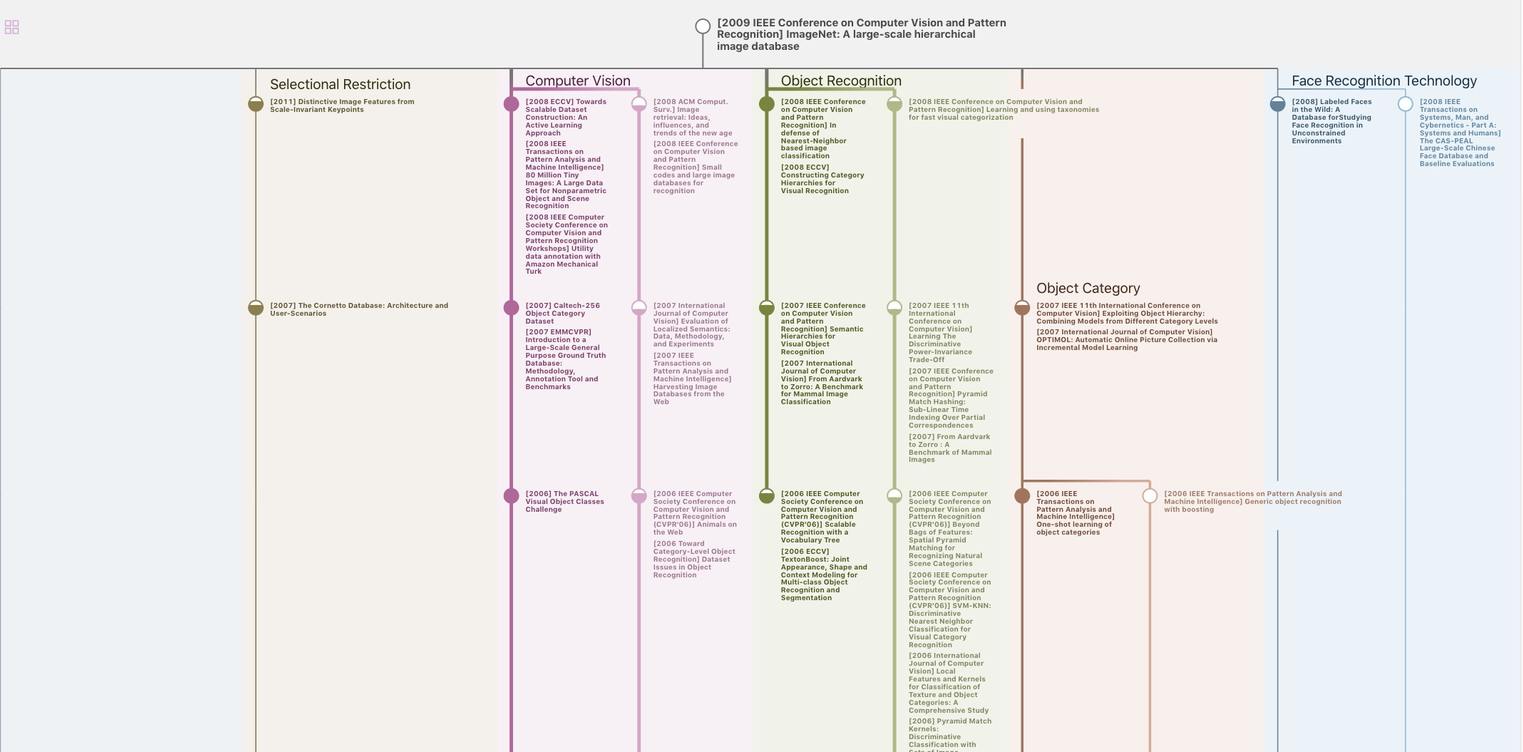
生成溯源树,研究论文发展脉络
Chat Paper
正在生成论文摘要