Smoothed Analysis for Efficient Semi-definite Programming
conference on learning theory(2018)
摘要
Semi-definite programs are important in learning and combinatorial optimization with numerous applications. In pursuit of low rank solutions and low complexity algorithms, we consider the Burer-Monteiro factorization approach for solving SDPs. Existing results for such factorization based technique either apply to a very restricted class of problems citep{boumal2016non} or do not provide polynomial time computational complexity citep{journee2010low}. In this work, we show that Burer-Monteiro factorization can be applied to emph{any} canonical form SDP with a feasible solution, and show that we can find approximate global optima for any rank-constrained SDP as long as the number of constraints scale sub-quadratically with the desired rank of the optimal solution. Our result leverages a simple penalty function based formulation of SDP along with smooth analysis of perturbed SDP to avoid worst-case cost matrices. Applications to two important problems, MAXCUT and matrix-completion, demonstrate generality of our technique and how we can ensure polynomial time algorithm despite producing optimal low-rank solutions.
更多查看译文
AI 理解论文
溯源树
样例
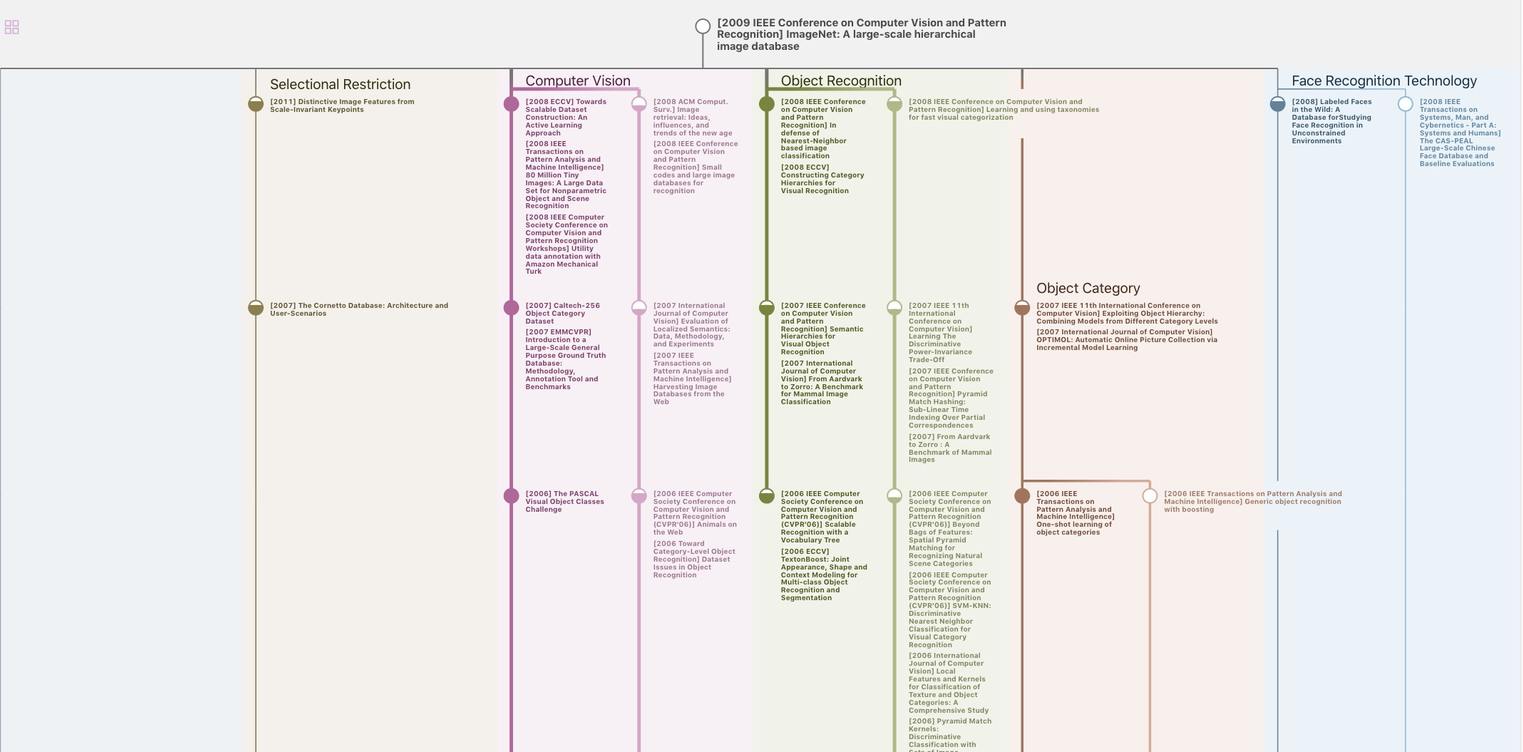
生成溯源树,研究论文发展脉络
Chat Paper
正在生成论文摘要