Mining therapeutic insights from large scale drug screenings with transfer learning
bioRxiv(2018)
摘要
Despite the abundance of large-scale molecular and drug-response data, our ability to extract the underlying mechanisms of diseases and treatment efficacy has been in general limited. Machine learning algorithms applied to those data sets most often are used to provide predictions without interpretation, or reveal single drug-gene association and fail to derive robust insights. We propose to use Macau, a bayesian multitask multi-relational algorithm to generalize from individual drugs and genes and explore the association between the drug targets and signaling pathway activations. A typical insight would be: Activation of pathway Y will confer sensitivity to any drug targeting protein X. We applied our methodology to the Genomics of Drug Sensitivity in Cancer (GDSC) screening, using signaling pathwaysu0027 activities as cell line input and nominal targets as drug input. The interactions between the drug target and the pathway activity can guide a tissue specific treatment strategy by for example suggesting how to modulate a certain protein to maximize the drug response for a given tissue. We confirmed in literature drug combination strategies derived from our result for brain, skin and stomach tissues. Such an analysis of interactions across tissues might help drug repurposing and patient stratification strategies.
更多查看译文
AI 理解论文
溯源树
样例
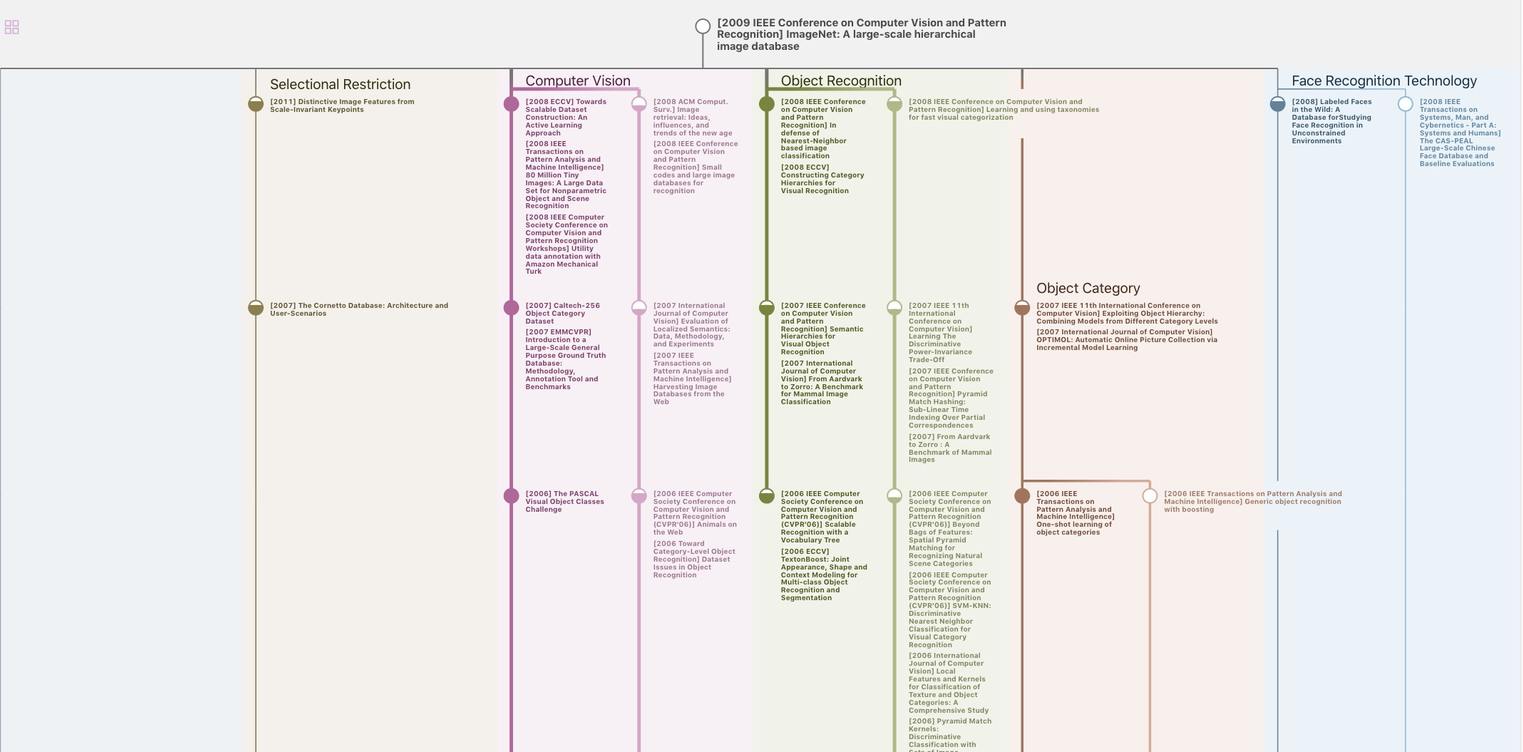
生成溯源树,研究论文发展脉络
Chat Paper
正在生成论文摘要