Application and validation of a line-source dispersion model to estimate small scale traffic-related particulate matter concentrations across the conterminous US
Air Quality, Atmosphere & Health(2018)
摘要
Numerous studies document increased health risks from exposure to traffic and traffic-related particulate matter (PM). However, many studies use simple exposure metrics to represent traffic-related PM, and/or are limited to small geographic areas over relatively short (e.g., 1 year) time periods. We developed a modeling approach for the conterminous US from 1999 to 2011 that applies a line-source Gaussian plume dispersion model using several spatially and/or temporally varying inputs (including daily meteorology) to produce high spatial resolution estimates of primary near-road traffic-related PM levels. We compared two methods of spatially averaging traffic counts: spatial smoothing generalized additive models and kernel density. Also, we evaluated and validated the output from the line-source dispersion modeling approach in a spatio-temporal model of 24-h average PM < 2.5 μm (PM 2.5 ) elemental carbon (EC) levels. We found that spatial smoothing of traffic count point data performed better than a kernel density approach. Predictive accuracy of the spatio-temporal model of PM 2.5 EC levels was moderate for 24-h averages (cross-validation (CV) R 2 = 0.532) and higher for longer averaging times (CV R 2 = 0.707 and 0.795 for monthly and annual averages, respectively). PM 2.5 EC levels increased monotonically with line-source dispersion model output. Predictive accuracy was higher when the spatio-temporal model of PM 2.5 EC included line-source dispersion model output compared to distance to road terms. Our approach provides estimates of primary traffic-related PM levels with high spatial resolution across the conterminous US from 1999 to 2011. Spatio-temporal model predictions describe 24-h average PM 2.5 EC levels at unmeasured locations well, especially over longer averaging times.
更多查看译文
关键词
Air pollution,Spatial smoothing,Highway proximity,Traffic counts,Dispersion models
AI 理解论文
溯源树
样例
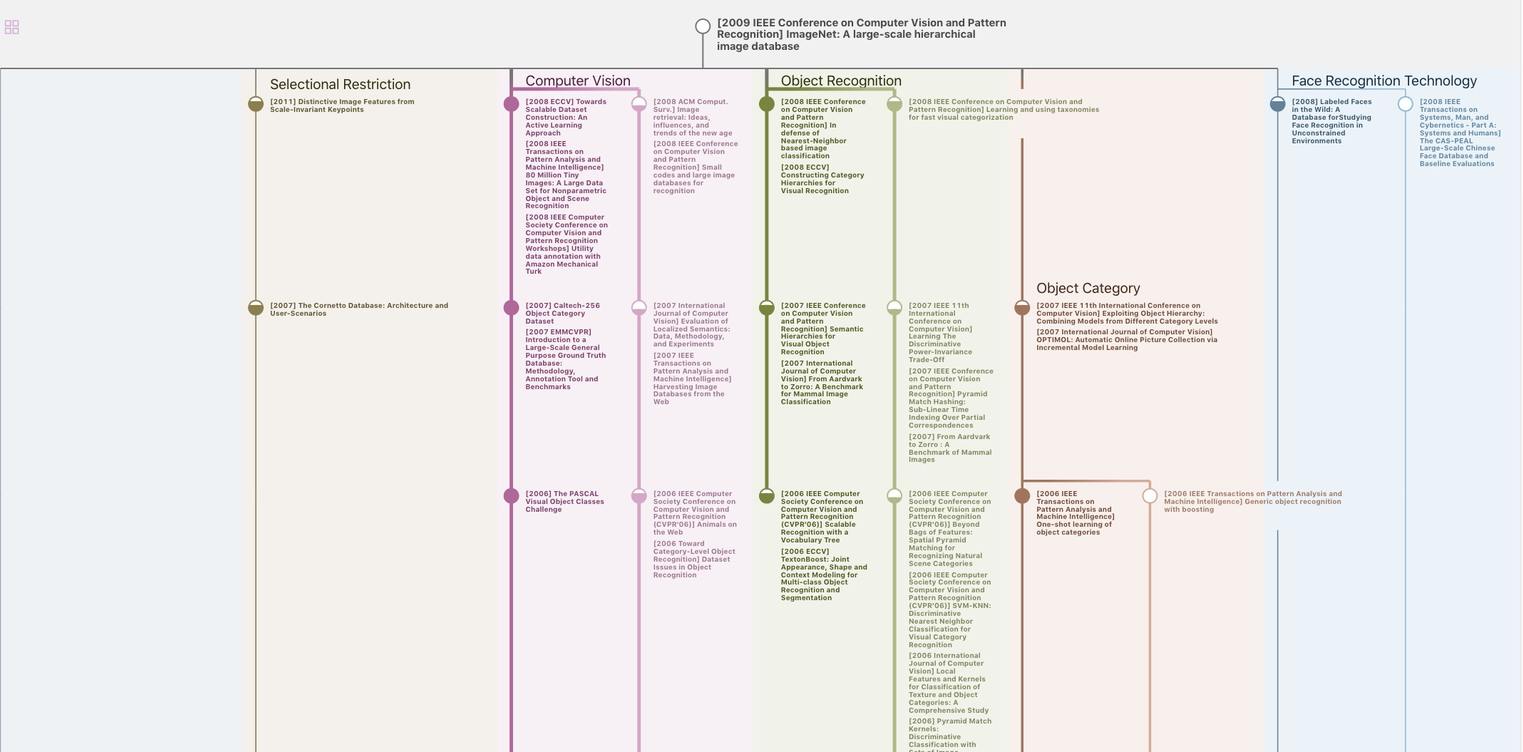
生成溯源树,研究论文发展脉络
Chat Paper
正在生成论文摘要