Soft maximin estimation for heterogeneous data
arXiv: Methodology(2022)
摘要
Extracting a common robust signal from data divided into heterogeneous groups is challenging when each group-in addition to the signal-contains large, unique variation components. Previously, maximin estimation was proposed as a robust method in the presence of heterogeneous noise. We propose soft maximin estimation as a computationally attractive alternative aimed at striking a balance between pooled estimation and (hard) maximin estimation. The soft maximin method provides a range of estimators, controlled by a parameter zeta>0$$ \zeta >0 $$, that interpolates pooled least squares estimation and maximin estimation. By establishing relevant theoretical properties we argue that the soft maximin method is statistically sensible and computationally attractive. We demonstrate, on real and simulated data, that soft maximin estimation can offer improvements over both pooled OLS and hard maximin in terms of predictive performance and computational complexity. A time and memory efficient implementation is provided in the R package SMME available on CRAN.
更多查看译文
关键词
convex optimization,heterogeneous data,robust estimation,sparse estimation
AI 理解论文
溯源树
样例
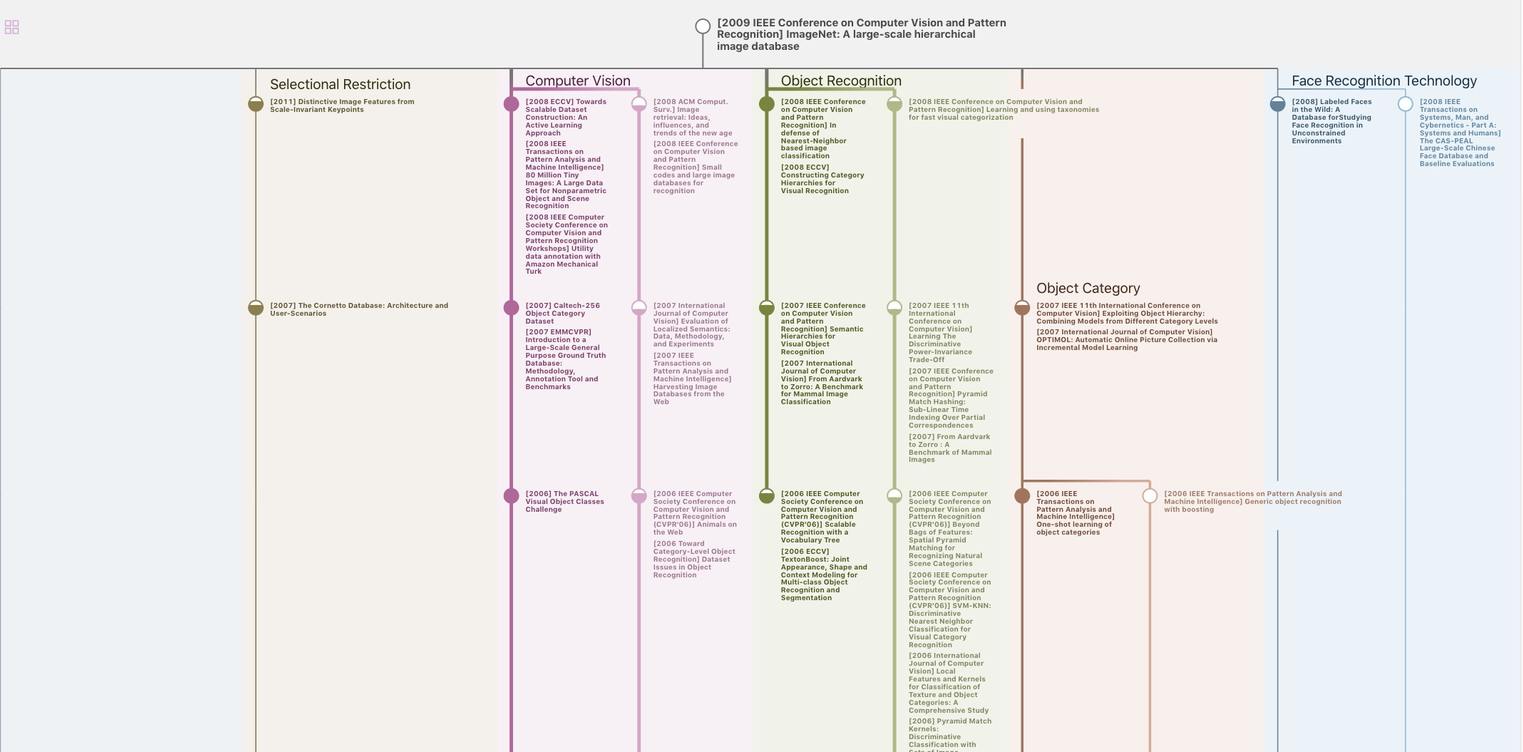
生成溯源树,研究论文发展脉络
Chat Paper
正在生成论文摘要