Forest fire forecasting using ensemble learning approaches
Neural Computing and Applications(2018)
摘要
Frequent and intense forest fires have posed severe challenges to forest management in many countries worldwide. Since human experts may overlook important signals, the development of reliable prediction models with various types of data generated by automatic tools is crucial for establishing rigorous and effective forest firefighting plans. This study applied recently emerged ensemble learning methods to predict the burned area of forest fires and the occurrence of large-scale forest fires using the forest fire dataset from the University of California, Irvine machine learning repository collected from the northeastern region of Portugal. The results showed that the tuned random forest approach performed better than other regression models did with regard to the prediction accuracy of the burned area. In addition, extreme gradient boosting outperformed other classification models in terms of its predictive accuracy of large-scale fire occurrences. The findings showed that ensemble learning methods not only have great potential for broader application in forest fire automatic precaution and prevention systems but also provide important techniques for forest firefighting decision making in terms of fire resource allocation and strategies, which can ultimately improve the efficiency of forest fire management worldwide.
更多查看译文
关键词
Ensemble learning, Random forests, Extreme gradient boosting, Large-scale forest fires
AI 理解论文
溯源树
样例
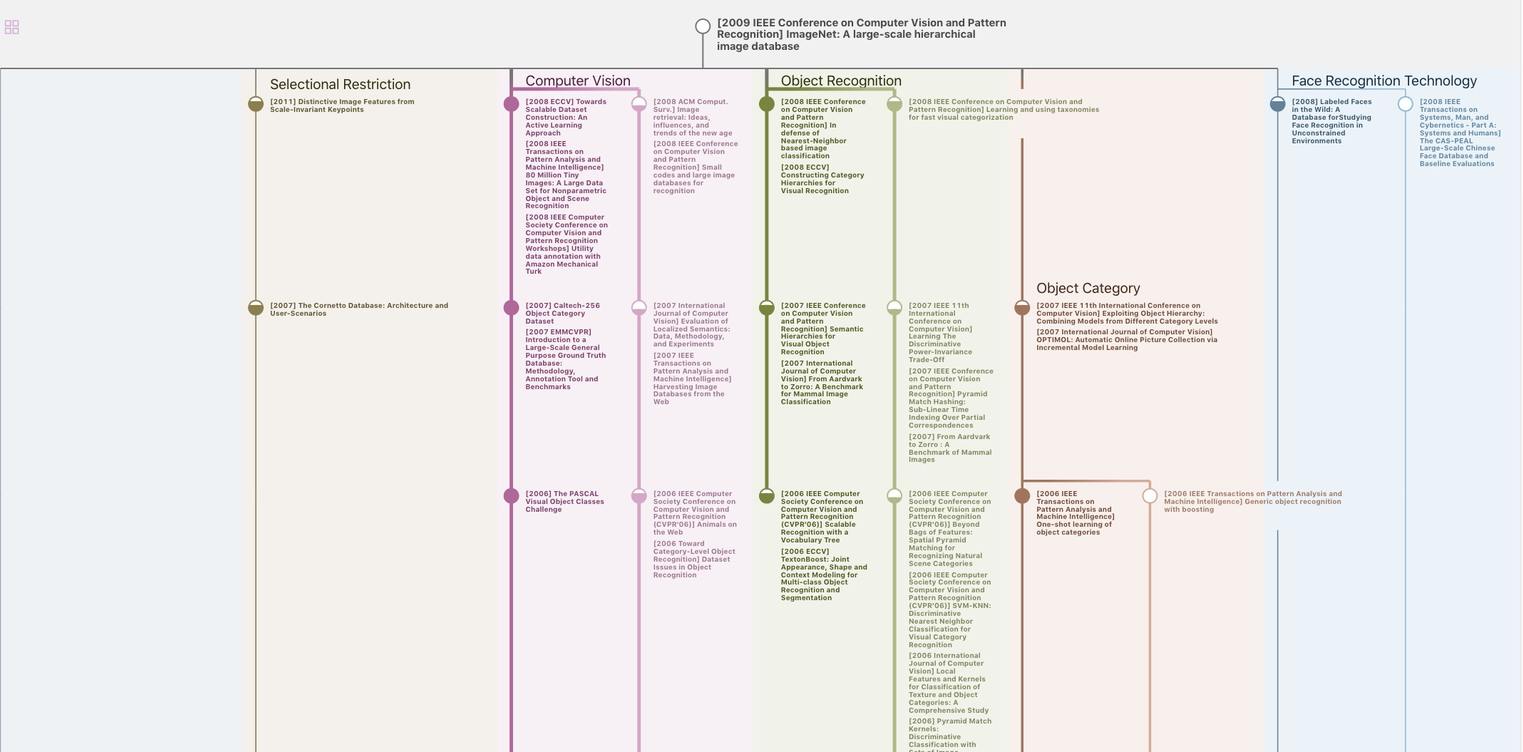
生成溯源树,研究论文发展脉络
Chat Paper
正在生成论文摘要