Deep Learning Meets Wireless Network Optimization: Identify Critical Links
IEEE Transactions on Network Science and Engineering(2020)
摘要
With the superior capability of discovering intricate structure of large data sets, deep learning has been widely applied in various areas including wireless networking. While existing deep learning applications mainly focus on data analysis, the role it can play on fundamental research issues in wireless networks is yet to be explored. With the proliferation of wireless networking infrastructure and mobile applications, wireless network optimization has seen a tremendous increase in problem size and complexity, calling for a paradigm for efficient computation. This paper presents a pioneering study on how to exploit deep learning for significant performance gain in wireless network optimization. Analysis on the flow constrained optimization problems suggests the possibility that a smaller-sized problem can be solved while sharing equally optimal solutions with the original problem, by excluding the potentially unused links from the problem formulation. To this end, we design a deep learning framework to find the latent relationship between flow information and link usage by learning from past computation experience. Numerical results demonstrate that the proposed method is capable of identifying critical links and can reduce computation cost by up to 50% without affecting optimality, thus greatly improve the efficiency of solving network optimization problems.
更多查看译文
关键词
Optimization,Machine learning,Wireless networks,Task analysis,Feature extraction,Resource management
AI 理解论文
溯源树
样例
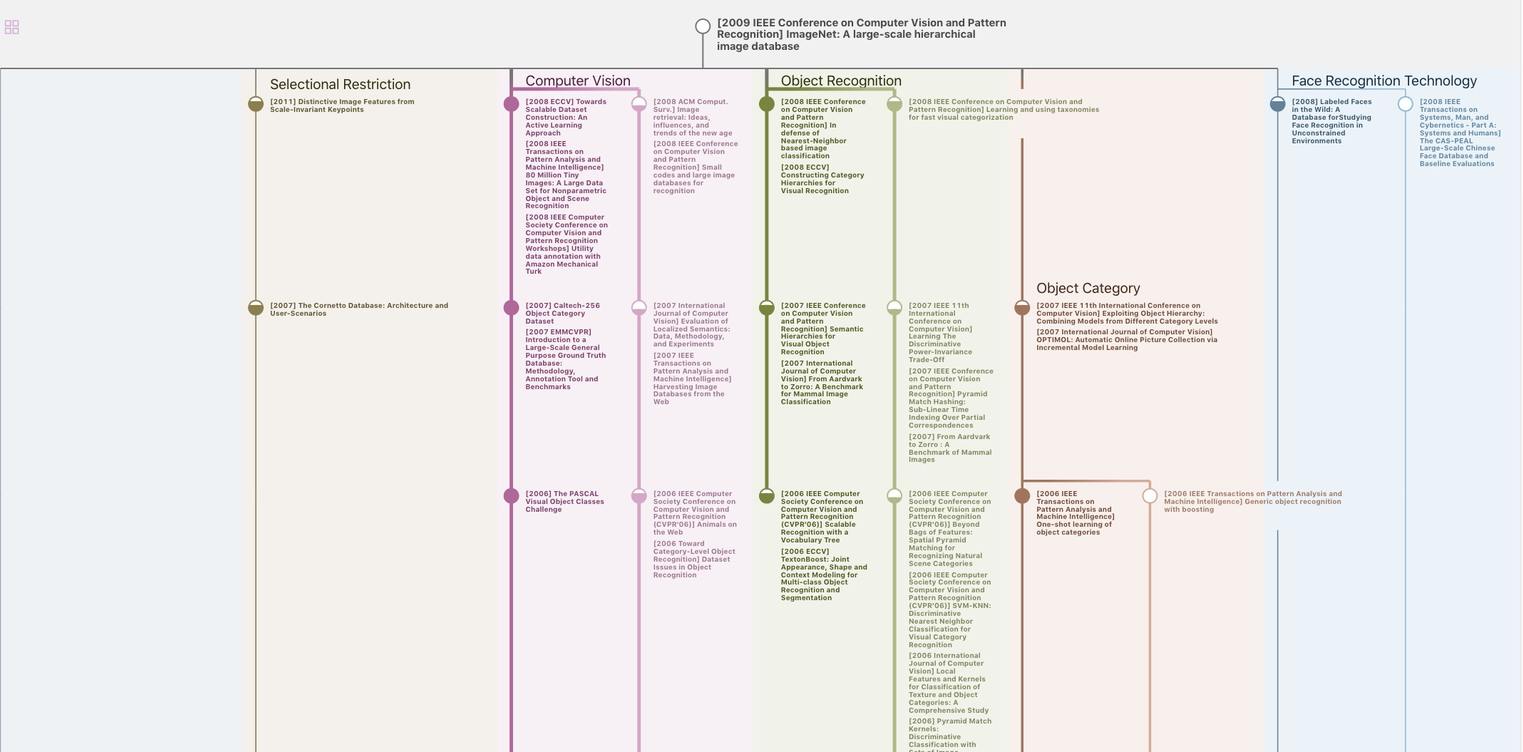
生成溯源树,研究论文发展脉络
Chat Paper
正在生成论文摘要