High performance algorithms for quantum gravity and cosmology
arXiv: General Relativity and Quantum Cosmology(2018)
摘要
Large scale numerical experiments are commonplace today in theoretical physics. The high performance algorithms described herein are the most compact, efficient methods known for representing and analyzing systems modeled well by sets or graphs. After studying how these implementations maximize instruction throughput and optimize memory access patterns, we apply them to causal set quantum gravity, in which spacetime is represented by a partially ordered set. We build upon the low-level set and graph algorithms to optimize the calculation of the causal set action, and then discuss how to measure boundaries of a discrete spacetime. We then examine the broader applicability of these algorithms to greedy information routing in random geometric graphs embedded in Lorentzian manifolds, which requires us to find new closed-form solutions to the geodesic differential equations in Friedmann-Lemaitre-Robertson-Walker spacetimes. Finally, we consider the vacuum selection problem in string theory, where we show a network-centered approach yields a dynamical mechanism for vacuum selection in the context of multiverse cosmology. These algorithms have broad applicability to many physical systems, and they improve existing methods by reducing simulation runtimes by orders of magnitude.
更多查看译文
AI 理解论文
溯源树
样例
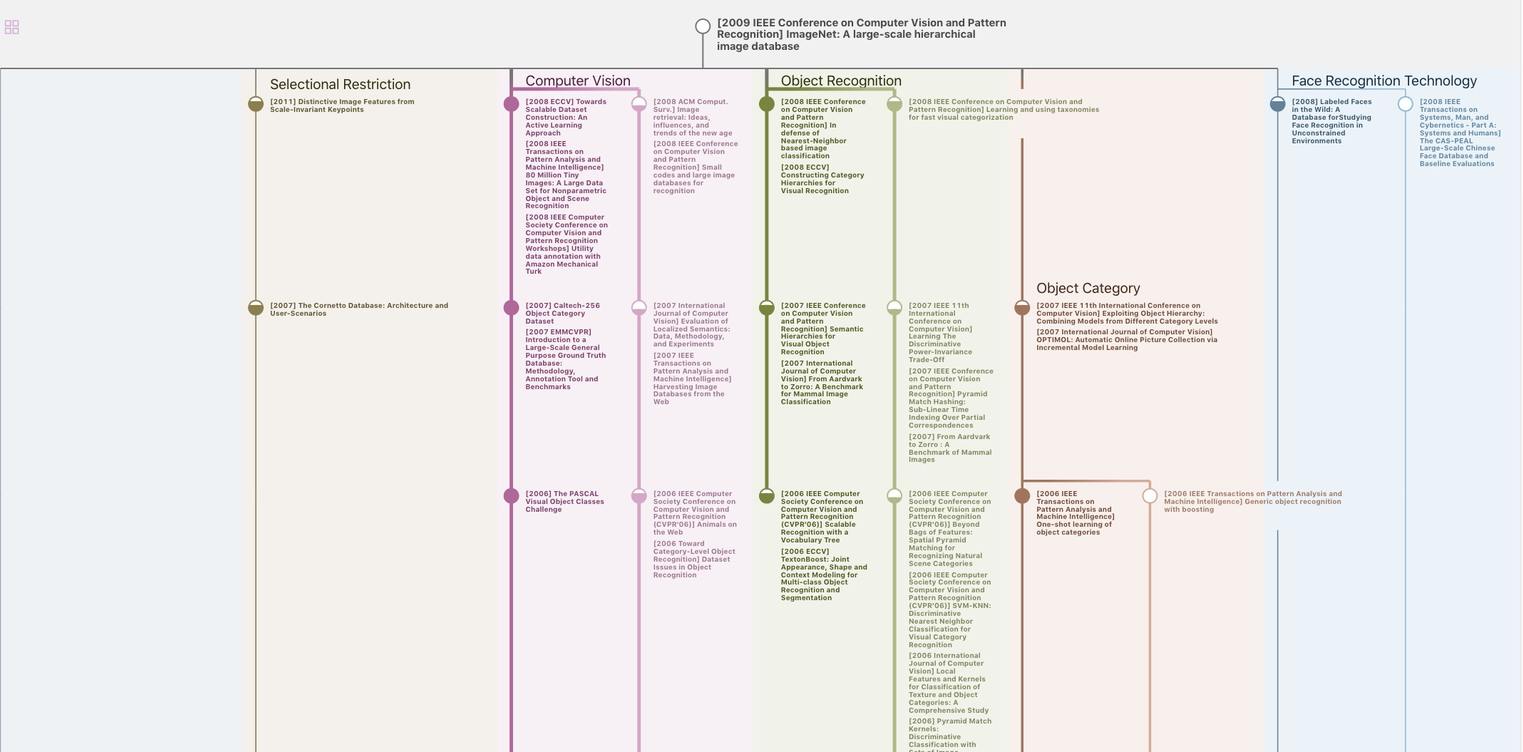
生成溯源树,研究论文发展脉络
Chat Paper
正在生成论文摘要