Extreme Dimension Reduction for Handling Covariate Shift
arXiv: Learning(2017)
摘要
In the covariate shift learning scenario, the training and test covariate distributions differ, so that a predictoru0027s average loss over the training and test distributions also differ. In this work, we explore the potential of extreme dimension reduction, i.e. to very low dimensions, in improving the performance of importance weighting methods for handling covariate shift, which fail in high dimensions due to potentially high train/test covariate divergence and the inability to accurately estimate the requisite density ratios. We first formulate and solve a problem optimizing over linear subspaces a combination of their predictive utility and train/test divergence within. Applying it to simulated and real data, we show extreme dimension reduction helps sometimes but not always, due to a bias introduced by dimension reduction.
更多查看译文
AI 理解论文
溯源树
样例
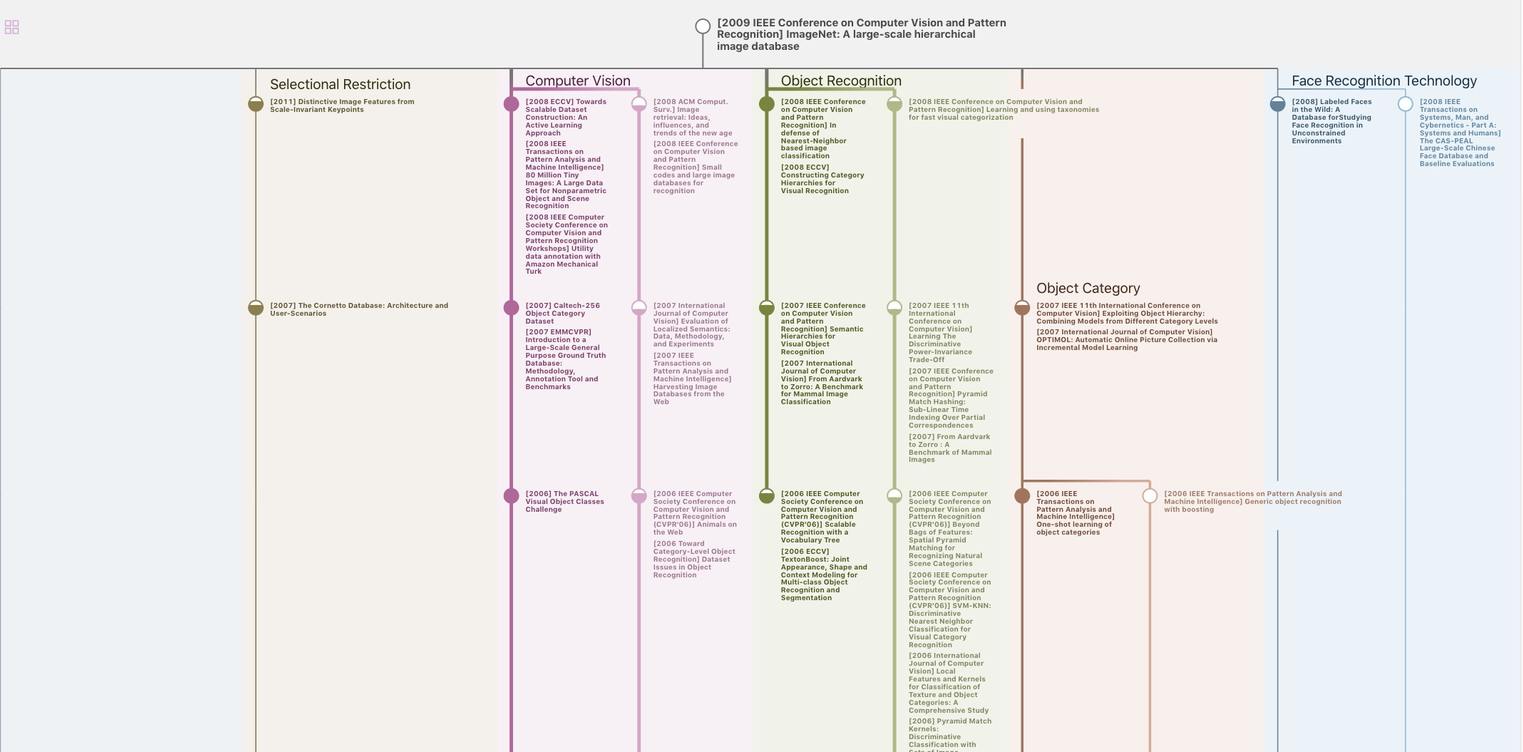
生成溯源树,研究论文发展脉络
Chat Paper
正在生成论文摘要