An Adaptive Method For N-Dimensional Tensor Factorization On Sparse Data Processing
PROCEEDINGS OF 2017 3RD IEEE INTERNATIONAL CONFERENCE ON COMPUTER AND COMMUNICATIONS (ICCC)(2017)
摘要
Big data produced in our life implies rich information and can help us better understand the world when used correctly. However, the data collected is always too sparse to be directly applied. Tensor factorization as an extension of matrix factorization has been more and more popular on data mining. The classical methods for tensor factorization are all for dense tensors, which is no longer efficient for sparse data tensor. The most-used factorization method for sparse tensor also exists some problems, such as long convergence time, low fitting precision, complex parameter settings and so on. In this paper, we propose an adaptive stochastic gradient descent method, called RMSGD for N-dimensional tensor factorization to deal with data sparsity problem and predict the missing values in a sparse tensor precisely. We evaluate our method based on two real data sets, i.e., the GPS trajectories generated by 2000 taxis in Beijing and the 311 complaint data collected from New York City (NYC). The results demonstrate that the effectiveness and efficiency of our method are better than the baseline approaches.
更多查看译文
关键词
gradient descent, tensor factorization, big data, sparsity
AI 理解论文
溯源树
样例
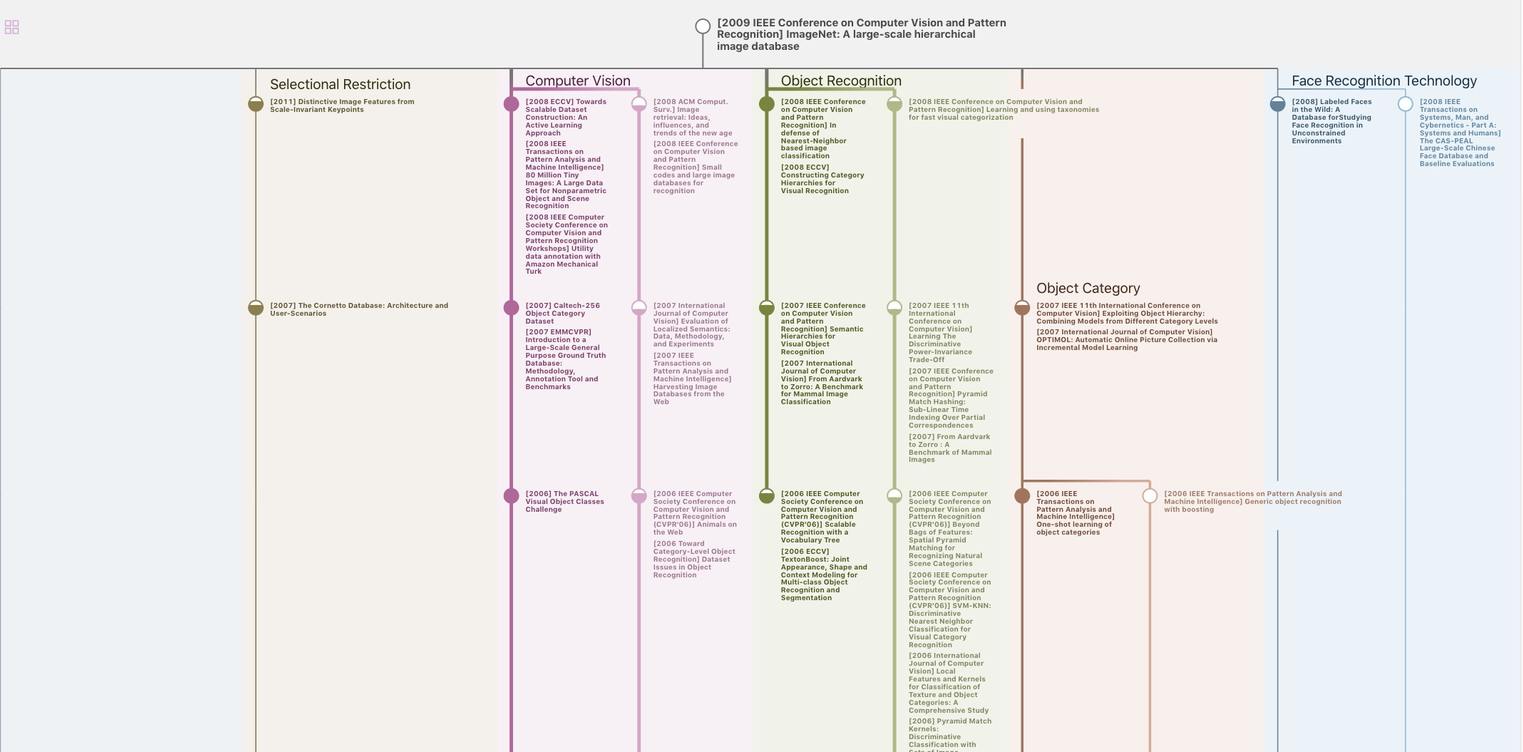
生成溯源树,研究论文发展脉络
Chat Paper
正在生成论文摘要