Tracking Dynamics of Opinion Behaviors with a Content-based Sequential Opinion Influence Model
IEEE Transactions on Affective Computing(2020)
摘要
Nowadays, social media has become a popular channel for people to exchange opinions through the user-generated text. Exploring the mechanisms about how customersu0027 opinions towards products are influenced by friends, and further predicting their future opinions have attracted great attention from corporate administrators and researchers. Various influence models have already been proposed for the opinion prediction problem. However, they largely formulate opinions as derived sentiment categories or values but ignore the role of the content information. Besides, existing models only make use of the most recently received information without taking into consideration the long-term historical communication. To keep track of user opinion behaviors and infer user opinion influence from the historical exchanged textual information, we develop a content-based sequential opinion influence framework. Based on this framework, two opinion sentiment prediction models with alternative prediction strategies are proposed. In the experiments conducted on three Twitter datasets, the proposed models outperform other popular influence models. An interesting finding based on a further analysis of user characteristic is that an individualu0027s influence is correlated to her/his style of expressions.
更多查看译文
关键词
Predictive models, Computational modeling, Twitter, Recurrent neural networks, Training, Ions, Influence model, opinion dynamics, sentiment prediction
AI 理解论文
溯源树
样例
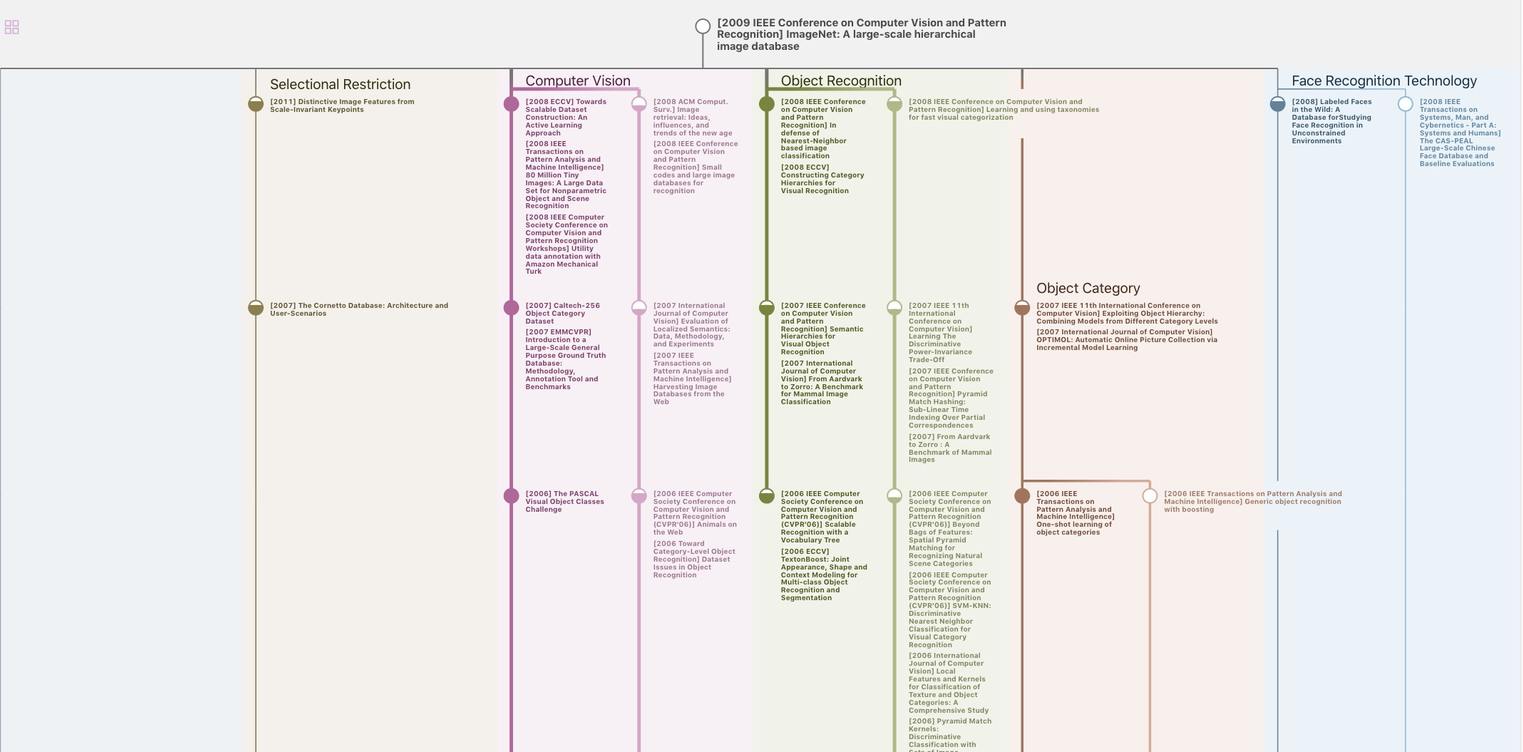
生成溯源树,研究论文发展脉络
Chat Paper
正在生成论文摘要