LOCAL WEAK CONVERGENCE FOR PAGERANK
ANNALS OF APPLIED PROBABILITY(2020)
摘要
PageRank is a well-known algorithm for measuring centrality in networks. It was originally proposed by Google for ranking pages in the World Wide Web. One of the intriguing empirical properties of PageRank is the so-called 'power-law hypothesis': in a scale-free network, the PageRank scores follow a power law with the same exponent as the (in-)degrees. To date, this hypothesis has been confirmed empirically and in several specific random graphs models. In contrast, this paper does not focus on one random graph model but investigates the existence of an asymptotic PageRank distribution, when the graph size goes to infinity, using local weak convergence. This may help to identify general network structures in which the power-law hypothesis holds. We start from the definition of local weak convergence for sequences of (random) undirected graphs, and extend this notion to directed graphs. To this end, we define an exploration process in the directed setting that keeps track of in- and out-degrees of vertices. Then we use this to prove the existence of an asymptotic PageRank distribution. As a result, the limiting distribution of PageRank can be computed directly as a function of the limiting object. We apply our results to the directed configuration model and continuous-time branching processes trees, as well as to preferential attachment models.
更多查看译文
关键词
Directed random graphs,PageRank,local weak convergence
AI 理解论文
溯源树
样例
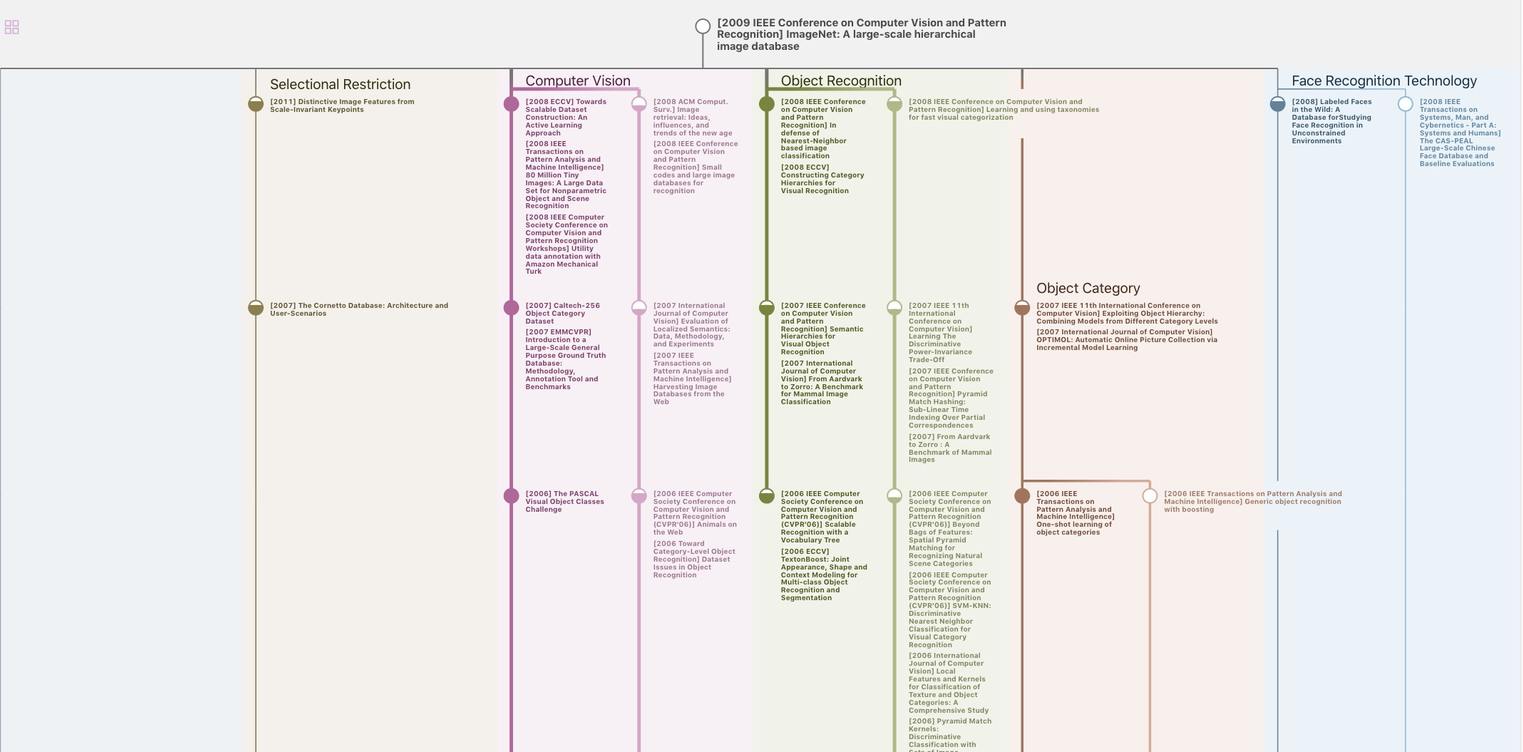
生成溯源树,研究论文发展脉络
Chat Paper
正在生成论文摘要