The Roles of Suprasegmental Features in Predicting English Oral Proficiency with an Automated System.
LANGUAGE ASSESSMENT QUARTERLY(2018)
摘要
Suprasegmental features have received growing attention in the field of oral assessment. In this article we describe a set of computer algorithms that automatically scores the oral proficiency of non-native speakers using unconstrained English speech. The algorithms employ machine learning and 11 suprasegmental measures divided into four groups (prominence, filled pause, speech rate, and intonation) to calculate the proficiency scores. In test responses from 120 non-native speakers of English monologues from the Cambridge English Language Assessment (CELA), the Pearson's correlation between the computer's calculated proficiency levels and the official CELA proficiency levels was 0.718. The current findings provide empirical evidence that prominence and intonation are salient features in the computer model's prediction of proficiency.
更多查看译文
AI 理解论文
溯源树
样例
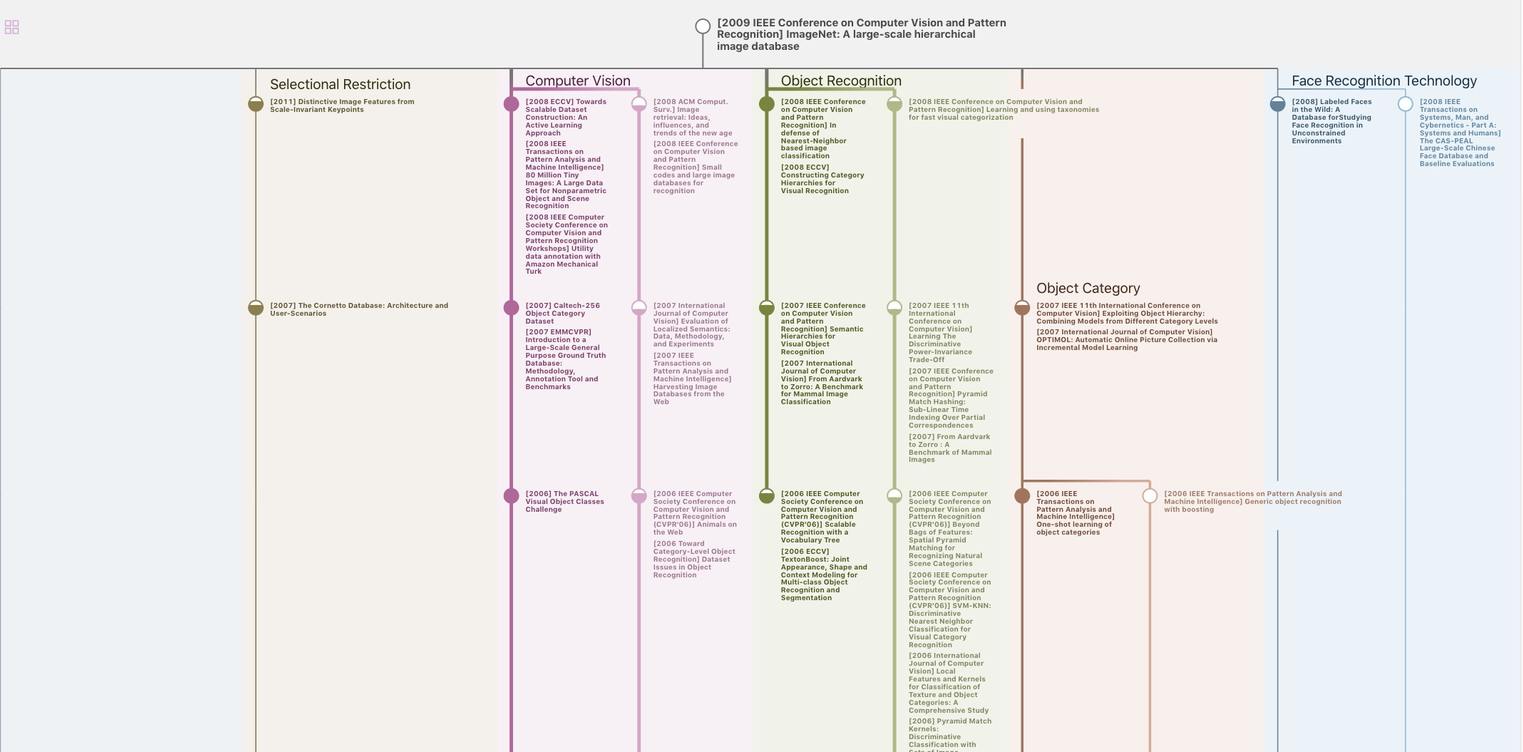
生成溯源树,研究论文发展脉络
Chat Paper
正在生成论文摘要