Region of interest extraction via common salient feature analysis and feedback reinforcement strategy for remote sensing images
GISCIENCE & REMOTE SENSING(2018)
摘要
Saliency detection is an effective approach to extract regions of interest (ROIs) for remote sensing images. However, existing saliency detection models mainly focus on ROI extraction from a single image and usually are not able to produce satisfactory results because of complex background interference in remote sensing images. The employment of mutual information in a set of remote sensing images can provide an effective solution to this issue. In this paper, we propose a novel saliency detection model for multiple remote sensing images to simultaneously extract ROIs and identify images without ROIs. First, common salient feature analysis based on synthesized feature clustering and global contrast is implemented to exploit global correspondence in the synthesis feature domain, thereby highlighting preliminary ROIs against background interference and assigning lower saliency values to images without ROIs. Then, we design an exclusion criterion based on saliency value judgment to remove images without ROIs, and the remaining saliency maps are refined by an enhancement strategy. Finally, the enhanced maps serve as a feedback to yield a homogenous synthesized feature space in which integral ROIs with subtle borders are extracted by the reused cluster-based saliency calculation. Experiments reveal that our model outperforms seven state-of-the-art models by achieving the best ROC curve (AUC=0.945) and maximal F-measure at 0.729.
更多查看译文
关键词
Remote sensing,saliency detection,region of interest extraction,common salient feature analysis,feedback reinforcement
AI 理解论文
溯源树
样例
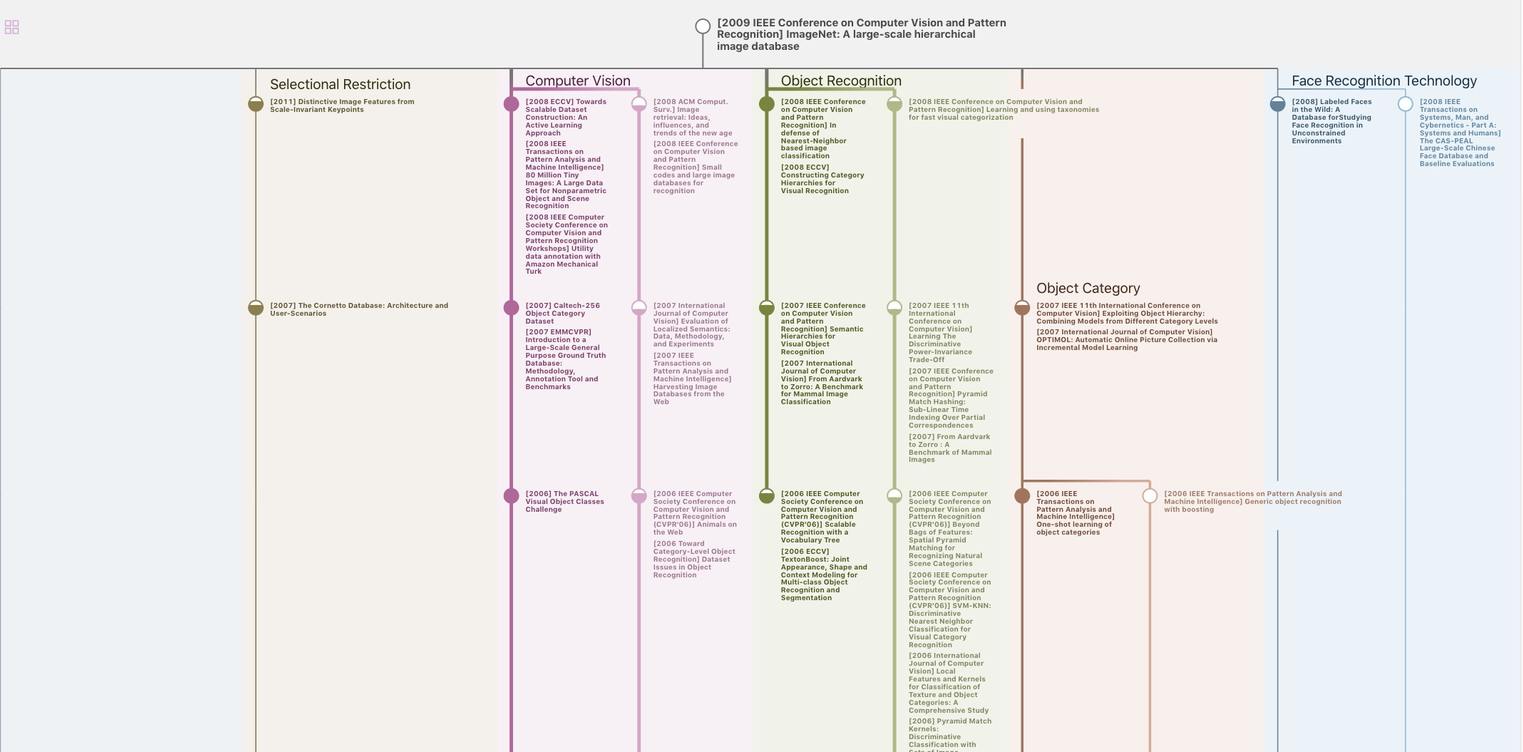
生成溯源树,研究论文发展脉络
Chat Paper
正在生成论文摘要