TESTABILITY OF HIGH-DIMENSIONAL LINEAR MODELS WITH NONSPARSE STRUCTURES
ANNALS OF STATISTICS(2022)
摘要
Understanding statistical inference under possibly nonsparse high-dimensional models has gained much interest recently. For a given component of the regression coefficient, we show that the difficulty of the problem depends on the sparsity of the corresponding row of the precision matrix of the covariates, not the sparsity of the regression coefficients. We develop new concepts of uniform and essentially uniform nontestability that allow the study of limitations of tests across a broad set of alternatives. Uniform nontestability identifies a collection of alternatives such that the power of any test, against any alternative in the group, is asymptotically at most equal to the nominal size. Implications of the new constructions include new minimax testability results that, in sharp contrast to the current results, do not depend on the sparsity of the regression parameters. We identify new tradeoffs between testability and feature correlation. In particular, we show that, in models with weak feature correlations, minimax lower bound can be attained by a test whose power has the root n rate, regardless of the size of the model sparsity.
更多查看译文
关键词
Minimax theory, l(2)-constraint, confidence intervals, uniform nontestability
AI 理解论文
溯源树
样例
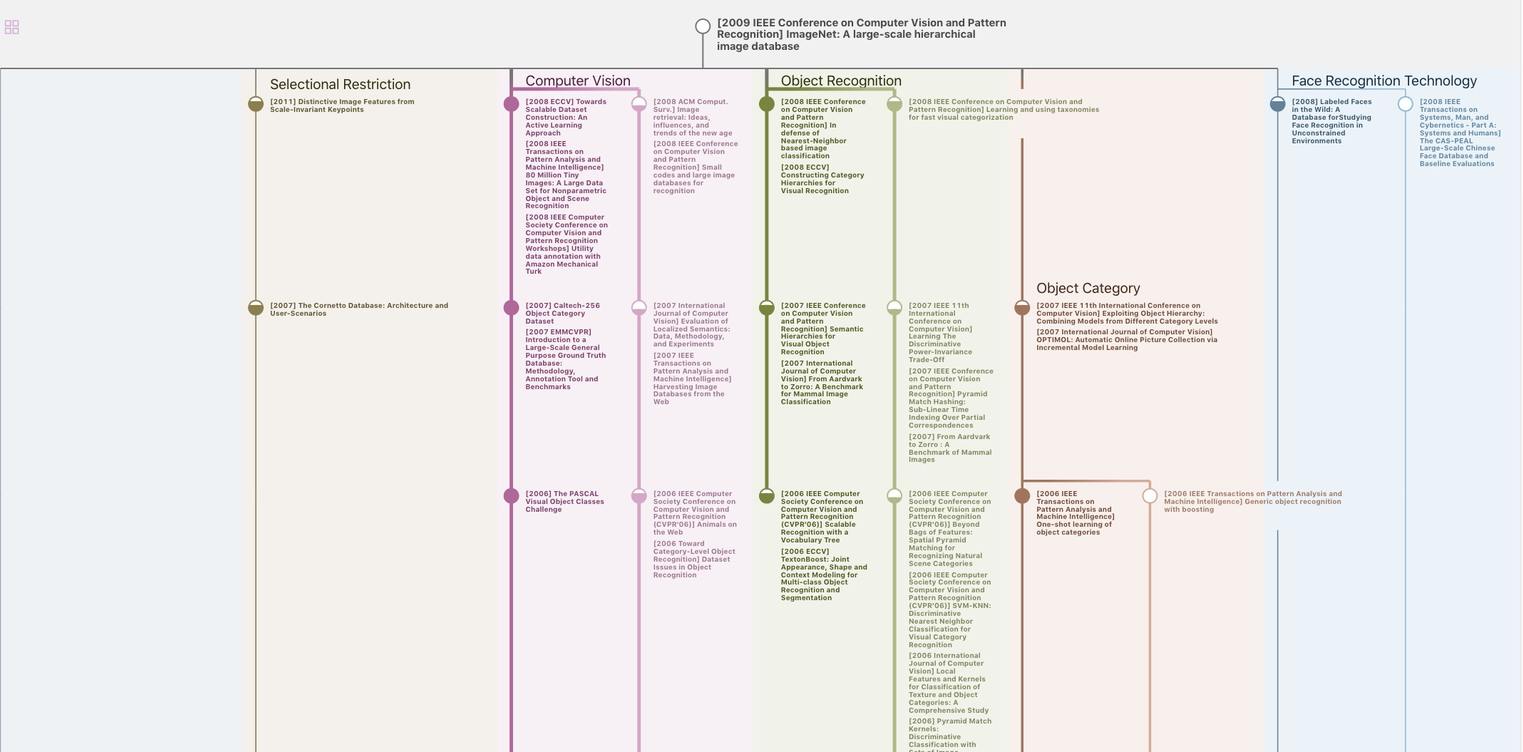
生成溯源树,研究论文发展脉络
Chat Paper
正在生成论文摘要