Air Markov Chain Monte Carlo
arXiv: Computation(2018)
摘要
We introduce a class of Adapted Increasingly Rarely Markov Chain Monte Carlo (AirMCMC) algorithms where the underlying Markov kernel is allowed to be changed based on the whole available chain output but only at specific time points separated by an increasing number of iterations. The main motivation is the ease of analysis of such algorithms. Under the assumption of either simultaneous or (weaker) local simultaneous geometric drift condition, or simultaneous polynomial drift we prove the $L_2-$convergence, Weak and Strong Laws of Large Numbers (WLLN, SLLN), Central Limit Theorem (CLT), and discuss how our approach extends the existing results. We argue that many of the known Adaptive MCMC algorithms may be transformed into the corresponding Air versions, and provide an empirical evidence that performance of the Air version stays virtually the same.
更多查看译文
AI 理解论文
溯源树
样例
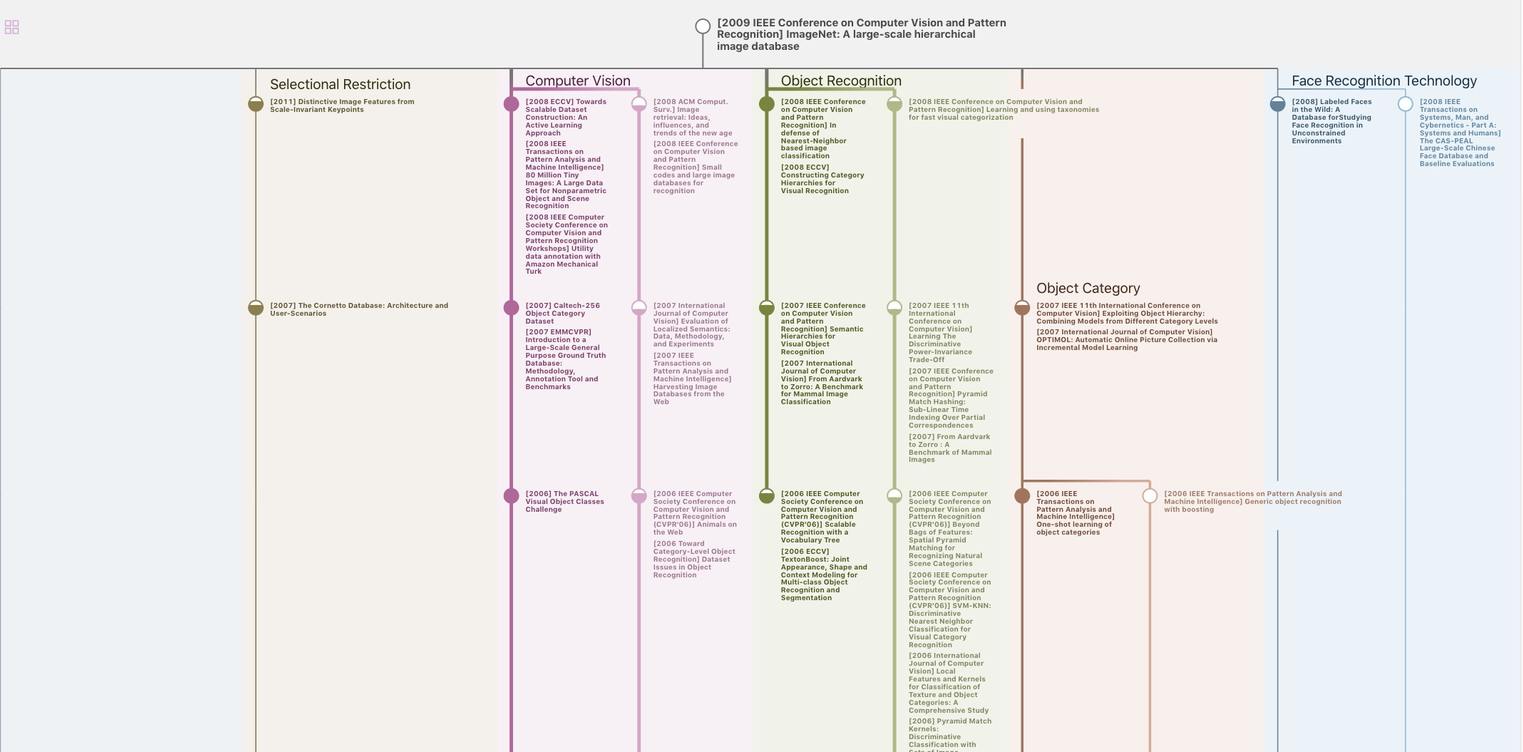
生成溯源树,研究论文发展脉络
Chat Paper
正在生成论文摘要