Accelerating data gravitation-based classification using GPU
The Journal of Supercomputing(2018)
摘要
Data gravitation-based classification model, a new physic law inspired classification model, has been demonstrated to be an effective classification model for both standard and imbalanced tasks. However, due to its large scale of gravitational computation during the feature weighting process, DGC suffers from high computational complexity, especially for large data sets. In this paper, we address the problem of speeding up gravitational computation using graphics processing unit (GPU). We design a GPU parallel algorithm namely GPU–DGC to accelerate the feature weighting process of the DGC model. Our GPU–DGC model distributes the gravitational computing process to parallel GPU threads, in order to compute gravitation simultaneously. We use 25 open classification data sets to evaluate the parallel performance of our algorithm. The relationship between the speedup ratio and the number of GPU threads is discovered and discussed based on the empirical studies. The experimental results show the effectiveness of GPU–DGC, with the maximum speedup ratio of 87 to the serial DGC. Its sensitivity to the number of GPU threads is also discovered in the empirical studies.
更多查看译文
关键词
Machine learning, Parallel algorithm, Data gravitation classification, Graphics processing unit
AI 理解论文
溯源树
样例
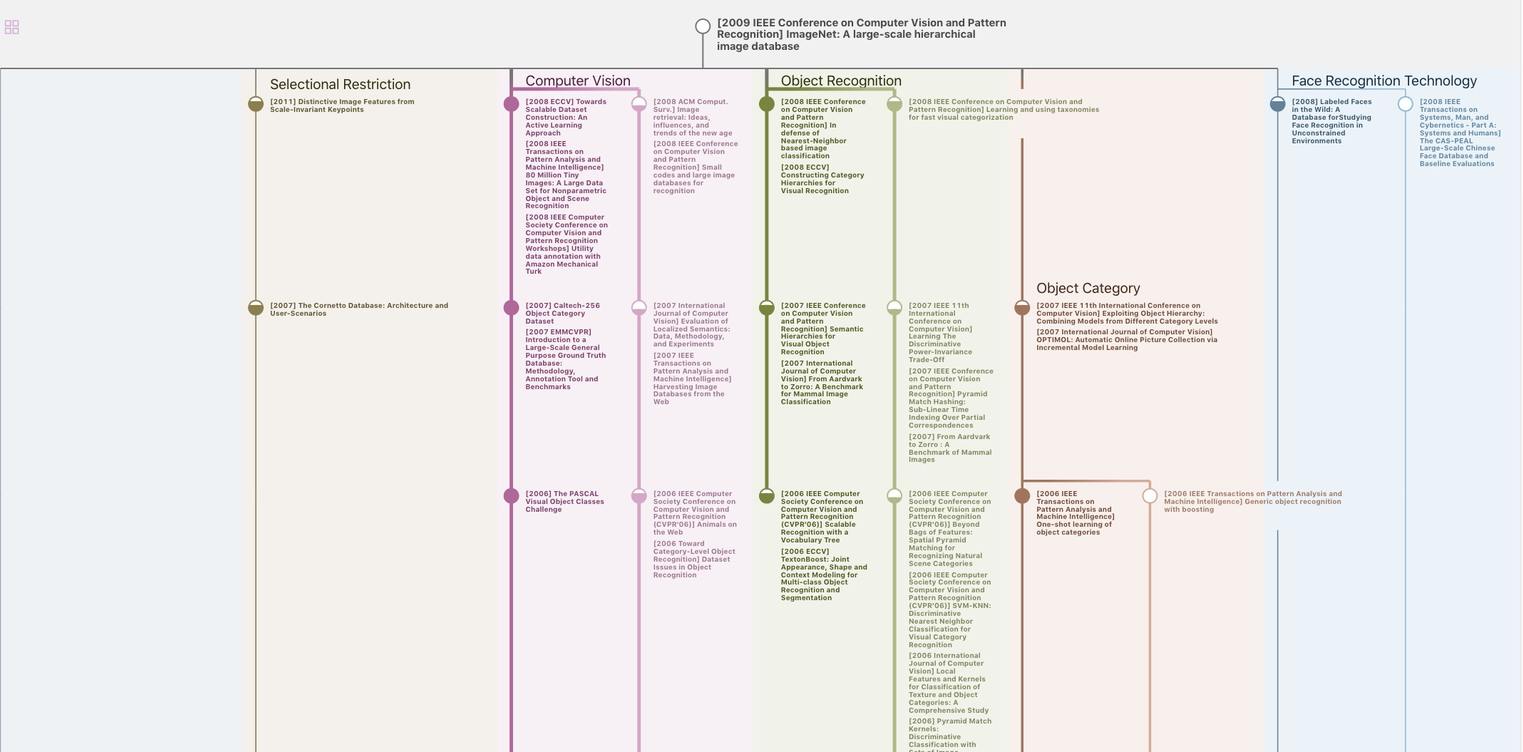
生成溯源树,研究论文发展脉络
Chat Paper
正在生成论文摘要