Progressive Learning Strategies For Multi-Class Classification
2017 INTERNATIONAL AUTOMATIC CONTROL CONFERENCE (CACS)(2017)
摘要
The progressive learning paradigm is inspired from human learning theory which enables a classifier to learn multiple new classes on the fly by adapting, growing and restructuring the network automatically to facilitate learning of the newly introduced classes while maintaining the knowledge of previously learnt classes. In this paper, we propose different progressive learning strategies for multi-class classification and analyze their performance metrics. The ability to learn completely new classes anytime makes the progressive learning classifiers highly suitable for several real-world applications in which the number of classes is often unknown apriori and new classes evolve over time. Several standard datasets are used to evaluate the performance of the different progressive learning strategies and are also compared with other existing online learning approaches.
更多查看译文
关键词
classification, machine learning, progressive learning, multi-class, online learning
AI 理解论文
溯源树
样例
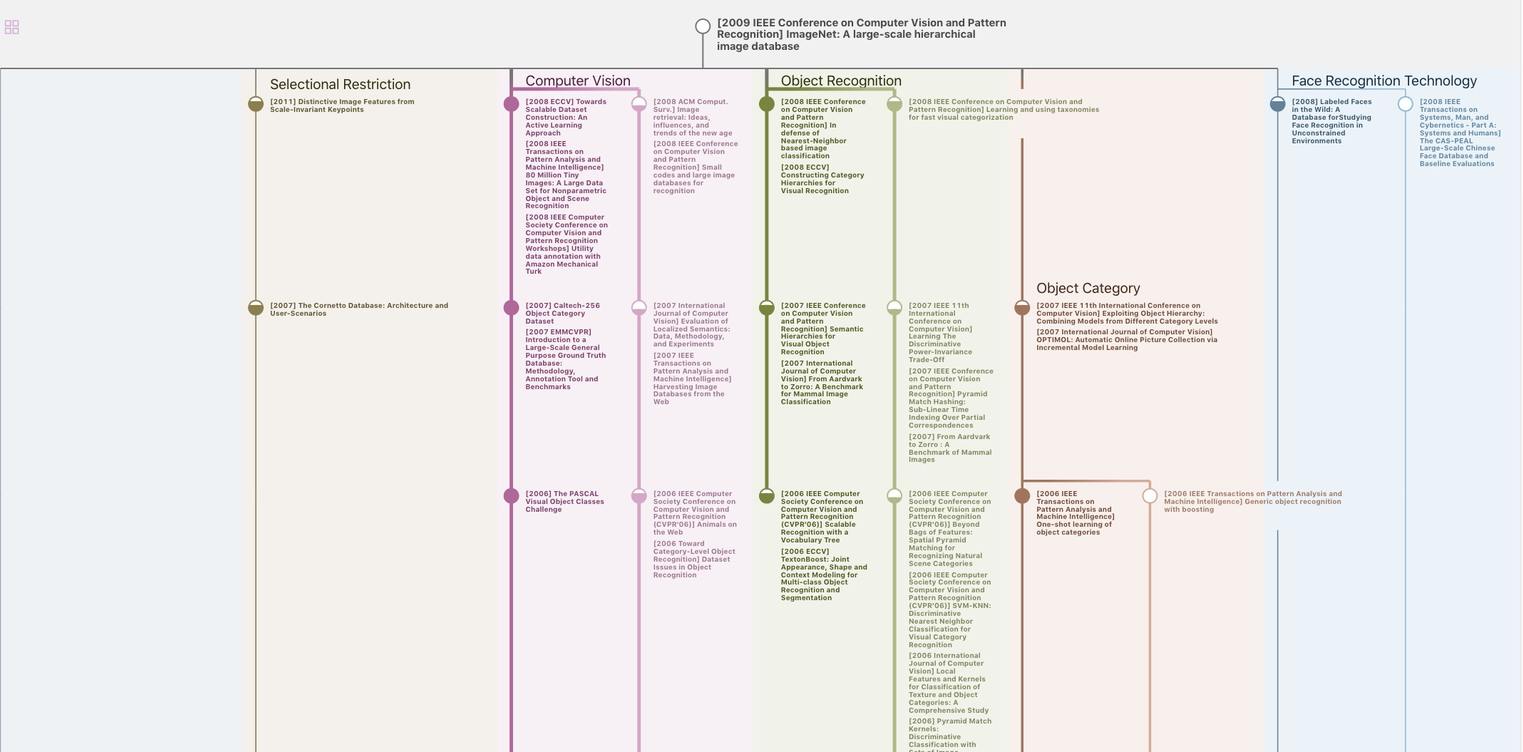
生成溯源树,研究论文发展脉络
Chat Paper
正在生成论文摘要