Application of evolutionary neural networks and support vector machines to model NO x emissions from gas turbines
JOURNAL OF ENVIRONMENTAL CHEMICAL ENGINEERING(2018)
摘要
Evolutionary artificial neural networks and support vector machines were investigated for the modeling of NO,, emissions from gas turbines. For ANN's, a genetic algorithm (GA) with indirect binary encoding was employed to obtain optimal multi-layer perceptron (MLP)-type architectures. The contribution of this research includes the use of an improved GA objective function that takes advantage of the number of effective parameters provided by Bayesian regularization. For SVM's, a GA is also used with a search space covering all hyperparameters, including the choice of the kernel itself. The objective function took advantage of the number of support vectors to yield models that are more capable of generalization and are less computationally expensive. To test the performance of these models, three datasets describing NO emissions from three different turbines were used. In previous works, these emissions were modeled using a shallow network architecture obtained by trial and error. When the performance of the models was compared, the optimized ANN's reduced the mean squared error (MSE) over testing sets by up to 90%, and optimized SVM's led to a reduction of up to 70%; with the latter's greatest advantage being computational efficiency and consistency with the different GA runs. The GA converged in a period in the order of minutes, which is more efficient than trial-and-error procedures. This work shows that more attention should be given to the influence of machine learning model architectures on the accuracy of PEMS models, as the computational cost of the GA is well justified by the resulting high accuracy.
更多查看译文
关键词
Predictive emissions monitoring,Artificial neural network,Support vector machines,Genetic algorithm,Gas turbine
AI 理解论文
溯源树
样例
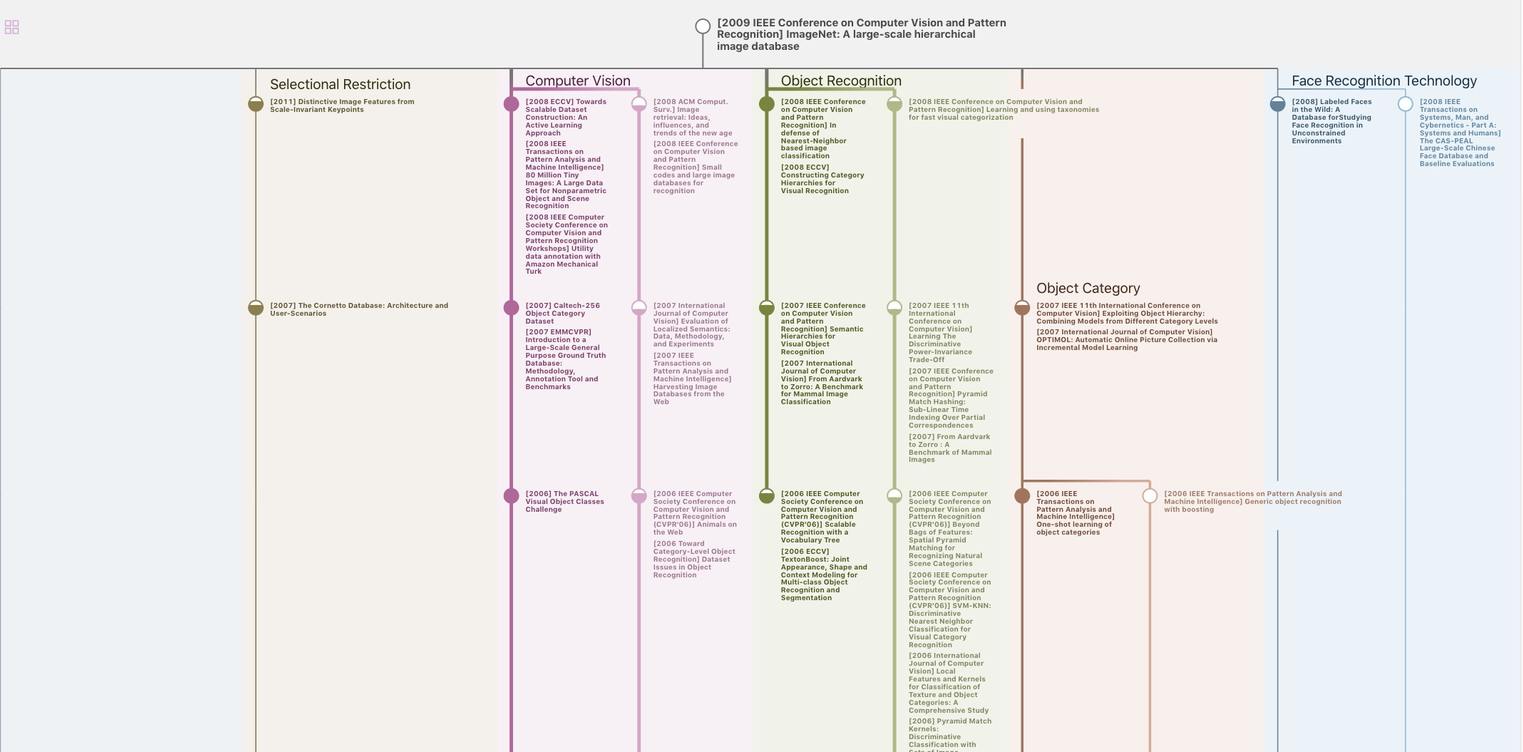
生成溯源树,研究论文发展脉络
Chat Paper
正在生成论文摘要