Associativity Analysis of SO 2 and NO 2 for Alberta Monitoring Data Using KZ Filtering and Hierarchical Clustering
Atmospheric Chemistry and Physics(2018)
摘要
Abstract. Associativity analysis is a powerful tool to deal with large-scale datasets by clustering the data on the basis of (dis)similarity, and can be used to assess the efficacy and design of air-quality monitoring networks. We describe here our use of Kolmogorov-Zurbenko filtering and hierarchical clustering of NO 2 and SO 2 passive and continuous monitoring data, to analyse and optimize air quality networks for these species in the province of Alberta, Canada. The methodology applied in this study assesses dissimilarity between monitoring station time series based on two metrics: 1-R, R being the Pearson correlation coefficient, and the Euclidean distance. We have combined the analytic power of hierarchical clustering with the spatial information provided by deterministic air quality model results, using the gridded time series of model output as potential station locations, as a proxy for assessing monitoring network design and for network optimization. We find that both metrics should be used to evaluate the similarity between monitoring time series, since this allows a cross-comparison in terms of temporal variation and magnitude of concentrations to assess station potential redundancy. Here, the relative level of potential redundancy of an existing monitoring location was ranked according to each dissimilarity metric, with sites forming clusters at low values of both 1-R and Euclidean distance being the most redundant. We demonstrate clustering results depend on the air contaminant analyzed, reflecting the difference in the respective emission sources of SO 2 and NO 2 in the region under study. Our work shows that much of the signal identifying the sources of NO 2 and SO 2 emissions resides in shorter time scales (hourly to daily) due to short-term variation of concentrations. However, the methodology nevertheless identifies stations mainly influenced by seasonality, if larger time scales (weekly to monthly) are considered. We have found that data consisting of longer-term averages may lose the short-term variation needed to identify local sources, implying that long-term averaged observations are not suitable for source identification purposes. In addition to averaging time, round-off levels in data reports, and the accuracy of instrumentation were also shown to have a negative influence on the clustering results. We have performed the first dissimilarity analysis based on gridded air-quality model output, and have shown that the methodology is capable of generating maps of sub-regions within which a single station will represent the entire sub-region, to a given level of dissimilarity. Maps of this nature may be combined with other georeferenced data (e.g. road networks, power availability) to assist in monitoring network design. We have also shown that our methodology is capable of identifying different sampling methodologies, as well as identifying outliers (stations’ time series which are markedly different from all others in a given dataset).
更多查看译文
AI 理解论文
溯源树
样例
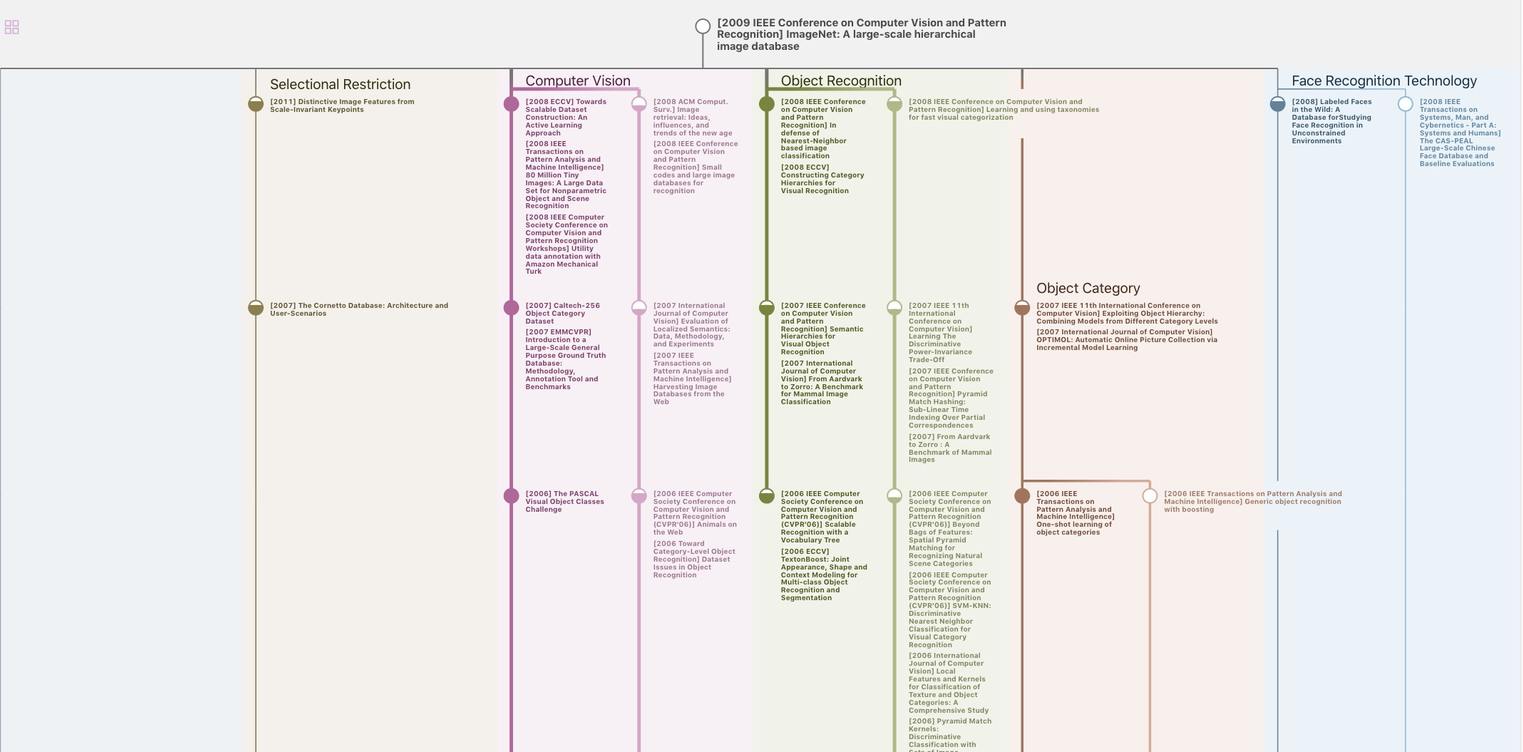
生成溯源树,研究论文发展脉络
Chat Paper
正在生成论文摘要