Fast And Accurate Vehicle Detection By Aspect Ratio Regression
2017 CHINESE AUTOMATION CONGRESS (CAC)(2017)
摘要
Traditional vehicle detectors always utilize single-template model to represent the vehicle which can not encircle vehicles with different aspect ratios. In this paper, we propose a fast and accurate approach for detecting vehicles which joints classification and aspect ratio regression. The key idea is extending the boosting decision trees method to estimate vehicle's aspect ratio during vehicle detection, and jointing regression and detection in the same cascaded framework. We propose two strategies to improve the accuracy of both detection and localization. One is to exploit a mixed error criterion for training split functions, which consider classification and regression error simultaneously, and the other is jointing classification and regression score based on their characteristics in the testing process. Furthermore, our approach doesn't bring additional calculation because the regressor shares features with the classifier, and the detector is still fast and more accurate. We collected a new dataset CFVHR that the camera is mounted on the vehicle. Experimental results on our dataset show that our approach achieves an obvious improvement compared with the ACF and LDCF detector.
更多查看译文
关键词
vehicle detection, decision trees, aspect ratio regression, classification
AI 理解论文
溯源树
样例
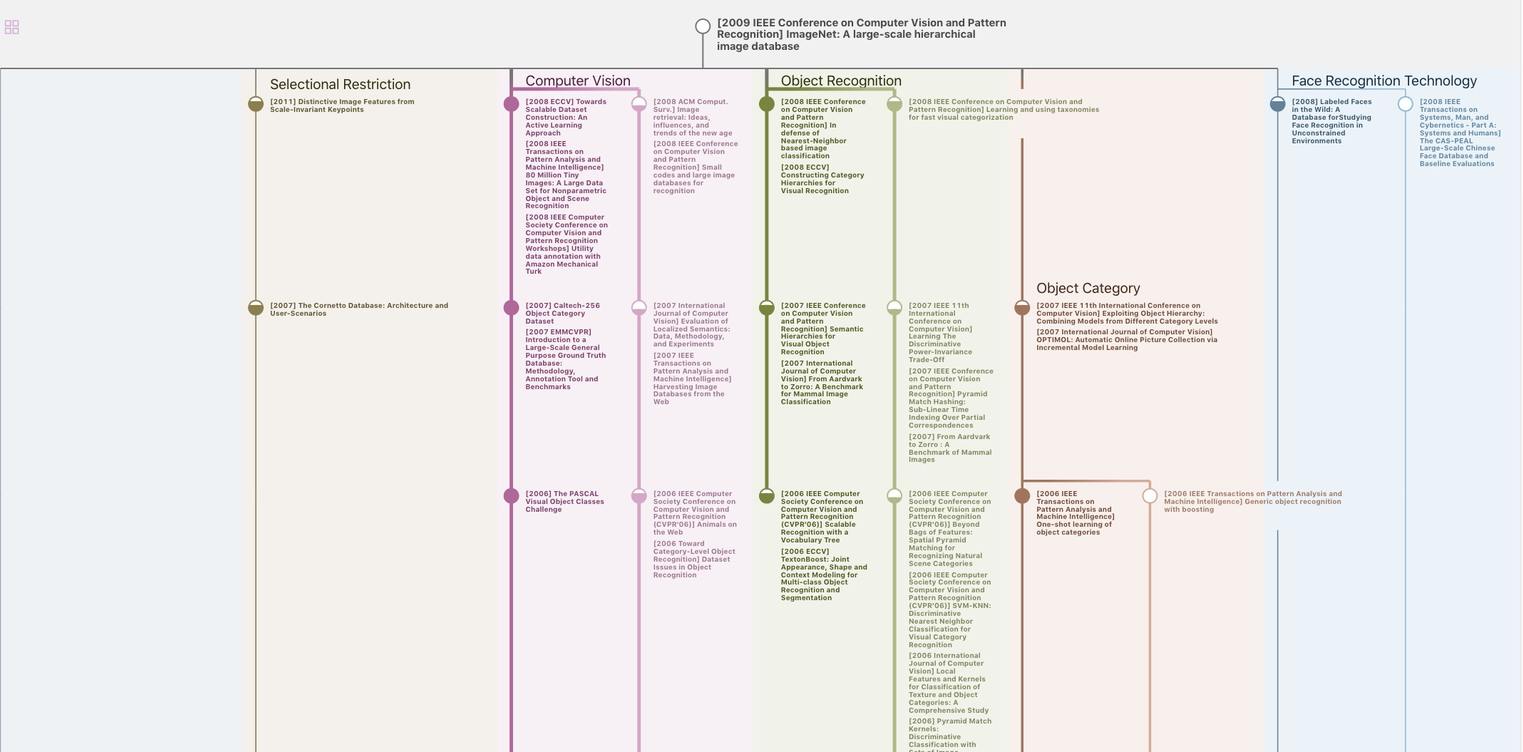
生成溯源树,研究论文发展脉络
Chat Paper
正在生成论文摘要