A Pairwise Learning To Rank Algorithm Based On Bounded Loss And Preference Weight
2017 CHINESE AUTOMATION CONGRESS (CAC)(2017)
摘要
Traditional pairwise learning to rank algorithms pay little attention to top ranked documents in the query list, and do not work well when they are used on a data set with multiple rating grades. In this paper, a novel pairwise learning to rank algorithm is proposed to solve this problem. This algorithm defines a bounded loss function and introduces the preference weights between document pairs into it. Because the batch gradient descent method will lead to slow optimization and the stochastic gradient descent method will be easily affected by noises, a mini-batch gradient descent method is proposed to optimize the algorithm, which makes the number of iteration no longer dependent on the size of samples. Finally, experiments on OHSUMED data set and MQ2008 data set demonstrate the effectiveness of the proposed algorithm.
更多查看译文
关键词
learning to rank, pairwise algorithm, bounded loss, preference weight, mini-batch gradient descent
AI 理解论文
溯源树
样例
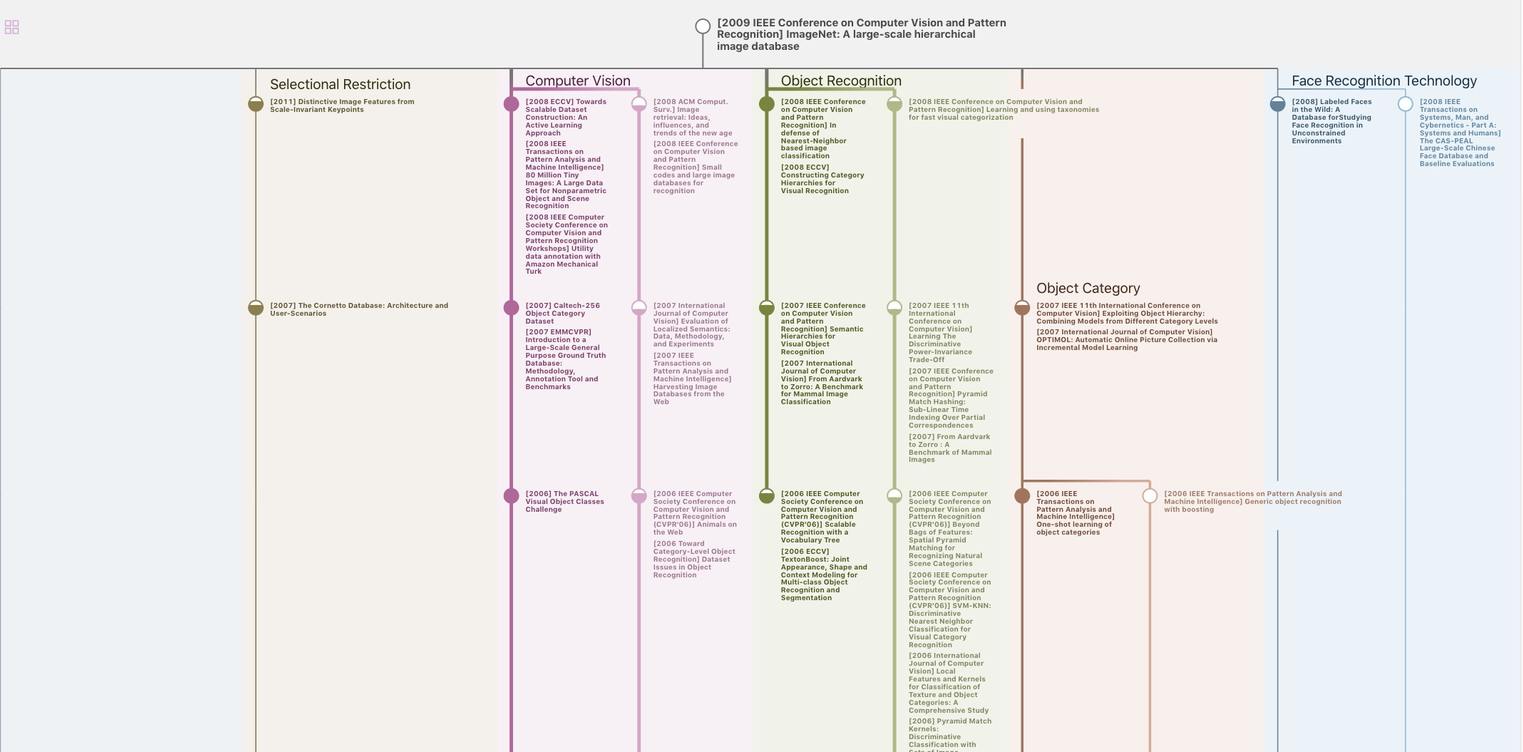
生成溯源树,研究论文发展脉络
Chat Paper
正在生成论文摘要