Comparing consensus Monte Carlo strategies for distributed Bayesian computation
BRAZILIAN JOURNAL OF PROBABILITY AND STATISTICS(2017)
摘要
Consensus Monte Carlo is an algorithm for conducting Monte Carlo based Bayesian inference on large data sets distributed across many worker machines in a data center. The algorithm operates by running a separate Monte Carlo algorithm on each worker machine, which only sees a portion of the full data set. The worker-level posterior samples are then combined to form a Monte Carlo approximation to the full posterior distribution based on the complete data set. We compare several methods of carrying out the combination, including a new method based on approximating worker-level simulations using a mixture of multivariate Gaussian distributions. We find that resampling and kernel density based methods break down after 10 or sometimes fewer dimensions, while the new mixture-based approach works well, but the necessary mixture models take too long to fit.
更多查看译文
关键词
Big data,cloud computing,Bayesian modeling
AI 理解论文
溯源树
样例
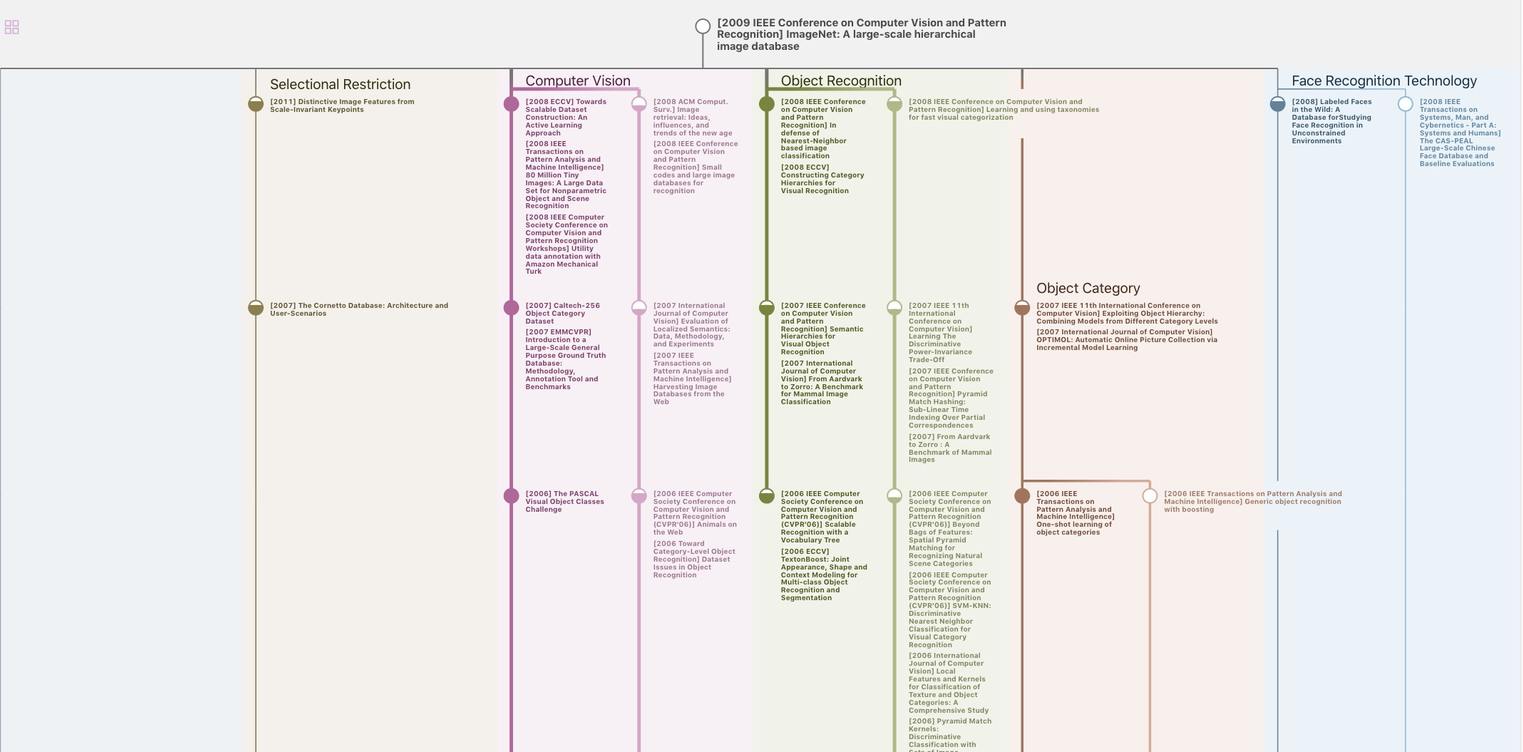
生成溯源树,研究论文发展脉络
Chat Paper
正在生成论文摘要