Evaluating a sepsis prediction machine learning algorithm using minimal electronic health record data in the emergency department and intensive care unit
bioRxiv(2018)
摘要
Introduction: Sepsis is a major health crisis in US hospitals, and several clinical identification systems have been designed to help care providers with early diagnosis of sepsis. However, many of these systems demonstrate low specificity or sensitivity, which limits their clinical utility. We evaluate the effects of a machine learning algodiagnostic (MLA) sepsis prediction and detection system using a before-and-after clinical study performed at Cabell Huntington Hospital (CHH) in Huntington, West Virginia. Prior to this study, CHH utilized the St. John9s Sepsis Agent (SJSA) as a rules-based sepsis detection system. Methods: The Predictive algoRithm for EValuation and Intervention in SEpsis (PREVISE) study was carried out between July 1, 2017 and August 30, 2017. All patients over the age of 18 who were admitted to the emergency department or intensive care units at CHH were monitored during the study. We assessed pre-implementation baseline metrics during the month of July, 2017, when the SJSA was active. During implementation in the month of August, 2017, SJSA and the MLA concurrently monitored patients for sepsis risk. At the conclusion of the study period, the primary outcome of sepsis-related in-hospital mortality and secondary outcome of sepsis-related hospital length of stay were compared between the two groups. Results: Sepsis-related in-hospital mortality decreased from 3.97% to 2.64%, a 33.5% relative decrease (P = 0.038), and sepsis-related length of stay decreased from 2.99 days in the pre-implementation phase to 2.48 days in the post-implementation phase, a 17.1% relative reduction (P u003c 0.001). Conclusion: Reductions in patient mortality and length-of-stay were observed with use of a machine learning algorithm for early sepsis detection in the emergency department and intensive care units at Cabell Huntington Hospital, and may present a method for improving patient outcomes. Trial Registration: ClinicalTrials.gov, NCT03235193, retrospectively registered on July 27th 2017.
更多查看译文
关键词
sepsis,prediction,machine learning,patient monitoring,electronic health records,alerts
AI 理解论文
溯源树
样例
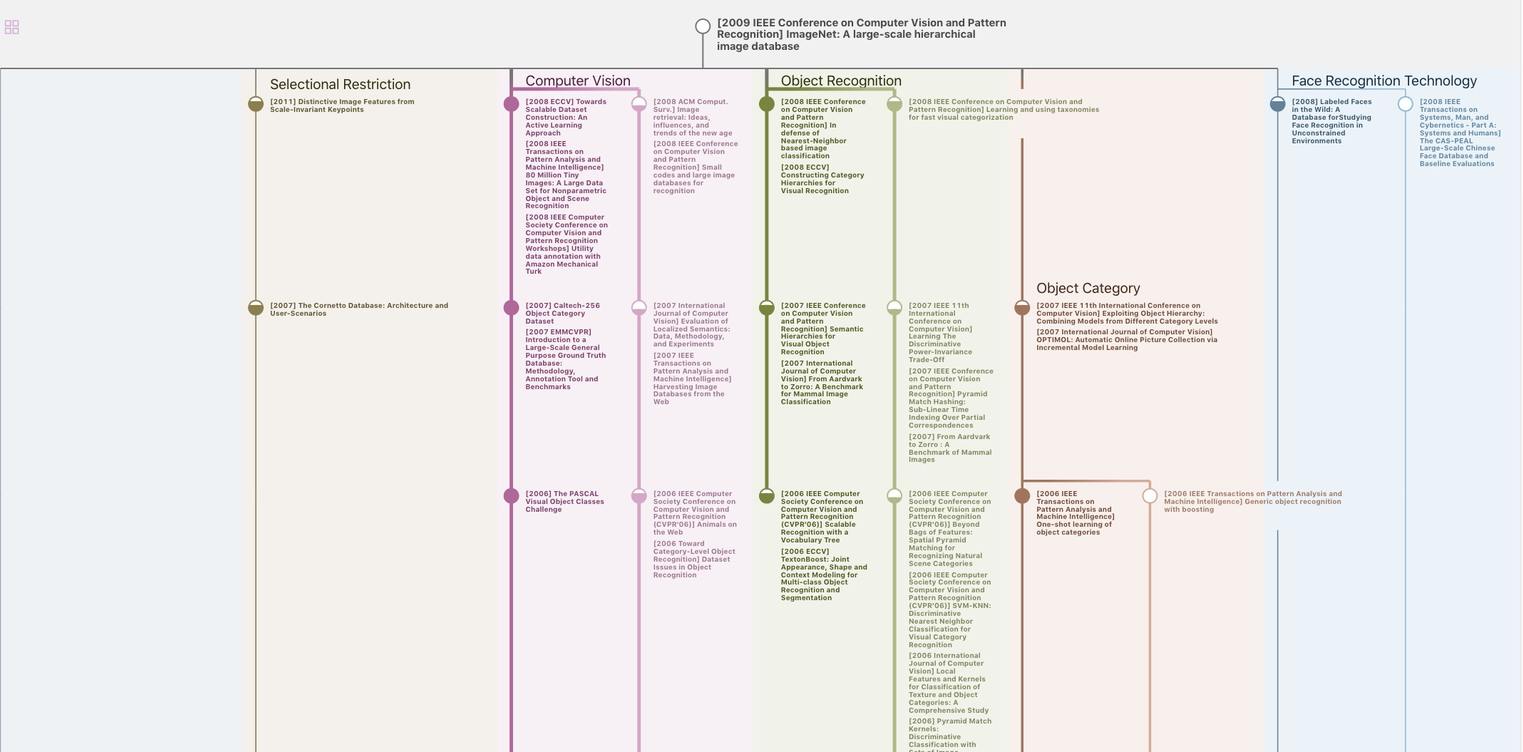
生成溯源树,研究论文发展脉络
Chat Paper
正在生成论文摘要