Fast case-based reasoning for large-scale streaming classification
8th International Conference of Pattern Recognition Systems (ICPRS 2017)(2017)
摘要
Processing high-speed big data streams is one of the most challenging tasks in machine learning nowadays. To deal with these unbounded and massive amounts of data, highly efficient methods that continuously update their structure are required. This paper aims at presenting a new incremental and distributed lazy classifier, and a distributed instance selection technique that efficiently process real-world data streams. Both algorithms have been implemented in a single system by using the Apache Spark platform. Thanks to this original design, the high computational requirements of standard lazy classifiers have been alleviated. A thorough experimental framework has been conducted on a set of big datasets, both artificial and real. Our study show the usefulness of our solutions and show that casebased reasoning can perform as a competitive option in largescale streaming environments.
更多查看译文
关键词
machine learning,data streams,big data,instance reduction,nearest neighbor,distributed computing,Apache Spark
AI 理解论文
溯源树
样例
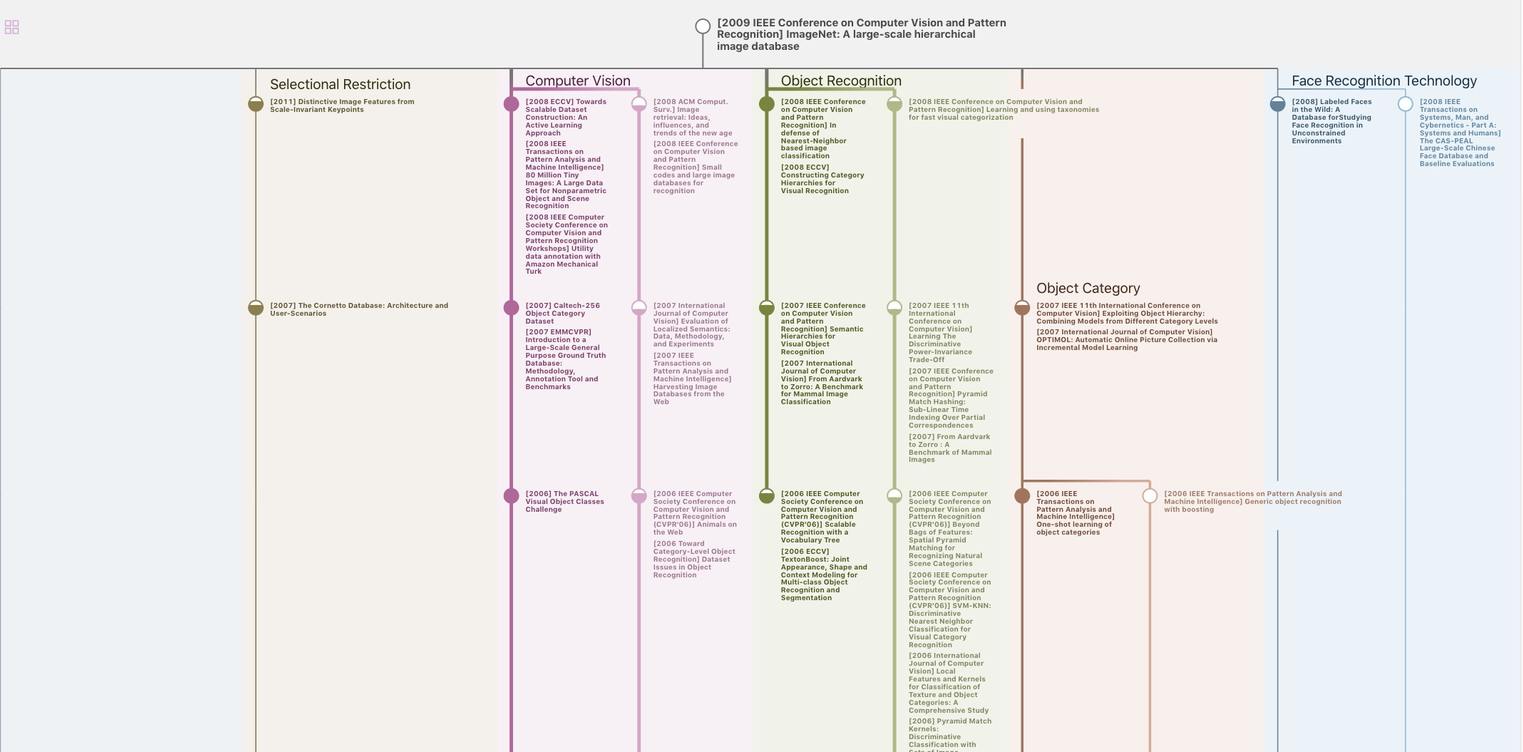
生成溯源树,研究论文发展脉络
Chat Paper
正在生成论文摘要