Improvement of land-cover classification over frequently cloud-covered areas using Landsat 8 time-series composites and an ensemble of supervised classifiers
INTERNATIONAL JOURNAL OF REMOTE SENSING(2018)
摘要
Recent abundance of moderate-to-high spatial resolution satellite imagery has facilitated land-cover map production. However, in cloud-prone areas, building high-resolution land-cover maps is still challenging due to infrequent satellite revisits and lack of cloud-free data. We propose a classification method for cloud-persistent areas with high temporal dynamics of land-cover types. First, compositing techniques are employed to create dense time-series composite images from all available Landsat 8 images. Then, spectral-temporal features are extracted to train an ensemble of five supervised classifiers. The resulting composite images are clear with at least 99.78% cloud-free pixels and are 20.47% better than their original images on average. We classify seven land classes, including paddy rice, cropland, grass/shrub, trees, bare land, impervious area, and waterbody over Hanoi, Vietnam, in 2016. Using a time series of composites significantly improves the classification performance with 10.03% higher overall accuracy (OA) compared to single composite classifications. Additionally, using time series of composites and the ensemble technique, which combines the best of five experimented classifiers (eXtreme Gradient Boosting, logistic regression, Support Vector Machine (SVM) with Radial Basis Function (RBF) kernel - SVM-RBF and Linear kernel - SVM-Linear, multilayer perceptron), performed best with 84% OA and 0.79 kappa coefficient.
更多查看译文
AI 理解论文
溯源树
样例
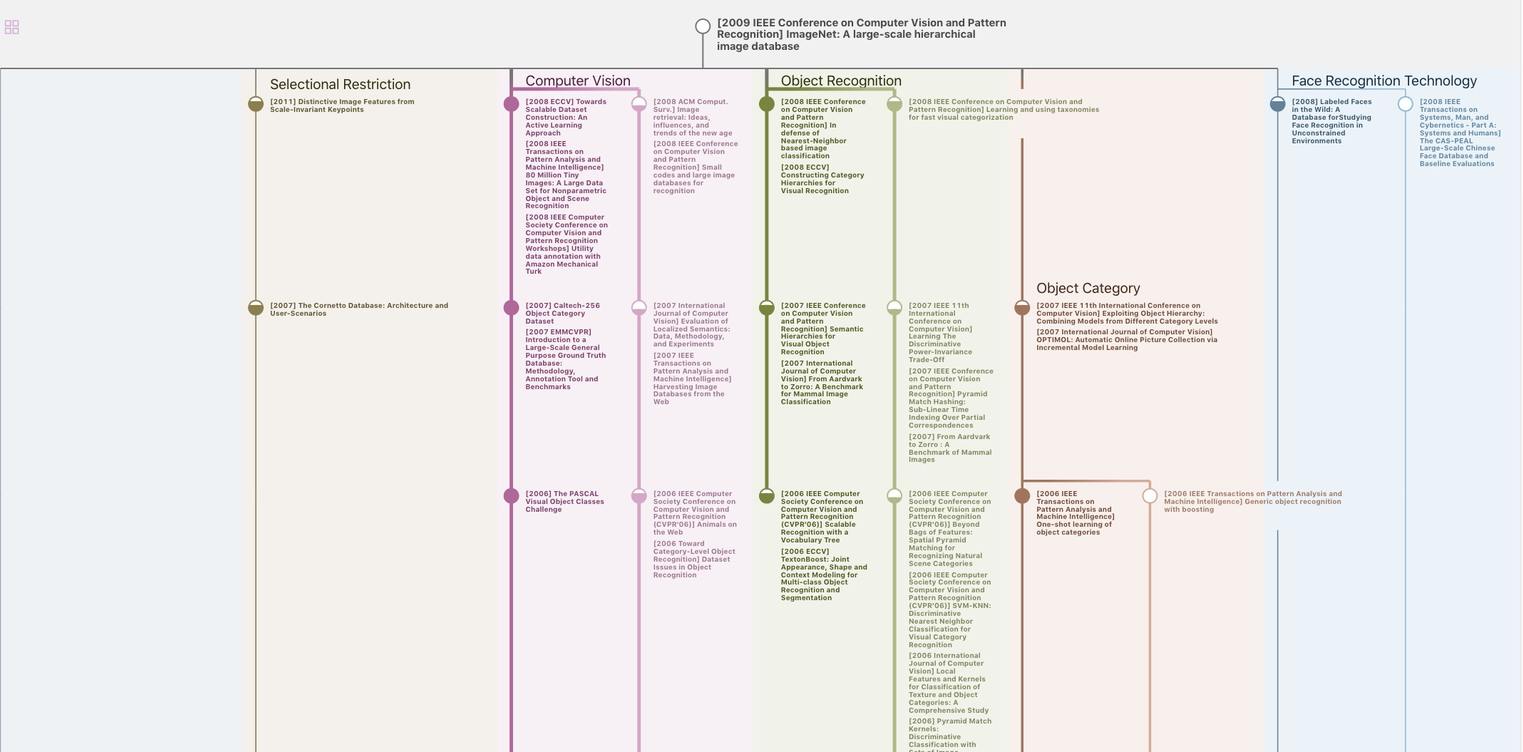
生成溯源树,研究论文发展脉络
Chat Paper
正在生成论文摘要