A Monte Carlo comparison of GMM and QMLE estimators for short dynamic panel data models with spatial errors
JOURNAL OF STATISTICAL COMPUTATION AND SIMULATION(2018)
摘要
We suggest a generalized spatial system GMM (SGMM) estimation for short dynamic panel data models with spatial errors and fixed effects when n is large and T is fixed (usually small). Monte Carlo studies are conducted to evaluate the finite sample properties with the quasi-maximum likelihood estimation (QMLE). The results show that, QMLE, with a proper approximation for initial observation, performs better than SGMM in general cases. However, it performs poorly when spatial dependence is large. QMLE and SGMM perform better for different parameters when there is unknown heteroscedasticity in the disturbances and the data are highly persistent. Both estimates are not sensitive to the treatment of initial values. Estimation of the spatial autoregressive parameter is generally biased when either the data are highly persistent or spatial dependence is large. Choices of spatial weights matrices and the sign of spatial dependence do affect the performance of the estimates, especially in the case of the heteroscedastic disturbance. We also give empirical guidelines for the model.
更多查看译文
关键词
Short dynamic panel data models,Spatial errors,Generalized spatial system GMM estimation,Quasi-maximum likelihood estimation,Monte carlo studies,62-07,62J12,62P20
AI 理解论文
溯源树
样例
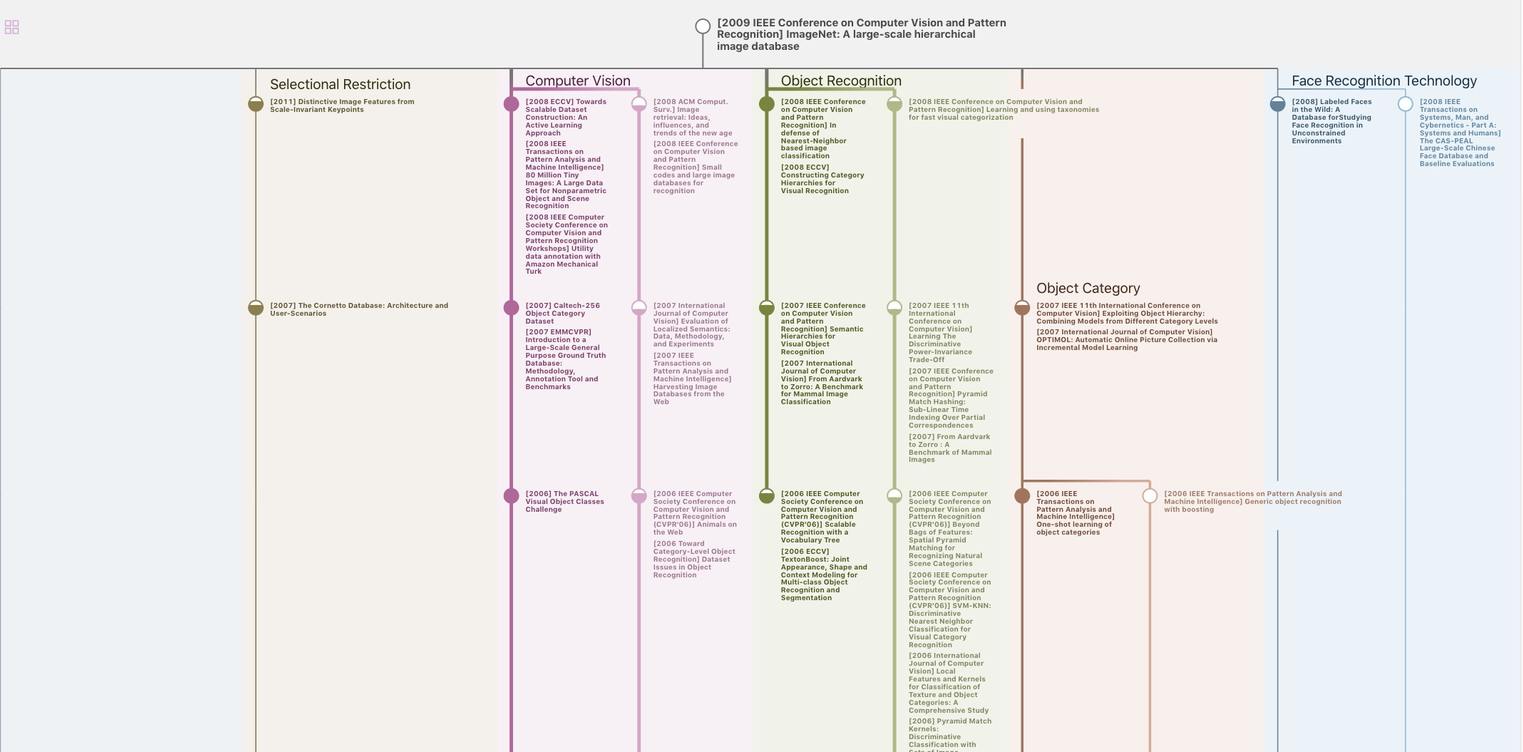
生成溯源树,研究论文发展脉络
Chat Paper
正在生成论文摘要