Functional Connectomics from Data: Probabilistic Graphical Models for Neuronal Network of C. elegans
bioRxiv(2017)
摘要
We propose a data-driven approach to represent neuronal network dynamics as a Probabilistic Graphical Model (PGM). Our approach learns the PGM structure by employing dimension reduction to network response dynamics evoked by stimuli applied to each neuron separately. The outcome model captures how stimuli propagate through the network and thus represents functional dependencies between neurons, i.e., functional connectome. The benefit of using a PGM as the functional connectome is that posterior inference can be done efficiently and circumvent the complexities in direct inference of response pathways in dynamic neuronal networks. In particular, posterior inference reveals the relations between known stimuli and downstream neurons or allows to query which stimuli are associated with downstream neurons. For validation and as an example for our approach we apply our methodology to a model of Caenorhabiditis elegans nervous system which structure and dynamics are well-studied. From its dynamical model we collect time series of the network response and use singular value decomposition to obtain a low-dimensional projection of the time series data. We then extract dominant patterns in each data matrix to get pairwise dependency information and create a graphical model for the full somatic nervous system. The PGM enables us to obtain and verify underlying neuronal pathways dominant for known behavioral scenarios and to detect possible pathways for novel scenarios.
更多查看译文
AI 理解论文
溯源树
样例
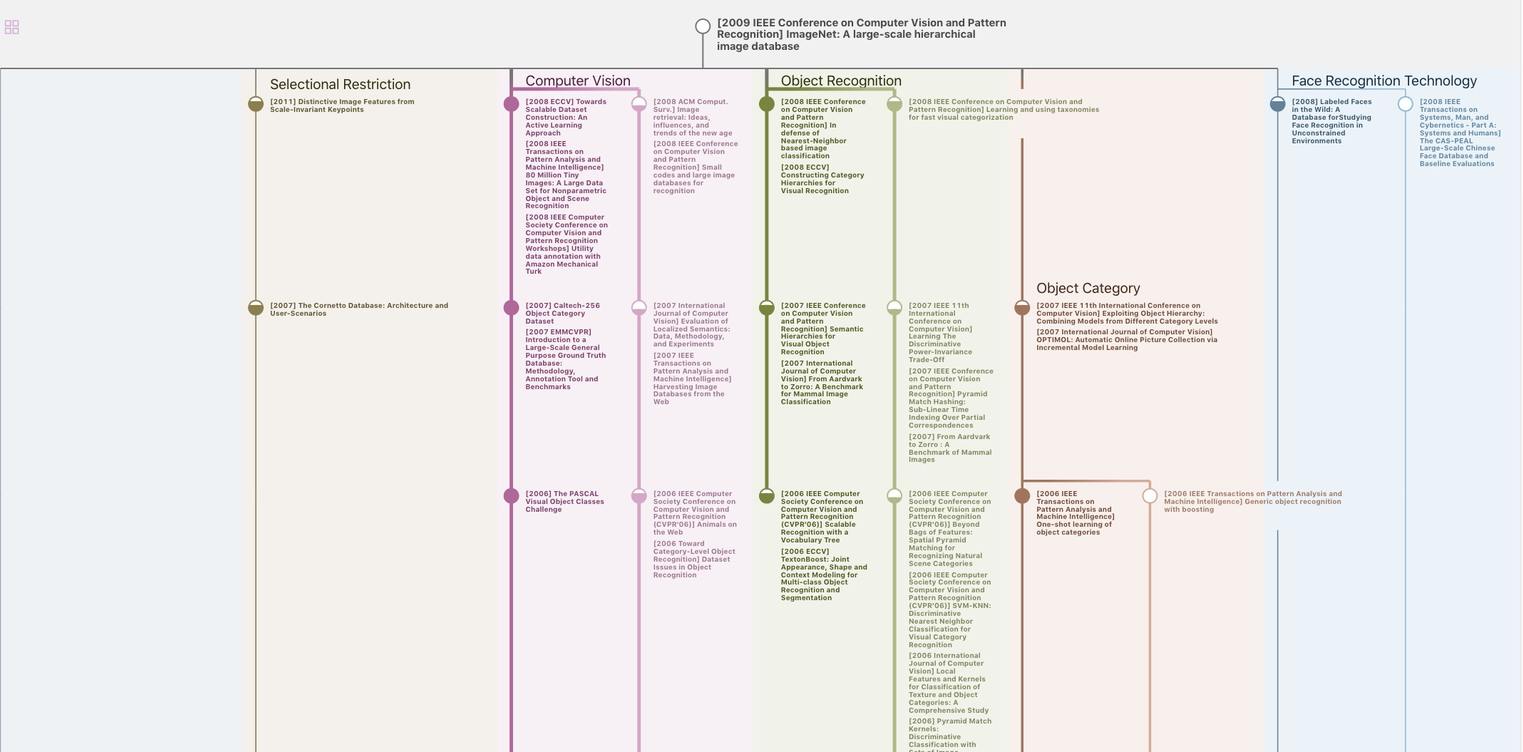
生成溯源树,研究论文发展脉络
Chat Paper
正在生成论文摘要