Online Heterogeneous Face Recognition Based on Total-Error-Rate Minimization
IEEE Transactions on Systems, Man, and Cybernetics(2020)
摘要
In this paper, we propose a recursive learning formulation for online heterogeneous face recognition (HFR). The main task is to compare between images which are acquired from different sensing spectrums for identity recognition. Using an extreme learning machine, the proposed recursive formulation seeks a direct optimization to the classification error goal where the solution converges exactly to the batch mode solution. Due to the nonlinear nature of the classification error objective function, formulation of a recursive solution that converges is an important and nontrivial task. Based on this recursive formulation, an online HFR system is designed. The system is evaluated using two challenging heterogeneous face databases with images captured under visible, near infrared and infrared spectrums. The proposed system shows promising performance which is comparable with that of competing state-of-the-arts.
更多查看译文
关键词
Feature extraction,Minimization,Visualization,Face recognition,Image recognition,Surveillance,Principal component analysis
AI 理解论文
溯源树
样例
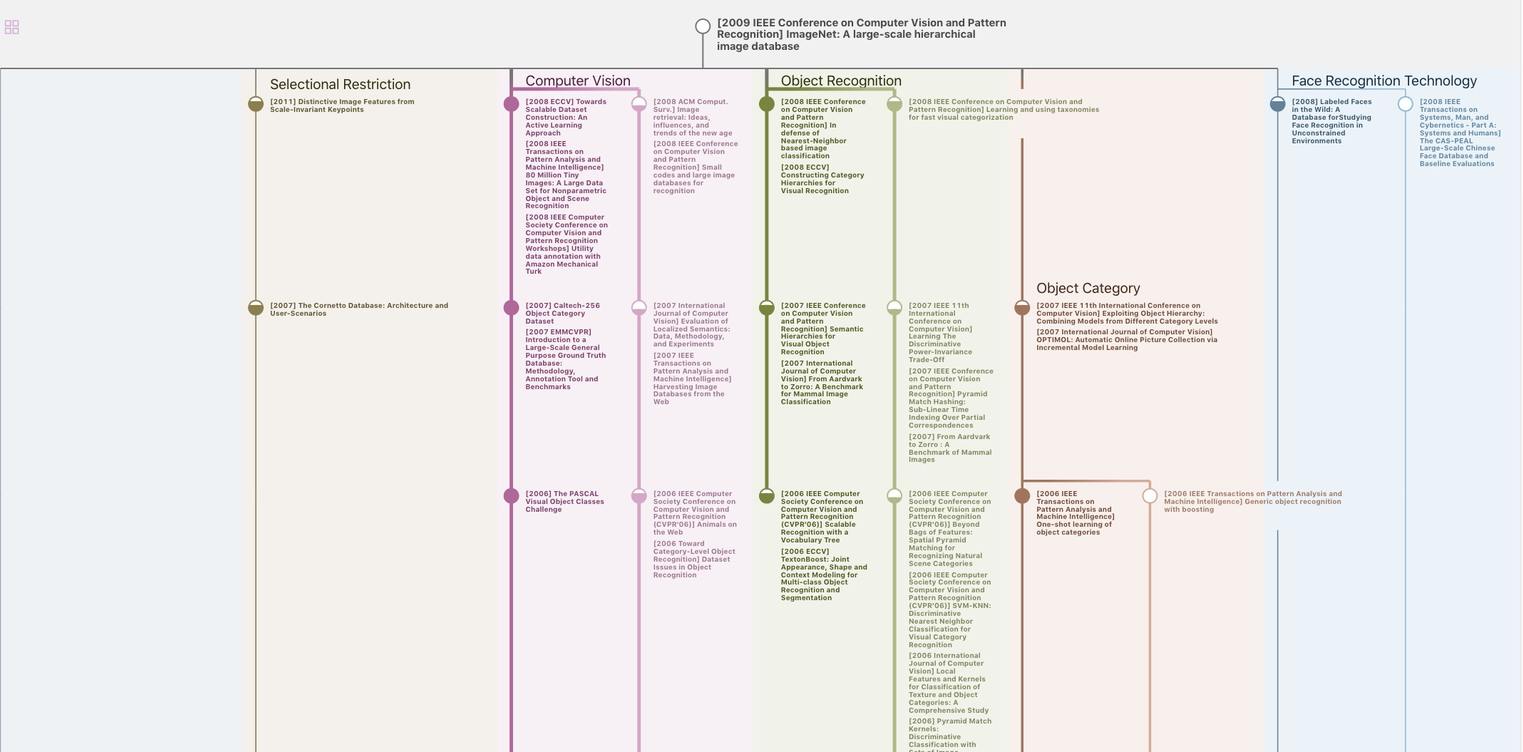
生成溯源树,研究论文发展脉络
Chat Paper
正在生成论文摘要