Efficiently Evaluating Targeting Policies Using Field Experiments
Social Science Research Network(2020)
摘要
Champion versus challenger field experiments are widely used to compare the performance of different targeting policies. These experiments randomly assign customers to receive marketing actions recommended by either the existing (champion) policy or the new (challenger) policy, and then compare the aggregate outcomes. We recommend an alternative experimental design and propose an alternative estimation approach to improve the evaluation of targeting policies. The recommended experimental design randomly assigns customers to marketing actions. This allows evaluation of any targeting policy without requiring an additional experiment, including policies designed after the experiment is implemented. The proposed estimation approach identifies customers for whom different policies recommend the same action and recognizes that for these customers there is no difference in performance. This allows for a more precise comparison of the policies. We illustrate the advantages of the experimental design and estimation approach using data from an actual field experiment. We also demonstrate that the grouping of customers, which is the foundation of our estimation approach, can help to improve the training of new targeting policies.
更多查看译文
关键词
targeting,field experiments,machine learning,counterfactual policy logging,policy evaluation
AI 理解论文
溯源树
样例
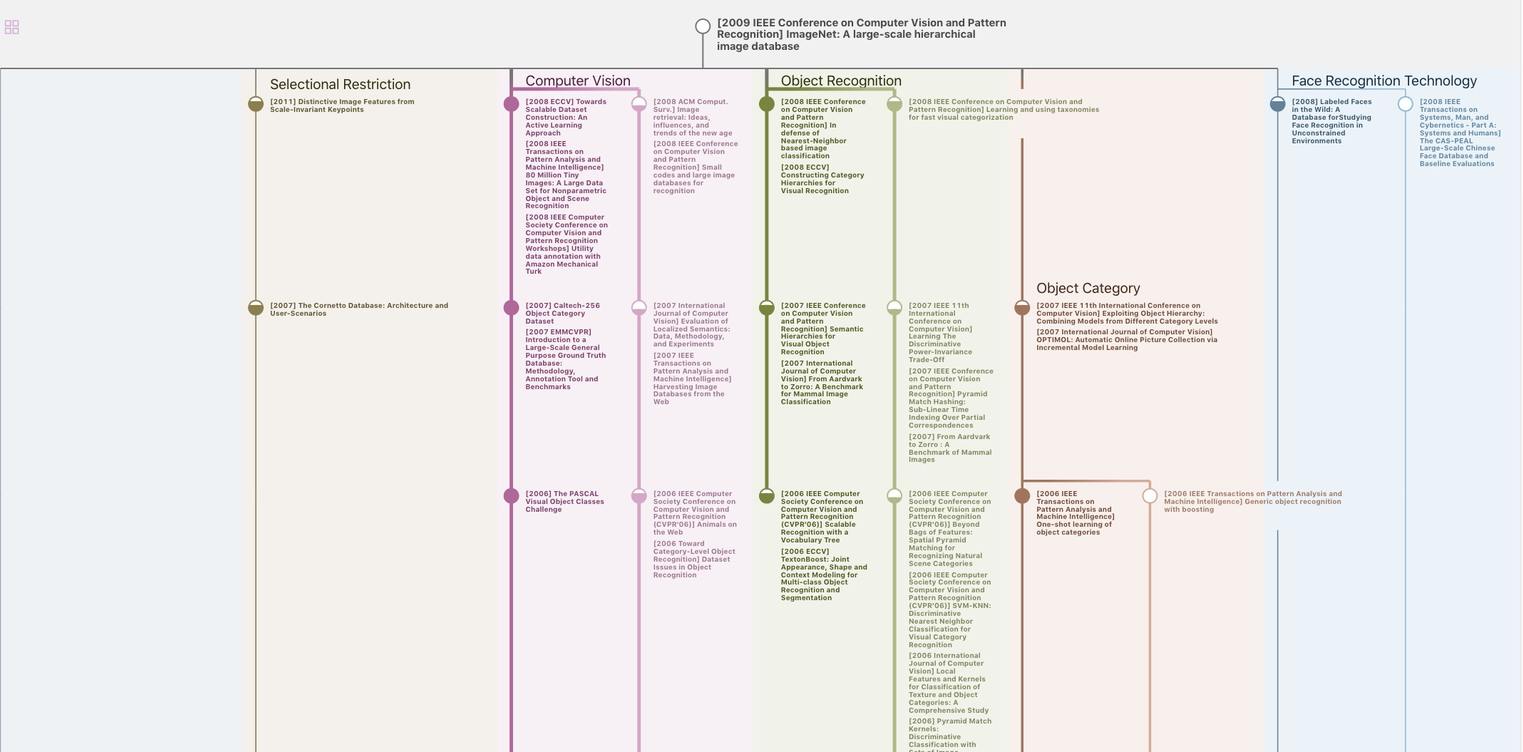
生成溯源树,研究论文发展脉络
Chat Paper
正在生成论文摘要