Adaptive Clustering Using Kernel Density Estimators
JOURNAL OF MACHINE LEARNING RESEARCH(2023)
摘要
We derive and analyze a generic, recursive algorithm for estimating all splits in a finite cluster tree as well as the corresponding clusters. We further investigate statistical properties of this generic clustering algorithm when it receives level set estimates from a kernel density estimator. In particular, we derive finite sample guarantees, consistency, rates of convergence, and an adaptive data-driven strategy for choosing the kernel bandwidth. For these results we do not need continuity assumptions on the density such as Holder continuity, but only require intuitive geometric assumptions of non-parametric nature. In addition, we compare our results to other guarantees found in the literature and also present some experiments comparing our algorithm to k-means and hierarchical clustering.
更多查看译文
关键词
cluster analysis,kernel density estimation,consistency,rates,adaptivity
AI 理解论文
溯源树
样例
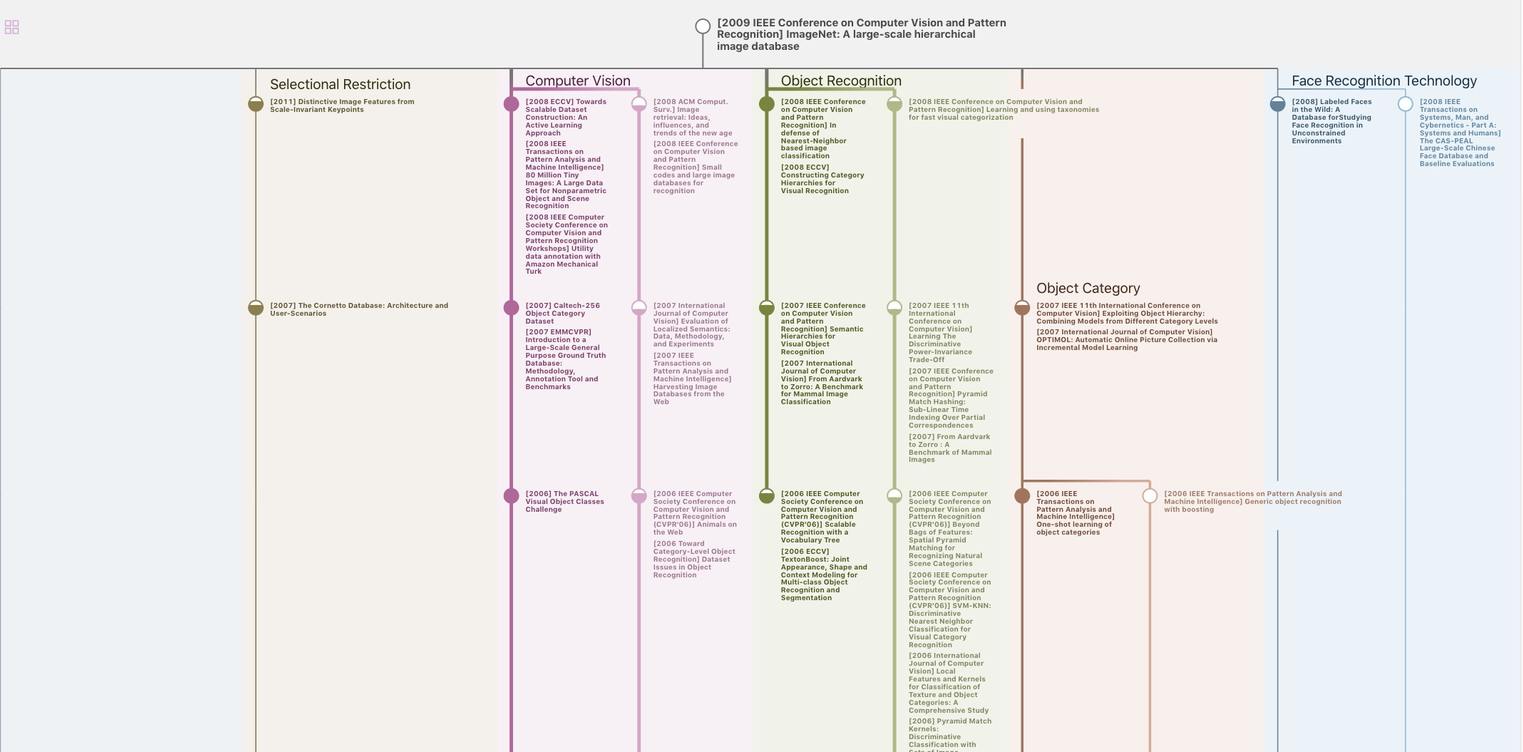
生成溯源树,研究论文发展脉络
Chat Paper
正在生成论文摘要