Supervised Vocal-Based Emotion Recognition Using Multiclass Support Vector Machine, Random Forests, and Adaboost
JOURNAL OF THE AUDIO ENGINEERING SOCIETY(2017)
摘要
This paper investigates and compares three different classifiers-multi-class Support Vector Machine, Adaboost, and random forests-for the purpose of vocal emotion recognition. Additionally, the decisions of all classifiers are combined using majority voting. The proposed method has been applied to two different emotional databases, which are the Surrey Audio-Visual Expressed Emotion Database and the Polish Emotional Speech Database. Fourteen features, namely pitch, intensity, first through fourth formants and their bandwidths, mean autocorrelation, mean noise-to-harmonics ratio, mean harmonics-to-noise ratio, and standard deviation have been extracted from both databases. Best recognition rate on the Surrey Audio-Visual Expressed Emotion Database has been achieved for the random forest algorithm, which is 75.71%. On the Polish Emotional Speech Database the best recognition rate has been achieved by applying the Adaboost classifier, which is 87.5%. The achieved recognition rates are higher than presented by Yuncu et al. on the same databases (73.81% on the Surrey Audio-Visual Expressed Emotion Database and 71.30% on the Polish Emotional Speech database).
更多查看译文
AI 理解论文
溯源树
样例
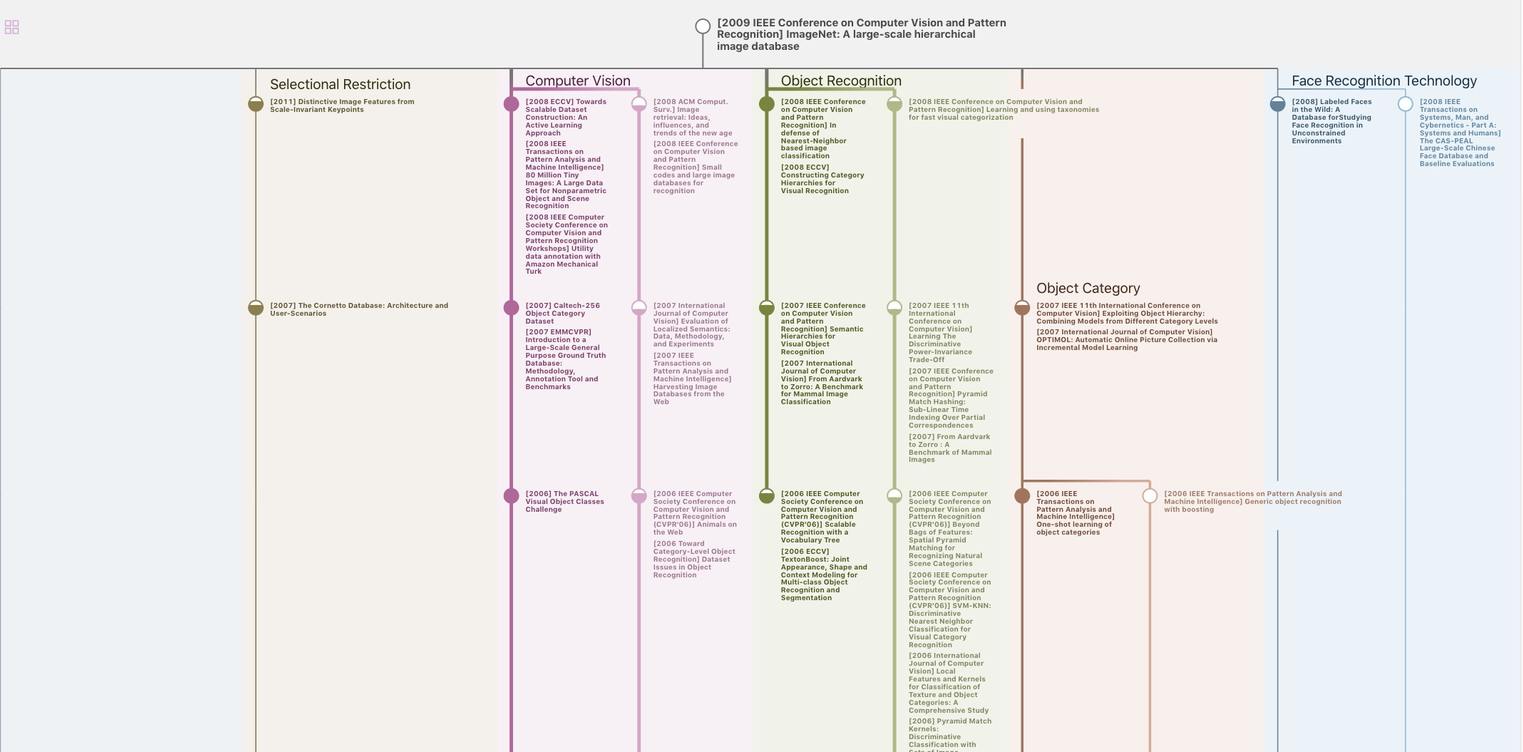
生成溯源树,研究论文发展脉络
Chat Paper
正在生成论文摘要