02.16 Machine learning of flow cytometry data encompassing seven systemic autoimmune diseases and controls
Annals of the Rheumatic Diseases(2017)
摘要
Background PRECISESADS 1 is an innovative medicine initiative project that is studying systemic autoimmune disease (SAD) patients using OMICs as well as multi-parameter flow cytometry in order to molecularly reclassify SADs. Here, we report on the results of supervised and unsupervised learning of nine flow cytometry panels each containing seven or eight cell surface markers that delineate 357 cellular population signatures across 298 individuals encompassing controls and seven SADs; mixed connective tissue disease (MCTD), primary antiphospholipid syndrome (PAPS), rheumatoid arthritis (RA), systemic lupus erythematosus (SLE), systemic sclerosis (Ssc), Sjogren’s syndrome (Sjs) and undifferentiated connective tissue disease (UCTD). Materials and methods In order to find the most relevant cellular populations differentiating each disease from controls, 10-fold cross-validated Boruta 2 feature selection was used in which a feature was deemed significant only if it was considered a relevant feature in each of the ten cross-validation folds. The features for each disease were then ranked using an ensemble of models. The cross-validated feature selection process was also coupled with random forest 3 classification to judge how predictive the disease-specific features are. Then all features were joined into a single matrix and filtered of controls in order to cluster and to visually reveal relationships through force-directed networks and t-Distributed Stochastic Neighbour Embedding. 4 Results Feature selection provided a list of relevant cellular populations for each disease that both agree with previous literature and point to previously unidentified SAD drivers. Random forest classification yielded high accuracy and moderate to substantial Cohen’s kappa agreement for each disease, respectively. Clustering and network building using the combined feature matrix showed that while intra-disease micro-clusters frequently occur, SAD clusters are also highly heterogeneous. Conclusions We leveraged the power of machine learning to data-mine a comprehensive list of SAD-pertinent cell populations. Our results verify well-documented relationships between specific cell populations and SADs and also reveal new relationships that warrant further investigation. Lastly, clustering and visualising the diseases using the relevant cell populations gives new insight into the inter-disease relationships of the highly heterogeneous SADs as well as providing valuable information for future studies that will come out of the PRECISESADS project. Funding This work has received support from the EU/EFPIA/Innovative Medicines Initiative Joint Undertaking PRECISESADS grant n° 1 15 565. References Hofmann-Apitius M, Alarcon-Riquelme ME, Chamberlain C and McHale D. “Towards the taxonomy of human disease.” Nat Rev Drug Discov . 2015;14(2):75–6. Miron B. Kursa and Witold R. Rudnicki. “Feature Selection with the Boruta Package.” Journal of Statistical Software . 2010;6(11):1–13. Leo Breiman. “Random Forests.” Machine Learning . 2001 ;45(1):5–32. van der Maaten LJP and Hinton GE. “Visualising high-dimensional data using t-SNE.” J Mach Learn Res . 2008;9:2579–2605.
更多查看译文
关键词
flow cytometry data,systemic autoimmune diseases,autoimmune diseases,machine learning
AI 理解论文
溯源树
样例
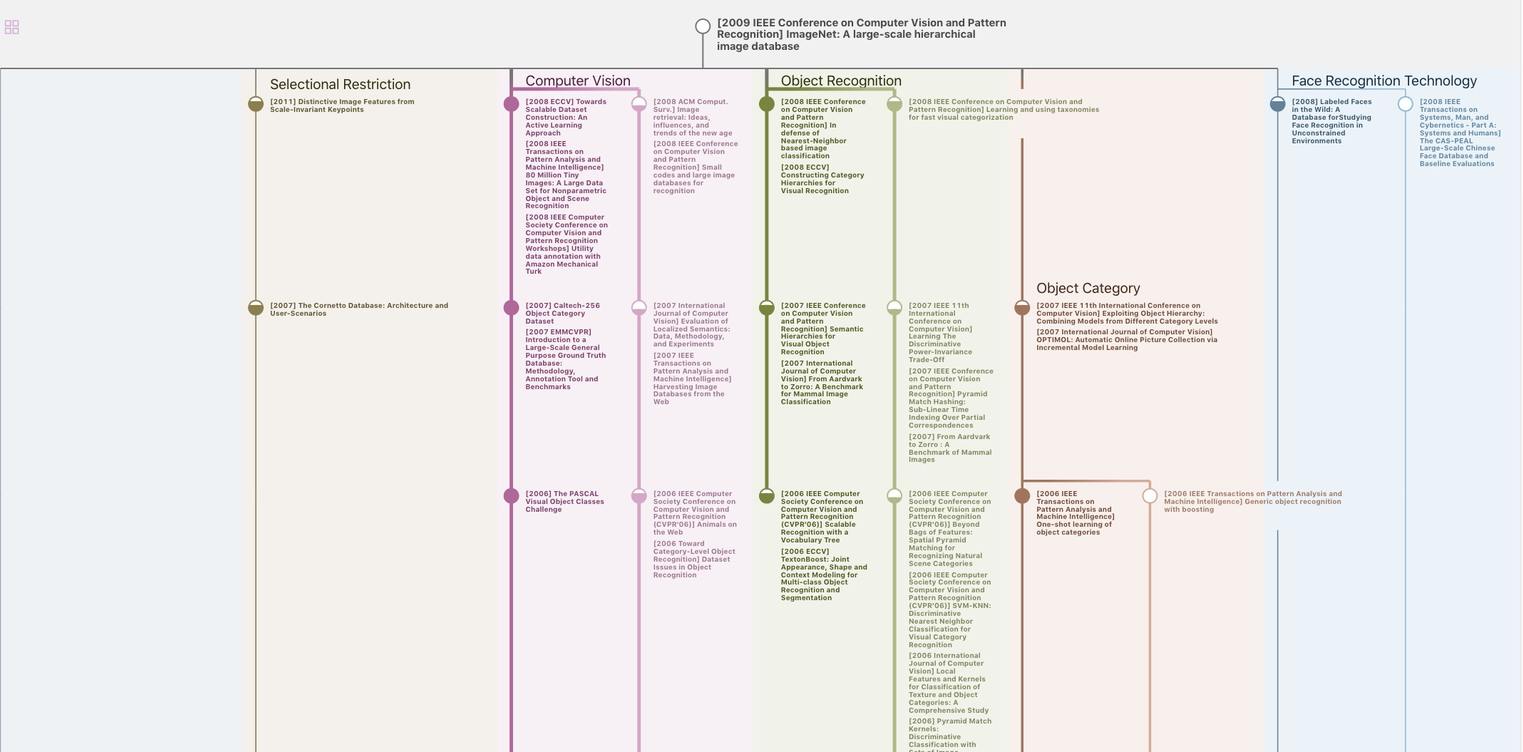
生成溯源树,研究论文发展脉络
Chat Paper
正在生成论文摘要