An Integrated Genomic And Proteomic Analysis Of Human Tumors Reveals Key Factors In Neoantigen Identification And Enables Epitope Prediction For Cancer Immunotherapy
CANCER RESEARCH(2017)
摘要
Background: Immunotherapy has become essential in the treatment of advanced cancer, with the advent of drug approvals in multiple indications and functional cures for a minority of patients. However, overall survival gains from immunotherapy have been limited and substantial progress remains. The discovery of tumor-specific neoantigens as principal targets of T cells unleashed by immune checkpoint blockade was a key breakthrough in immunotherapy research. Although example neoantigens have been reported, the impact of genomic features on HLA presentation and neoantigen identification has not been comprehensively assessed. Moreover, in silico methods for predicting which mutations lead to neoantigens are currently inadequate for most therapeutic applications, with a false positive rate of u003e95%. Methods: We performed deep tumor/normal exome sequencing (Illumina-based, average unique depth 557x/149x), tumor transcriptome sequencing (avg. 111M unique reads), and mass spec HLA class I peptide sequencing (Thermo Lumos, avg. 3,482 peptides) on fresh-frozen primary tumor specimens from 23 patients (22 NSCLC, 1 OC). Somatic mutations were called with a custom GATK-based pipeline, gene expression assessed with RSEM, and peptides matched to mass spectra using COMET/Percolator. We examined the effect of NGS parameters on neoantigen identification, analyzed HLA presentation as a function of genomic features, and created a novel deep learning model for epitope prediction. Results: We identified 6,252 nonsynonymous somatic mutations across the cohort (avg. 272 per patient) and found 3,874 (62%) transcribed in mRNA. Down-sampling tumor DNA NGS data to a more typical average unique coverage of ~200x and RNA to ~60M reads revealed 3,275 transcribed mutations, an overall 15% loss of candidate neoantigens and u003e25% loss in low tumor content samples, demonstrating the importance of sequencing depth. Gene expression measurement at the RNA level predicted HLA presentation more strongly than expected, with peptides from genes detected in RNA found presented u003e20X more often than peptides from non-detected genes. With this and public datasets we trained a model to predict HLA presentation and compared it to standard binding affinity prediction (e.g., NetMHC or MHCflurry) at the strong-binder 50nM threshold for well-characterized alleles. Whereas binding affinity-based prediction gave a positive predictive value (PPV) of only 3%, our integrated deep learning approach showed a PPV of u003e25% at equivalent recall, a near ten-fold gain. Conclusions: A novel genomic and proteomic analysis of human tumors establishes NGS requirements for neoantigen identification, characterizes key correlates of HLA presentation, and enables prediction for personalized cancer immunotherapy research. Citation Format: Brendan Bulik-Sullivan, Jennifer Busby, Matthew Davis, Andrew Clark, Tyler Murphy, Michele Busby, Fujiko Duke, Jessica Smith, Jason Hudak, Adnan Derti, Josh Francis, Roman Yelensky. An integrated genomic and proteomic analysis of human tumors reveals key factors in neoantigen identification and enables epitope prediction for cancer immunotherapy [abstract]. In: Proceedings of the American Association for Cancer Research Annual Meeting 2017; 2017 Apr 1-5; Washington, DC. Philadelphia (PA): AACR; Cancer Res 2017;77(13 Suppl):Abstract nr 629. doi:10.1158/1538-7445.AM2017-629
更多查看译文
AI 理解论文
溯源树
样例
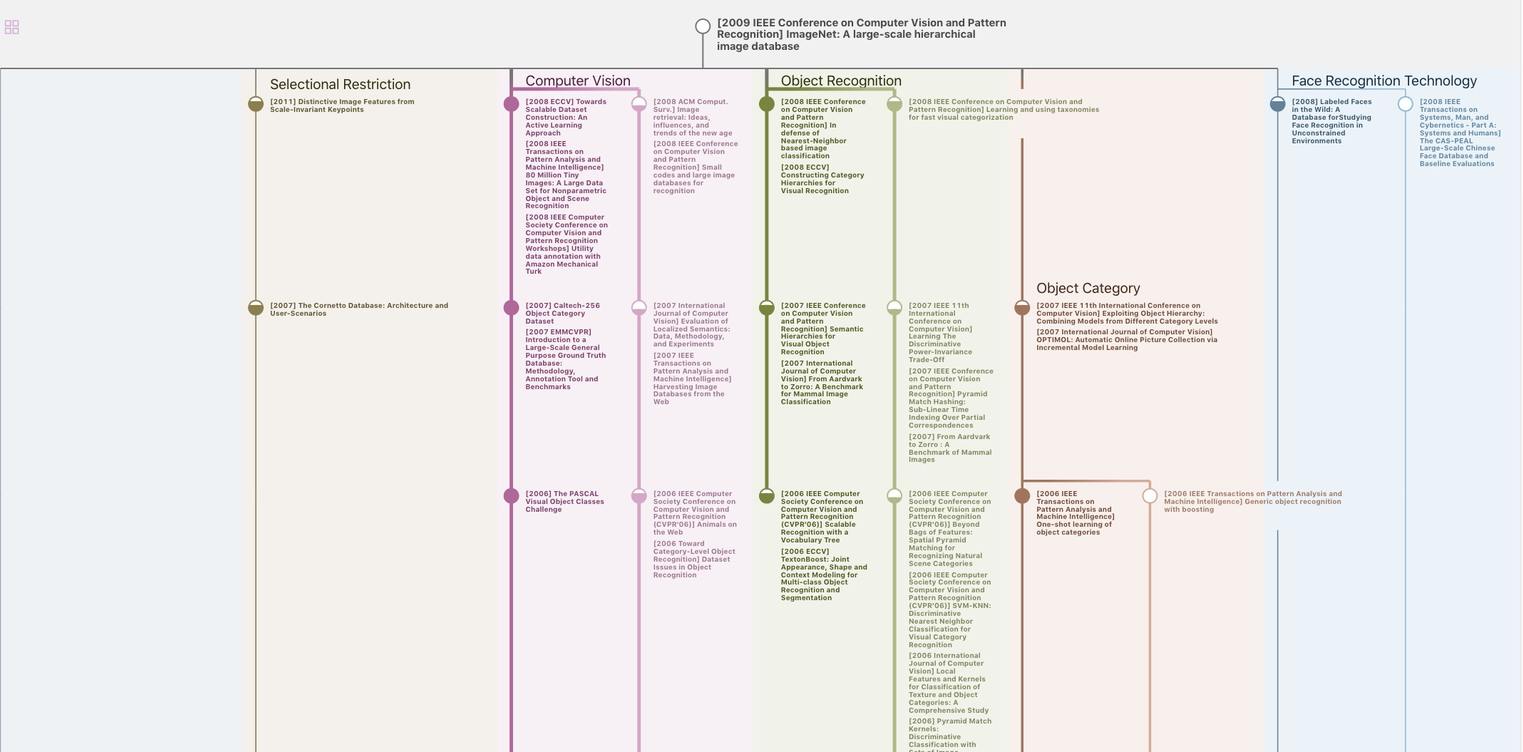
生成溯源树,研究论文发展脉络
Chat Paper
正在生成论文摘要