Prompt Programming for Large Language Models: Beyond the Few-Shot Paradigm
Conference on Human Factors in Computing Systems(2021)
摘要
BSTRACT Prevailing methods for mapping large generative language models to supervised tasks may fail to sufficiently probe models’ novel capabilities. Using GPT-3 as a case study, we show that 0-shot prompts can significantly outperform few-shot prompts. We suggest that the function of few-shot examples in these cases is better described as locating an already learned task rather than meta-learning. This analysis motivates rethinking the role of prompts in controlling and evaluating powerful language models. We discuss methods of prompt programming, emphasizing the usefulness of considering prompts through the lens of natural language. We explore techniques for exploiting the capacity of narratives and cultural anchors to encode nuanced intentions and techniques for encouraging deconstruction of a problem into components before producing a verdict. Informed by this more encompassing theory of prompt programming, we also introduce the idea of a metaprompt that seeds the model to generate its own natural language prompts for a range of tasks. Finally, we discuss how these more general methods of interacting with language models can be incorporated into existing and future benchmarks and practical applications.
更多查看译文
关键词
language models, transformers, GPT-3, few-shot learning, prompt programming, metaprompts, serial reasoning, semiotics
AI 理解论文
溯源树
样例
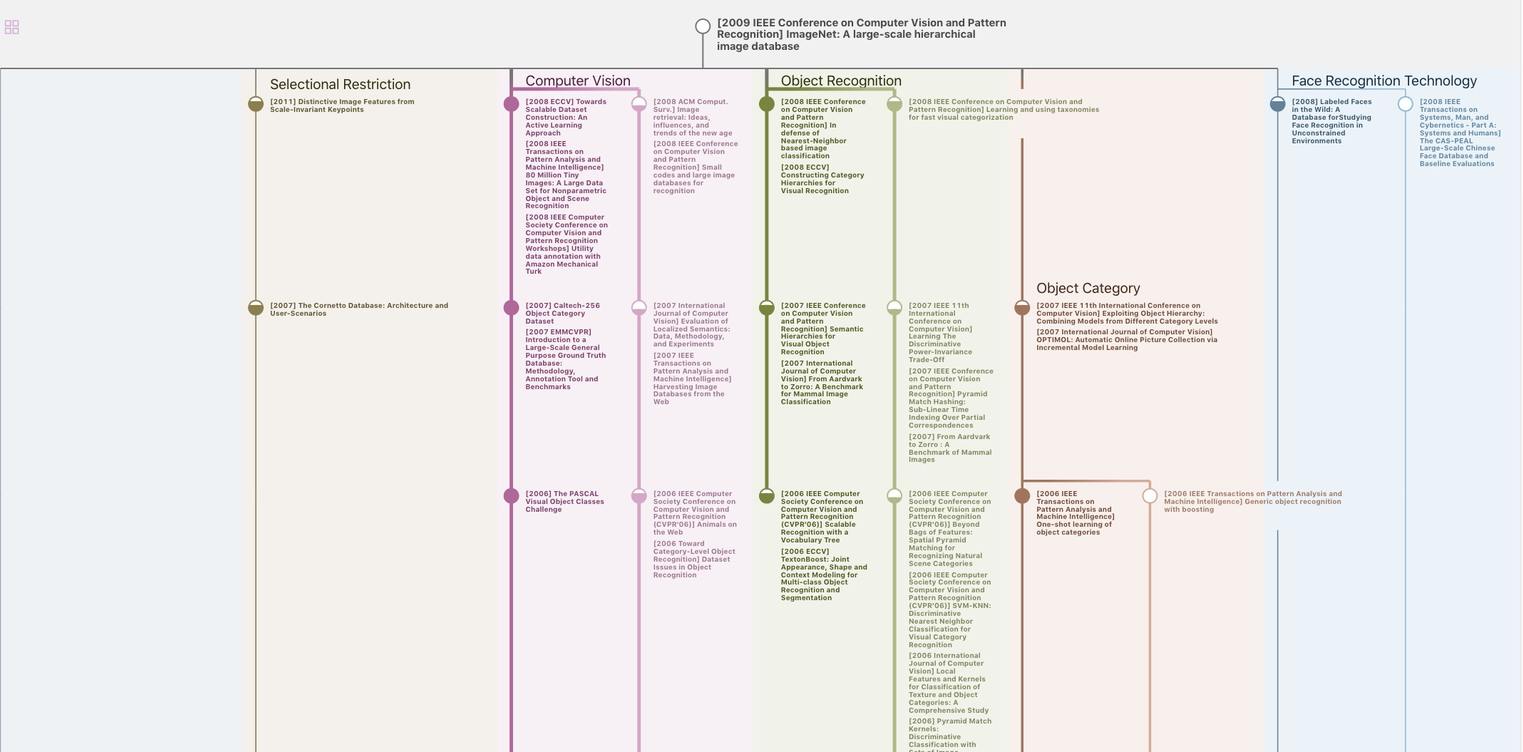
生成溯源树,研究论文发展脉络
Chat Paper
正在生成论文摘要