On a simple method for testing independencies in Bayesian networks: Testing Independencies in Bayesian Networks
COMPUTATIONAL INTELLIGENCE(2018)
摘要
Testing independencies is a fundamental task in reasoning with Bayesian networks (BNs). In practice, d-separation is often used for this task, since it has linear-time complexity. However, many have had difficulties understanding d-separation in BNs. An equivalent method that is easier to understand, called m-separation, transforms the problem from directed separation in BNs into classical separation in undirected graphs. Two main steps of this transformation are pruning the BN and adding undirected edges. In this paper, we propose u-separation as an even simpler method for testing independencies in a BN. Our approach also converts the problem into classical separation in an undirected graph. However, our method is based upon the novel concepts of inaugural variables and rationalization. Thereby, the primary advantage of u-separation over m-separation is that m-separation can prune unnecessarily and add superfluous edges. Our experiment results show that u-separation performs 73% fewer modifications on average than m-separation.
更多查看译文
关键词
Bayesian networks,d-separation,m-separation,testing independencies
AI 理解论文
溯源树
样例
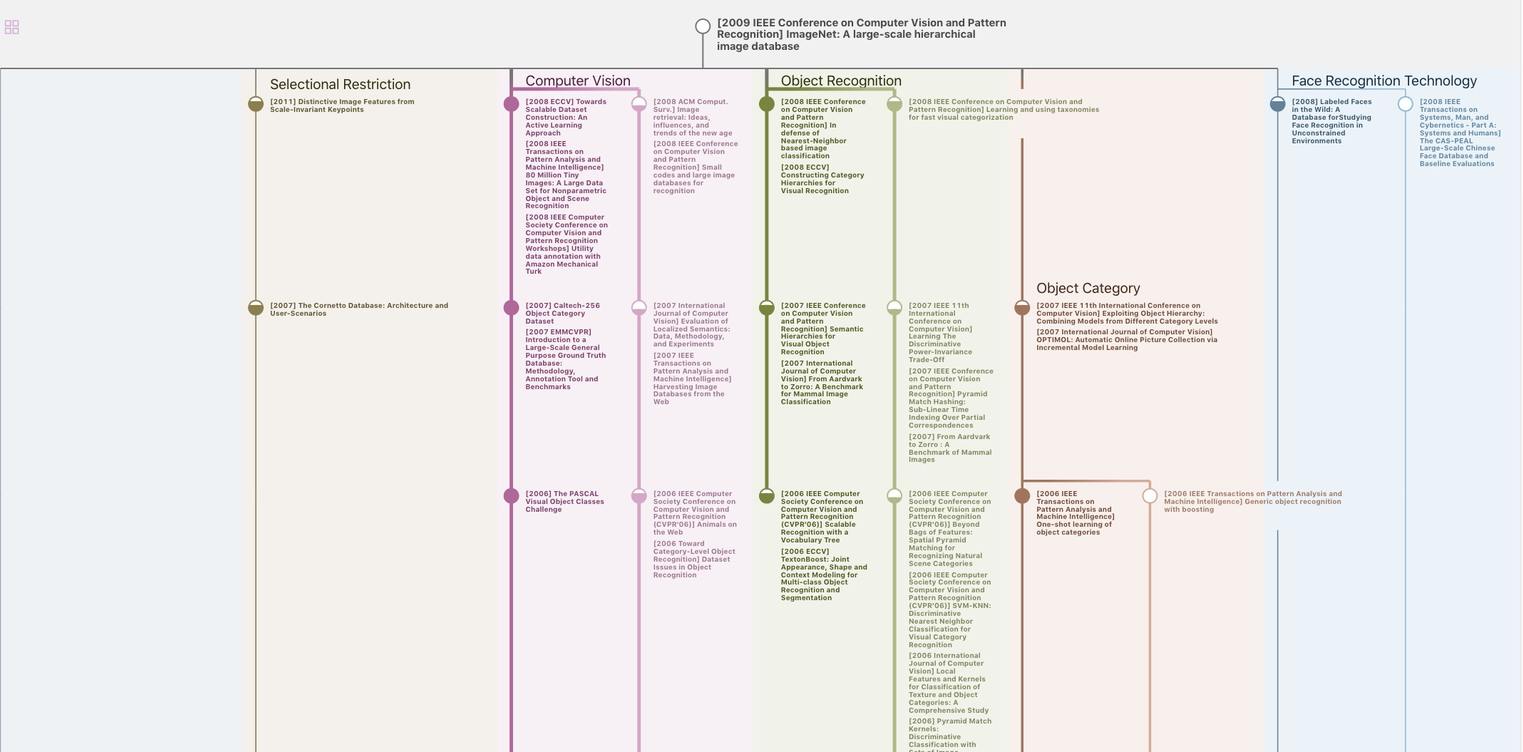
生成溯源树,研究论文发展脉络
Chat Paper
正在生成论文摘要