A method of minimum volume simplex analysis constrained unmixing for hyperspectral image
Proceedings of SPIE(2017)
摘要
The signal recorded by a low resolution hyperspectral remote sensor from a given pixel, letting alone the effects of the complex terrain, is a mixture of substances. To improve the accuracy of classification and sub-pixel object detection, hyperspectral unmixing(HU) is a frontier-line in remote sensing area. Unmixing algorithm based on geometric has become popular since the hyperspectral image possesses abundant spectral information and the mixed model is easy to understand. However, most of the algorithms are based on pure pixel assumption, and since the non-linear mixed model is complex, it is hard to obtain the optimal endmembers especially under a highly mixed spectral data. To provide a simple but accurate method, we propose a minimum volume simplex analysis constrained (MVSAC) unmixing algorithm. The proposed approach combines the algebraic constraints that are inherent to the convex minimum volume with abundance soft constraint. While considering abundance fraction, we can obtain the pure endmember set and abundance fraction correspondingly, and the final unmixing result is closer to reality and has better accuracy. We illustrate the performance of the proposed algorithm in unmixing simulated data and real hyperspectral data, and the result indicates that the proposed method can obtain the distinct signatures correctly without redundant endmember and yields much better performance than the pure pixel based algorithm.
更多查看译文
关键词
hyperspectral image,unmixing,endmember extraction,abundance estimation,spectral analysis,remote sensing
AI 理解论文
溯源树
样例
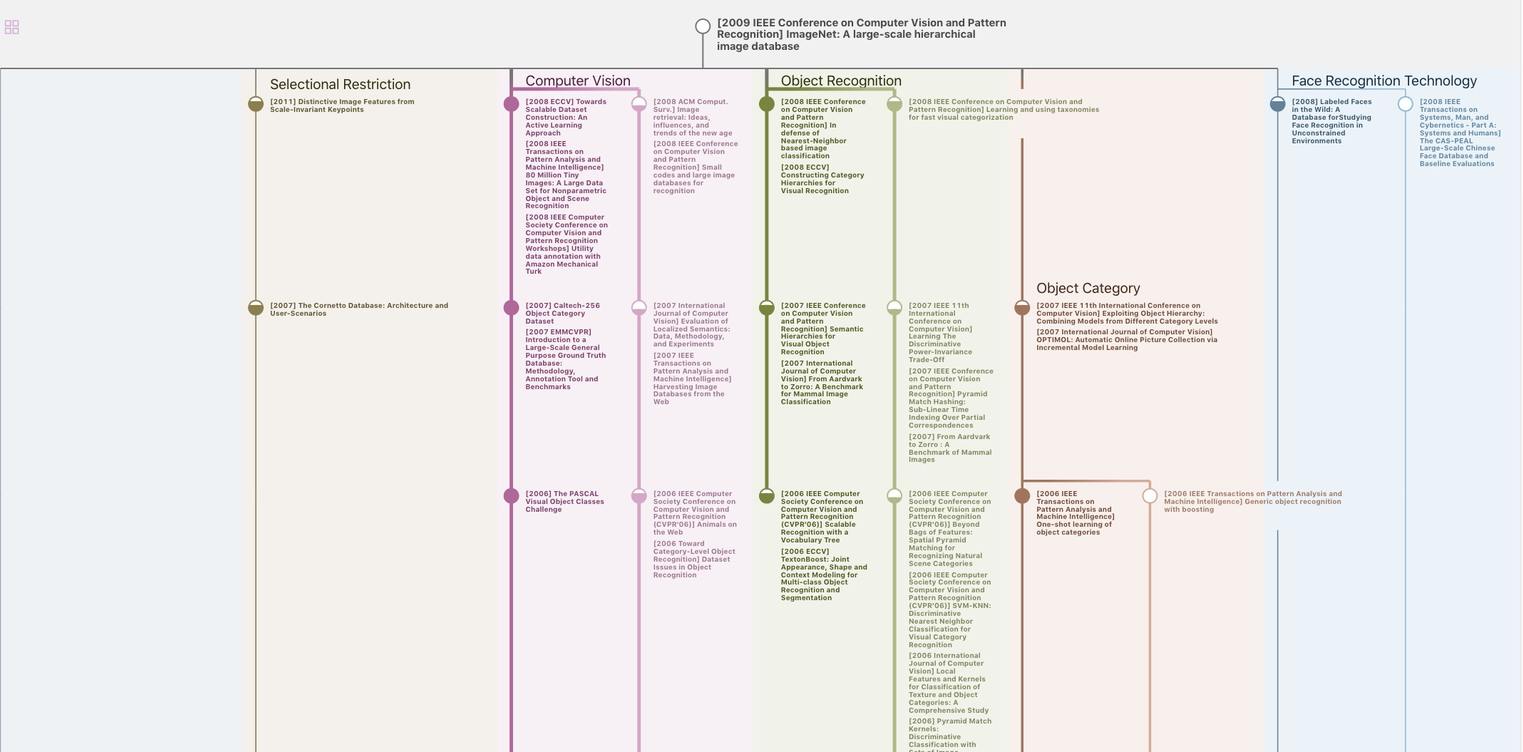
生成溯源树,研究论文发展脉络
Chat Paper
正在生成论文摘要