Selective Sampling Using Active Learning For Short-Term Wind Speed Prediction
2017 29TH CHINESE CONTROL AND DECISION CONFERENCE (CCDC)(2017)
摘要
Wind power prediction is very important to guarantee security and stability of the wind farm and power system operation, and wind speed forecasting is the key to wind power prediction. Due to dramatic changes and shorter collection intervals in wind speed, it generates a larger numbers of samples, which affects modeling time and accuracy. Therefore, a short-term wind speed prediction method based on active learning with sample selection is proposed. The main objective of active learning is to collect training samples by minimizing the error of the prediction process while minimizing the number of training samples used. First the initial training set is selected, then selecting the sample with higher Euclidean distance adding to the training set and delete the samples with lower cross-validation error. Finally, the establishment of support vector machine model and neural network model to predict the results of comparison. Taking a wind farm in Jiangsu Province as the actual experimental data, simulation results show that this method can ensure the prediction accuracy, while effectively reducing the number of training samples.
更多查看译文
关键词
Short-term Wind Speed Prediction, Active Learning, SVM, ANN
AI 理解论文
溯源树
样例
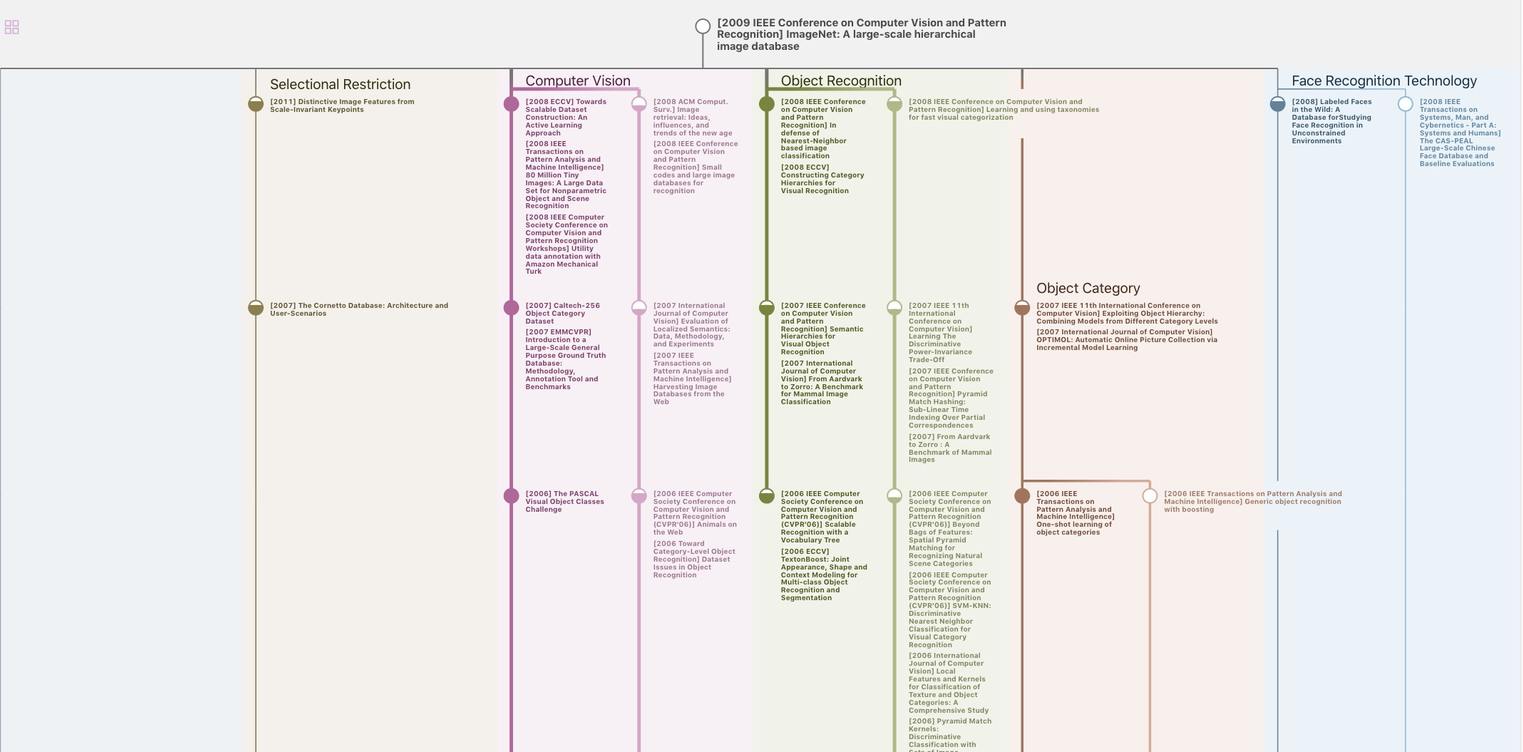
生成溯源树,研究论文发展脉络
Chat Paper
正在生成论文摘要