A discriminative model selection approach and its application to text classification
Neural Computing and Applications(2017)
摘要
Classification is one of the fundamental problems in data mining, in which a classification algorithm attempts to construct a classifier from a given set of training instances with class labels. It is well known that some classification algorithms perform very well on some domains, and poorly on others. For example, NB performs well on some domains, and poorly on others that involve correlated features. C4.5, on the other hand, typically works better than NB on such domains. To integrate their advantages and avoid their disadvantages, many model hybrid approaches, such as model insertion and model combination, are proposed. In this paper, we focus on a novel view and propose a discriminative model selection approach, called discriminative model selection (DMS). DMS discriminatively chooses different single models for different test instances and retains the interpretability of single models. Empirical studies on a collection of 36 classification problems from the University of California at Irvine repository show that our discriminative model selection approach outperforms single models, model insertion approaches and model combination approaches. Besides, we apply the proposed discriminative model selection approach to some state-of-the-art naive Bayes text classifiers and also improve their performance.
更多查看译文
关键词
Naive Bayes,C4.5,Discriminative model selection,Text classification
AI 理解论文
溯源树
样例
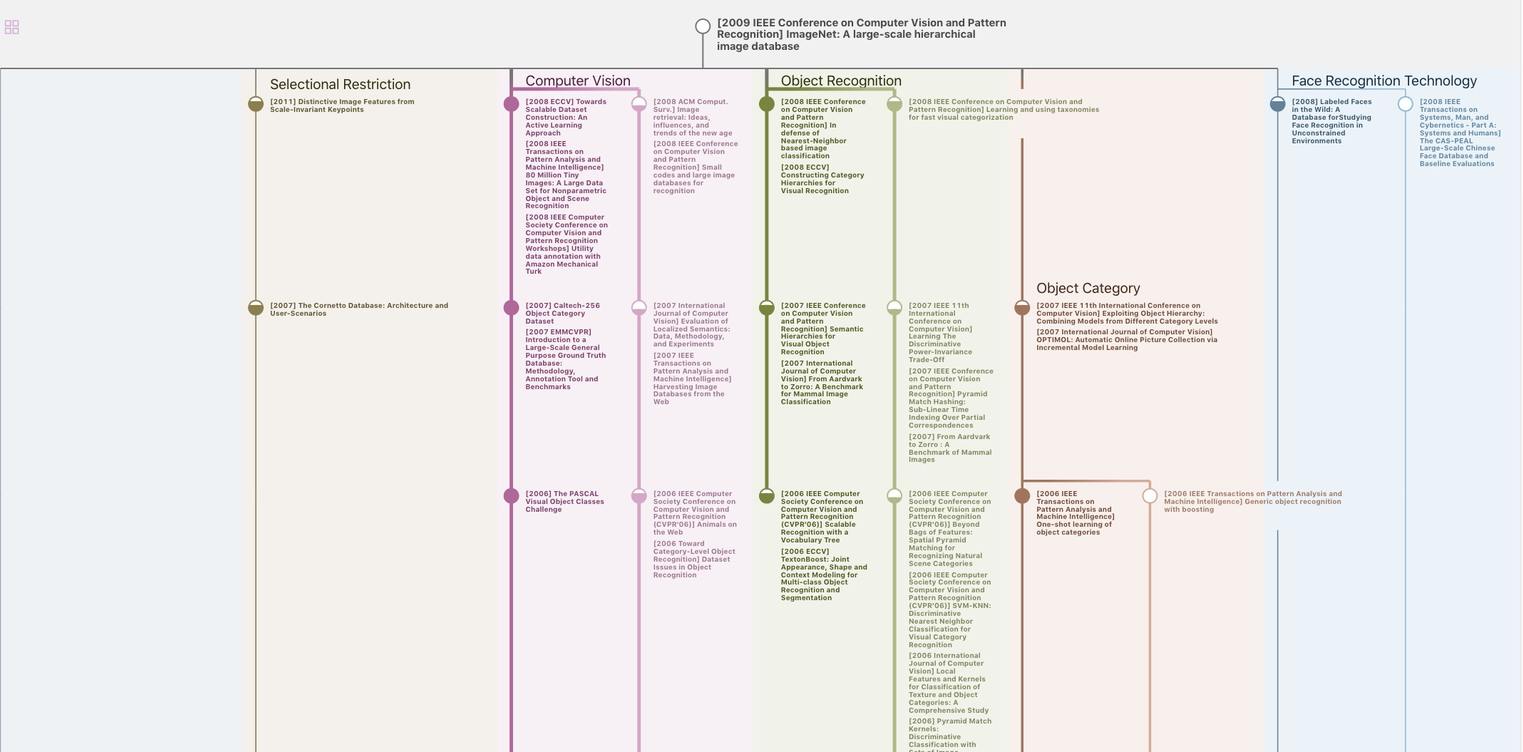
生成溯源树,研究论文发展脉络
Chat Paper
正在生成论文摘要