Effective Condition Number Bounds for Convex Regularization
IEEE Transactions on Information Theory(2020)
摘要
We derive bounds relating Renegar’s condition number to quantities that govern the statistical performance of convex regularization in settings that include the
$\ell _{1}$
-analysis setting. Using results from conic integral geometry, we show that the bounds can be made to depend only on a random projection, or restriction, of the analysis operator to a lower dimensional space, and can still be effective if these operators are ill-conditioned. As an application, we get new bounds for the undersampling phase transition of composite convex regularizers. Key tools in the analysis are Slepian’s inequality and the kinematic formula from integral geometry.
更多查看译文
关键词
Convex regularization,compressed sensing,integral geometry,convex optimization,dimension reduction
AI 理解论文
溯源树
样例
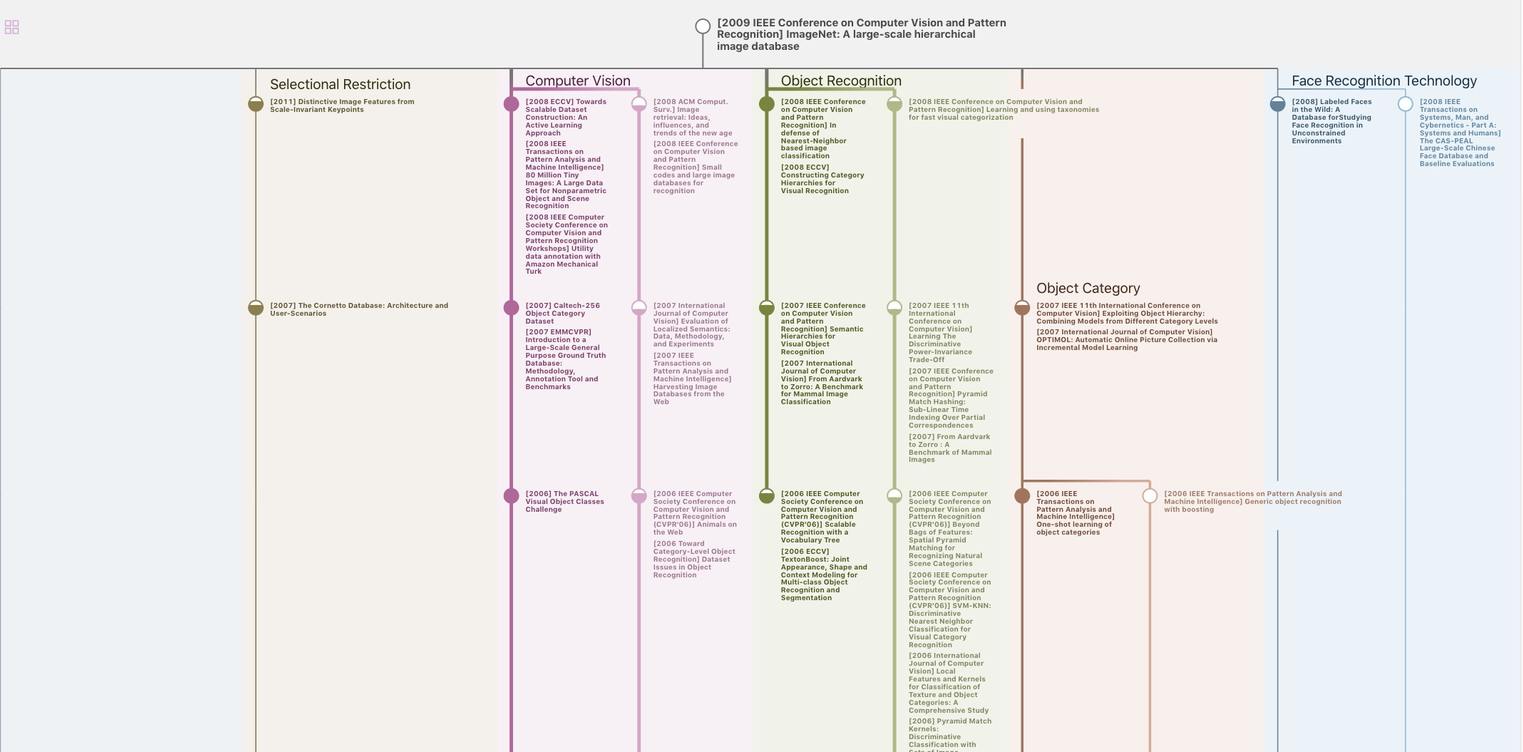
生成溯源树,研究论文发展脉络
Chat Paper
正在生成论文摘要