An adaptive data-driven method for accurate prediction of remaining useful life of rolling bearings
Frontiers in Mechanical Engineering(2017)
摘要
A novel data-driven method based on Gaussian mixture model (GMM) and distance evaluation technique (DET) is proposed to predict the remaining useful life (RUL) of rolling bearings. The data sets are clustered by GMM to divide all data sets into several health states adaptively and reasonably. The number of clusters is determined by the minimum description length principle. Thus, either the health state of the data sets or the number of the states is obtained automatically. Meanwhile, the abnormal data sets can be recognized during the clustering process and removed from the training data sets. After obtaining the health states, appropriate features are selected by DET for increasing the classification and prediction accuracy. In the prediction process, each vibration signal is decomposed into several components by empirical mode decomposition. Some common statistical parameters of the components are calculated first and then the features are clustered using GMM to divide the data sets into several health states and remove the abnormal data sets. Thereafter, appropriate statistical parameters of the generated components are selected using DET. Finally, least squares support vector machine is utilized to predict the RUL of rolling bearings. Experimental results indicate that the proposed method reliably predicts the RUL of rolling bearings.
更多查看译文
关键词
Gaussian mixture model,distance evaluation technique,health state,remaining useful life,rolling bearing
AI 理解论文
溯源树
样例
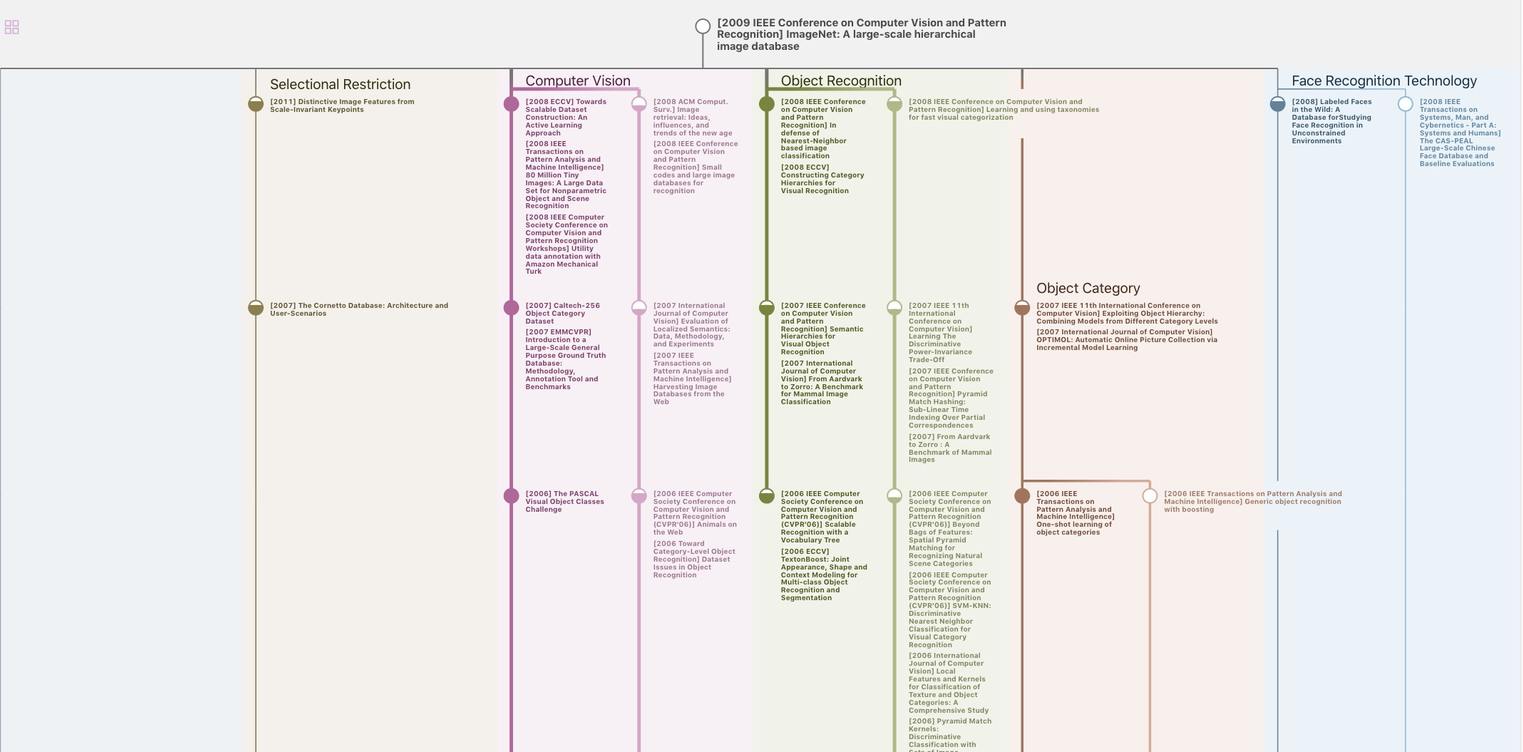
生成溯源树,研究论文发展脉络
Chat Paper
正在生成论文摘要