WITHDRAWN: Parallel Block Coordinate Descent with Path-Following for Constrained Folded Concave Linear Regression
Journal of Computational Science(2017)
摘要
Abstract This paper focuses on solving folded concave penalized linear regression with inequality constraints, which is one of the typical optimization problems of minimizing the sum of a smooth (possibly nonconvex nonlinear) function and a block-separable nonconvex nonsmooth function involving a large number of block variables subject to inequality constraints. Recent theoretical analysis has demonstrated the superiority of the solution of this problem over those obtained from its convex counterparts in statistics. However, solving such a large-scale nonconvex nonsmooth optimization problem associated with inequality constraints remains a big challenge. To this end, we propose a new parallel first-order optimization method, noted as Parallel Block Coordinate Descent with Path-Following (PBCD-PF), which combines the classical path-following method and the recent popular block coordinate descent method in a shared memory system. Experiments conducted on constrained folded concave penalized linear regression and the parallel experiment results of the proposed algorithm validate the proposed algorithmu0027s efficacy as well as scalability.
更多查看译文
AI 理解论文
溯源树
样例
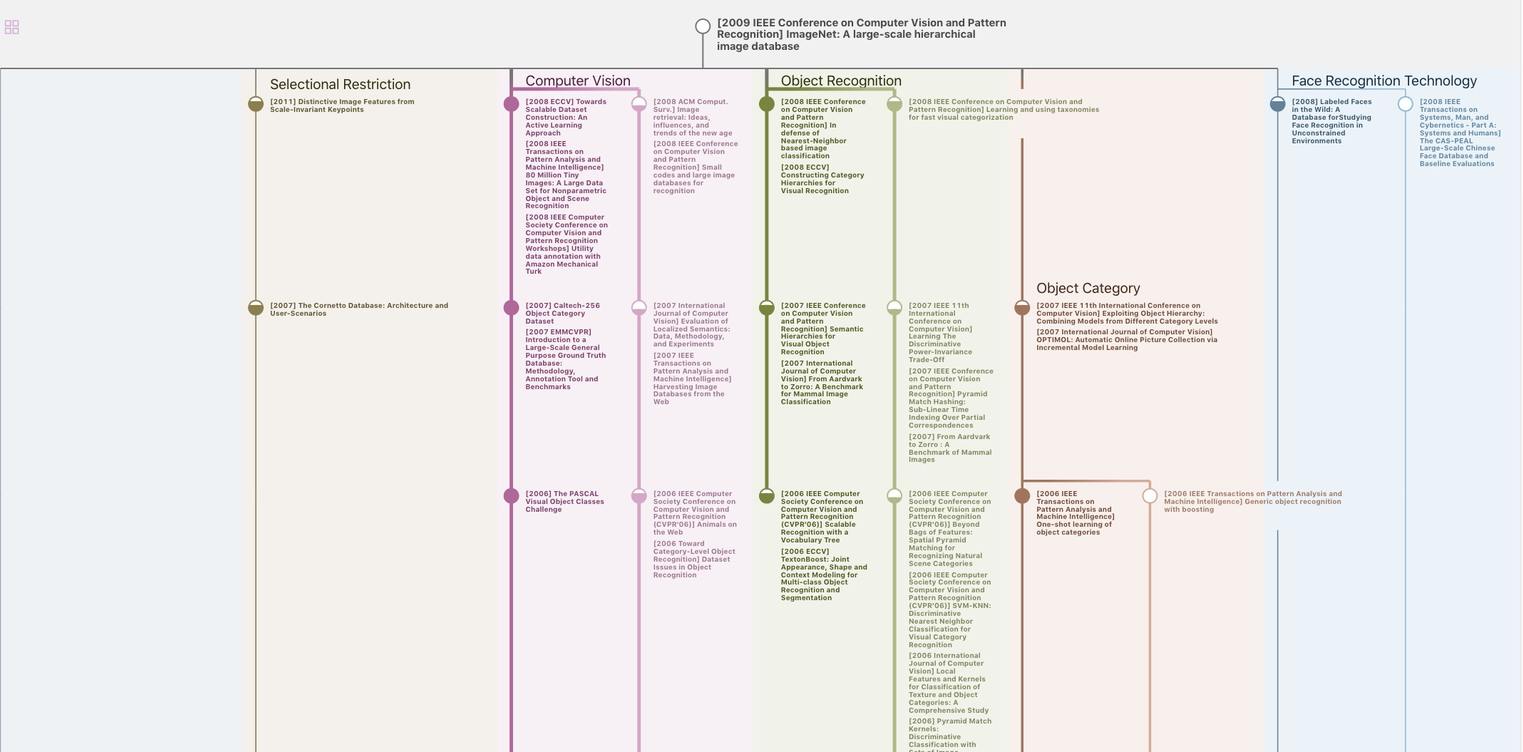
生成溯源树,研究论文发展脉络
Chat Paper
正在生成论文摘要