Novel Geometric Area Analysis Technique for Anomaly Detection using Trapezoidal Area Estimation on Large-Scale Networks
IEEE Transactions on Big Data(2019)
摘要
The prevalence of interconnected appliances and ubiquitous computing face serious threats from the hostile activities of network attackers. Conventional Intrusion Detection Systems (IDSs) are incapable of detecting these intrusive events as their outcomes reflect high false positive rates (FPRs). In this paper, we present a novel Geometric Area Analysis (GAA) technique based on Trapezoidal Area Estimation (TAE) for each observation computed from the parameters of the Beta Mixture Model (BMM) for features and the distances between observations. As this GAA-based detection depends on the methodology of anomaly-based detection (ADS), it constructs the areas of normal observations in a normal profile with those of the testing set estimated from the same parameters to recognise abnormal patterns. We also design a scalable framework for handling large-scale networks, and our GAA technique considers a decision engine module in this framework. The performance of our GAA technique is evaluated using the NSL-KDD and UNSW-NB15 datasets. To reduce the high-dimensional data of network connections, we apply the Principal Component Analysis (PCA) and evaluate its influence on the GAA technique. The empirical results show that our technique achieves a higher detection rate and lower FPR with a lower processing time than other competing methods.
更多查看译文
关键词
Gallium arsenide,Principal component analysis,Big Data,Feature extraction,Estimation,Security,Computers
AI 理解论文
溯源树
样例
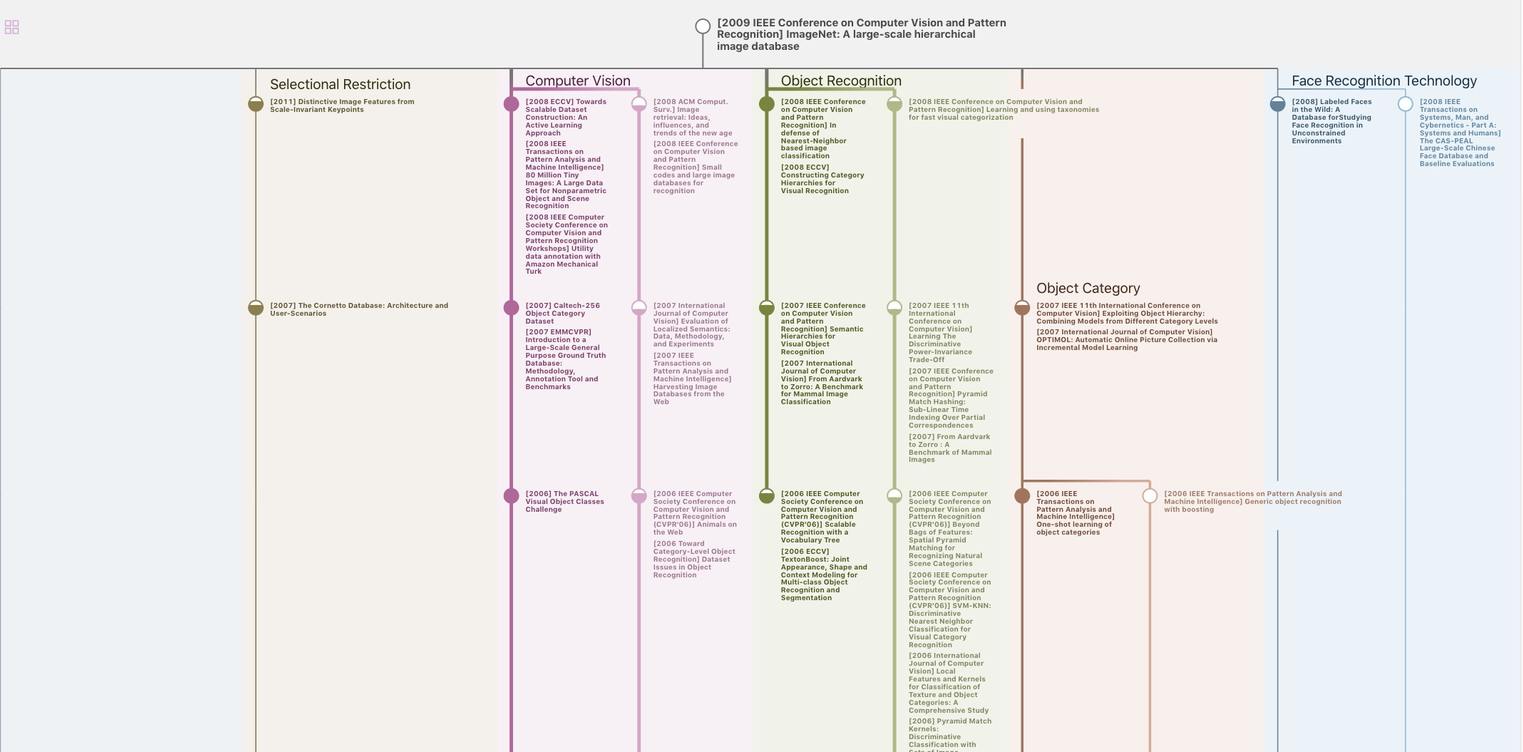
生成溯源树,研究论文发展脉络
Chat Paper
正在生成论文摘要