Contextual Autocomplete: A Novel User Interface Using Machine Learning to Improve Ontology Usage and Structured Data Capture for Presenting Problems in the Emergency Department
bioRxiv(2017)
摘要
Objective To determine the effect of contextual autocomplete, a user interface that uses machine learning, on the efficiency and quality of documentation of presenting problems (chief complaints) in the emergency department (ED).Materials and Method We used contextual autocomplete, a user interface that ranks concepts by their predicted probability, to help nurses enter data about a patient9s reason for visiting the ED. Predicted probabilities were calculated using a previously derived model based on triage vital signs and a brief free text note. We evaluated the percentage and quality of structured data captured using a prospective before-and-after study design.Results A total of 279,231 patient encounters were analyzed. Structured data capture improved from 26.2% to 97.2% (pu003c0.0001). During the post-implementation period, presenting problems were more complete (3.35 vs 3.66; p=0.0004), as precise (3.59 vs. 3.74; p=0.1), and higher in overall quality (3.38 vs. 3.72; p=0.0002). Our system reduced the mean number of keystrokes required to document a presenting problem from 11.6 to 0.6 (pu003c0.0001), a 95% improvement.Discussion We have demonstrated a technique that captures structured data on nearly all patients. We estimate that our system reduces the number of man-hours required annually to type presenting problems at our institution from 92.5 hours to 4.8 hours.Conclusion Implementation of a contextual autocomplete system resulted in improved structured data capture, ontology usage compliance, and data quality.
更多查看译文
关键词
Autocomplete,Contextual Autocomplete,Ontology,Structured Data,Emergency Medicine,Machine Learning
AI 理解论文
溯源树
样例
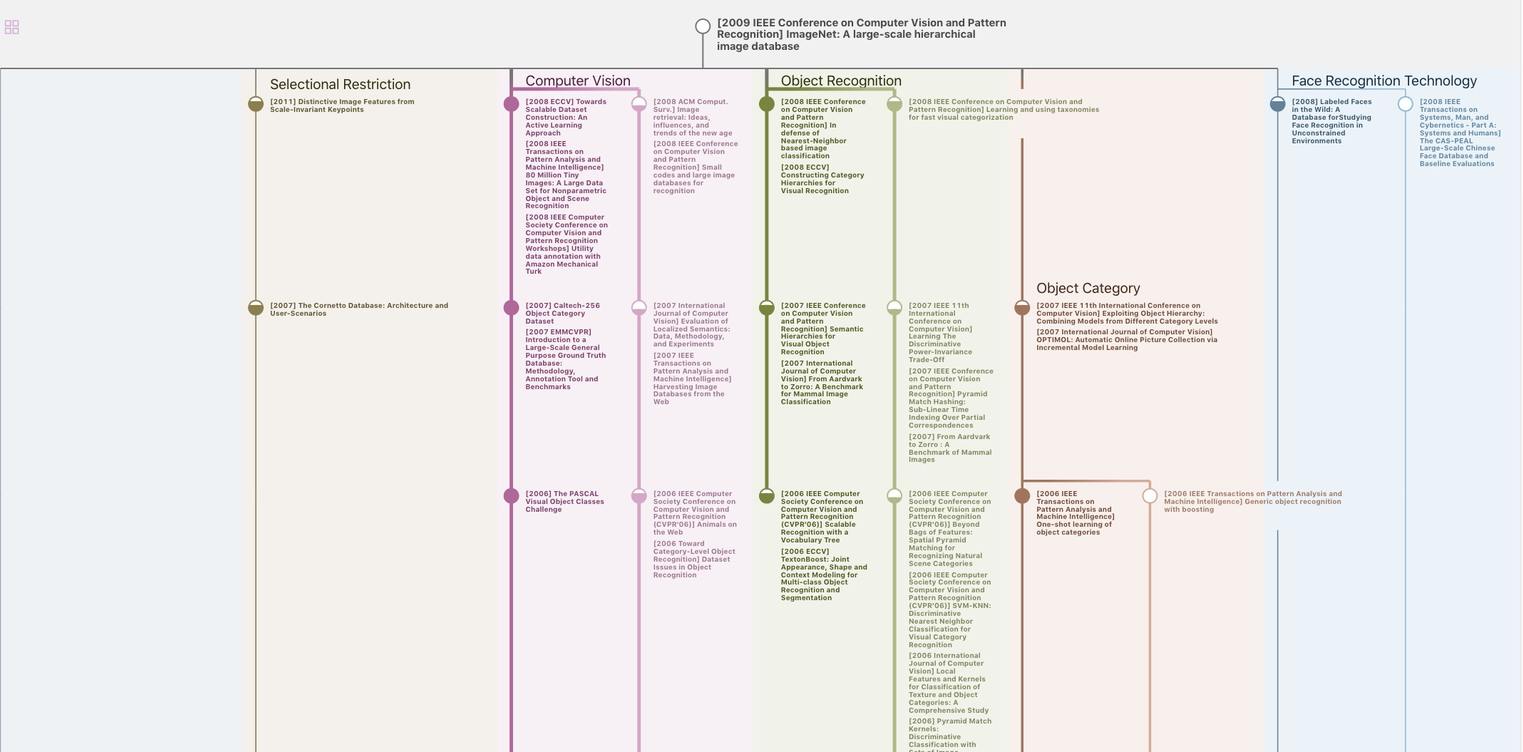
生成溯源树,研究论文发展脉络
Chat Paper
正在生成论文摘要