Utilization-based object recognition in confined spaces
Proceedings of SPIE(2017)
摘要
Recognizing substantially occluded objects in confined spaces is a very challenging problem for ground-based persistent surveillance systems. In this paper, we discuss the ontology inference of occluded object recognition in the context of in-vehicle group activities (IVGA) and describe an approach that we refer to as utilization-based object recognition method. We examine the performance of three types of classifiers tailored for the recognition of objects with partial visibility, namely, (1) Hausdorff Distance classifier, (2) Hamming Network classifier, and (3) Recurrent Neural Network classifier. In order to train these classifiers, we have generated multiple imagery datasets containing a mixture of common objects appearing inside a vehicle with full or partial visibility and occultation. To generate dynamic interactions between multiple people, we model the IVGA scenarios using a virtual simulation environment, in which a number of simulated actors perform a variety of IVGA tasks independently or jointly. This virtual simulation engine produces the much needed imagery datasets for the verification and validation of the efficiency and effectiveness of the selected object recognizers. Finally, we improve the performance of these object recognizers by incorporating human gestural information that differentiates various object utilization or handling methods through the analyses of dynamic human-object interactions (HOI), human-human interactions (HHI), and human-vehicle interactions (HVI) in the context of IVGA.
更多查看译文
关键词
Utilization-based Object Recognition,Ground-based Surveillance Systems,In-Vehicle Group Activity,Human-Object Interactions,Human-Human Interactions,and Human-Vehicle Interactions,Virtual Environment Simulation Modeling
AI 理解论文
溯源树
样例
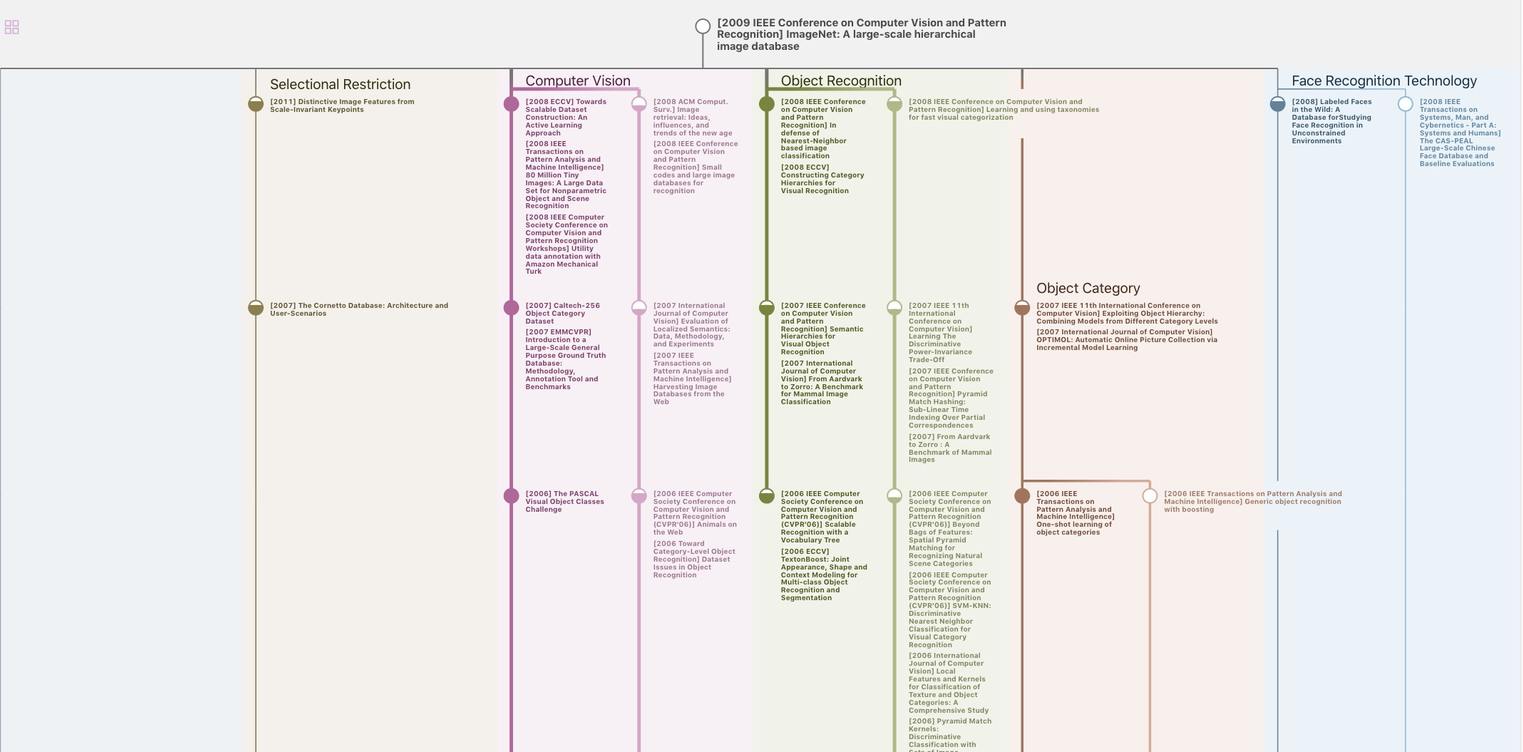
生成溯源树,研究论文发展脉络
Chat Paper
正在生成论文摘要