Semantic Analysis of Open Source Data for Syndromic Surveillance
Online Journal of Public Health Informatics(2017)
摘要
Objective The objective of this analysis is to leverage recent advances in natural language processing (NLP) to develop new methods and system capabilities for processing social media (Twitter messages) for situational awareness (SA), syndromic surveillance (SS), and event-based surveillance (EBS). Specifically, we evaluated the use of human-in-the-loop semantic analysis to assist public health (PH) SA stakeholders in SS and EBS using massive amounts of publicly available social media data. Introduction Social media messages are often short, informal, and ungrammatical. They frequently involve text, images, audio, or video, which makes the identification of useful information difficult. This complexity reduces the efficacy of standard information extraction techniques 1 . However, recent advances in NLP, especially methods tailored to social media 2 , have shown promise in improving real-time PH surveillance and emergency response 3 . Surveillance data derived from semantic analysis combined with traditional surveillance processes has potential to improve event detection and characterization. The CDC Office of Public Health Preparedness and Response (OPHPR), Division of Emergency Operations (DEO) and the Georgia Tech Research Institute have collaborated on the advancement of PH SA through development of new approaches in using semantic analysis for social media. Methods To understand how computational methods may benefit SS and EBS, we studied an iterative refinement process, in which the data user actively cultivated text-based topics (“semantic culling”) in a semi-automated SS process. This ‘human-in-the-loop’ process was critical for creating accurate and efficient extraction functions in large, dynamic volumes of data. The general process involved identifying a set of expert-supplied keywords, which were used to collect an initial set of social media messages. For purposes of this analysis researchers applied topic modeling to categorize related messages into clusters. Topic modeling uses statistical techniques to semantically cluster and automatically determine salient aggregations. A user then semantically culled messages according to their PH relevance. In June 2016, researchers collected 7,489 worldwide English- language Twitter messages (tweets) and compared three sampling methods: a baseline random sample (C1, n=2700), a keyword-based sample (C2, n=2689), and one gathered after semantically culling C2 topics of irrelevant messages (C3, n=2100). Researchers utilized a software tool, Luminoso Compass 4 , to sample and perform topic modeling using its real-time modeling and Twitter integration features. For C2 and C3, researchers sampled tweets that the Luminoso service matched to both clinical and layman definitions of Rash, Gastro-Intestinal syndromes 5 , and Zika-like symptoms. Layman terms were derived from clinical definitions from plain language medical thesauri. ANOVA statistics were calculated using SPSS software, version. Post-hoc pairwise comparisons were completed using ANOVA Turkey’s honest significant difference (HSD) test. Results An ANOVA was conducted, finding the following mean relevance values: 3% (+/- 0.01%), 24% (+/- 6.6%) and 27% (+/- 9.4%) respectively for C1, C2, and C3. Post-hoc pairwise comparison tests showed the percentages of discovered messages related to the event tweets using C2 and C3 methods were significantly higher than for the C1 method (random sampling) (pu003c0.05). This indicates that the human-in-the-loop approach provides benefits in filtering social media data for SS and ESB; notably, this increase is on the basis of a single iteration of semantic culling; subsequent iterations could be expected to increase the benefits. Conclusions This work demonstrates the benefits of incorporating non- traditional data sources into SS and EBS. It was shown that an NLP- based extraction method in combination with human-in-the-loop semantic analysis may enhance the potential value of social media (Twitter) for SS and EBS. It also supports the claim that advanced analytical tools for processing non-traditional SA, SS, and EBS sources, including social media, have the potential to enhance disease detection, risk assessment, and decision support, by reducing the time it takes to identify public health events.
更多查看译文
AI 理解论文
溯源树
样例
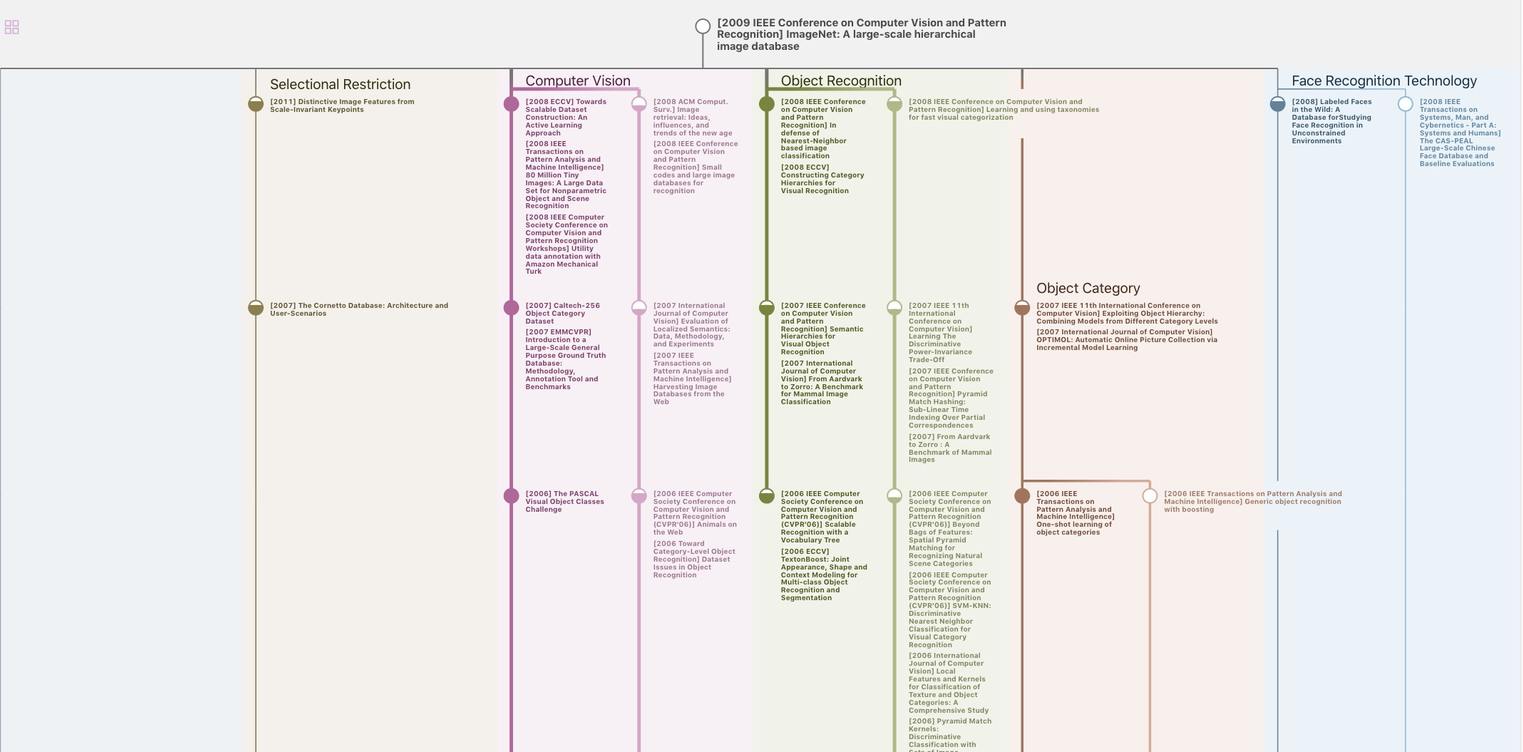
生成溯源树,研究论文发展脉络
Chat Paper
正在生成论文摘要