Quantifying multi-source uncertainties in multi-model predictions using the Bayesian model averaging scheme
HYDROLOGY RESEARCH(2018)
摘要
This study focuses on a quantitative multi-source uncertainty analysis of multi-model predictions. Three widely used hydrological models, i.e., Xinanjiang (XAJ), hybrid rainfall-runoff (HYB), and HYMOD (HYM), were calibrated by two parameter optimization algorithms, namely, shuffled complex evolution (SCE-UA) method and shuffled complex evolution metropolis (SCEM-UA) method on the Mishui basin, south China. The input uncertainty was quantified by utilizing a normally distributed error multiplier. The ensemble simulation sets calculated from the three models were combined using the Bayesian model averaging (BMA) method. Results indicate the following. (1) Both SCE-UA and SCEM-UA resulted in good and comparable streamflow simulations. Specifically, the SCEM-UA implied parameter uncertainty and provided the posterior distribution of the parameters. (2) In terms of the precipitation input uncertainty, precision of streamflow simulations did not improve remarkably. (3) The BMA combination not only improved the precision of streamflow prediction, but also quantified the uncertainty bounds of the simulation. (4) The prediction interval calculated using the SCEM-UA-based BMA combination approach appears superior to that calculated using the SCE-UA-based BMA combination for both high flows and low flows. Results suggest that the comprehensive uncertainty analysis by using the SCEM-UA algorithm and BMA method is superior for streamflow predictions and flood forecasting.
更多查看译文
关键词
Bayesian model averaging,ensemble,hydrological prediction,parameter optimization,uncertainty analysis
AI 理解论文
溯源树
样例
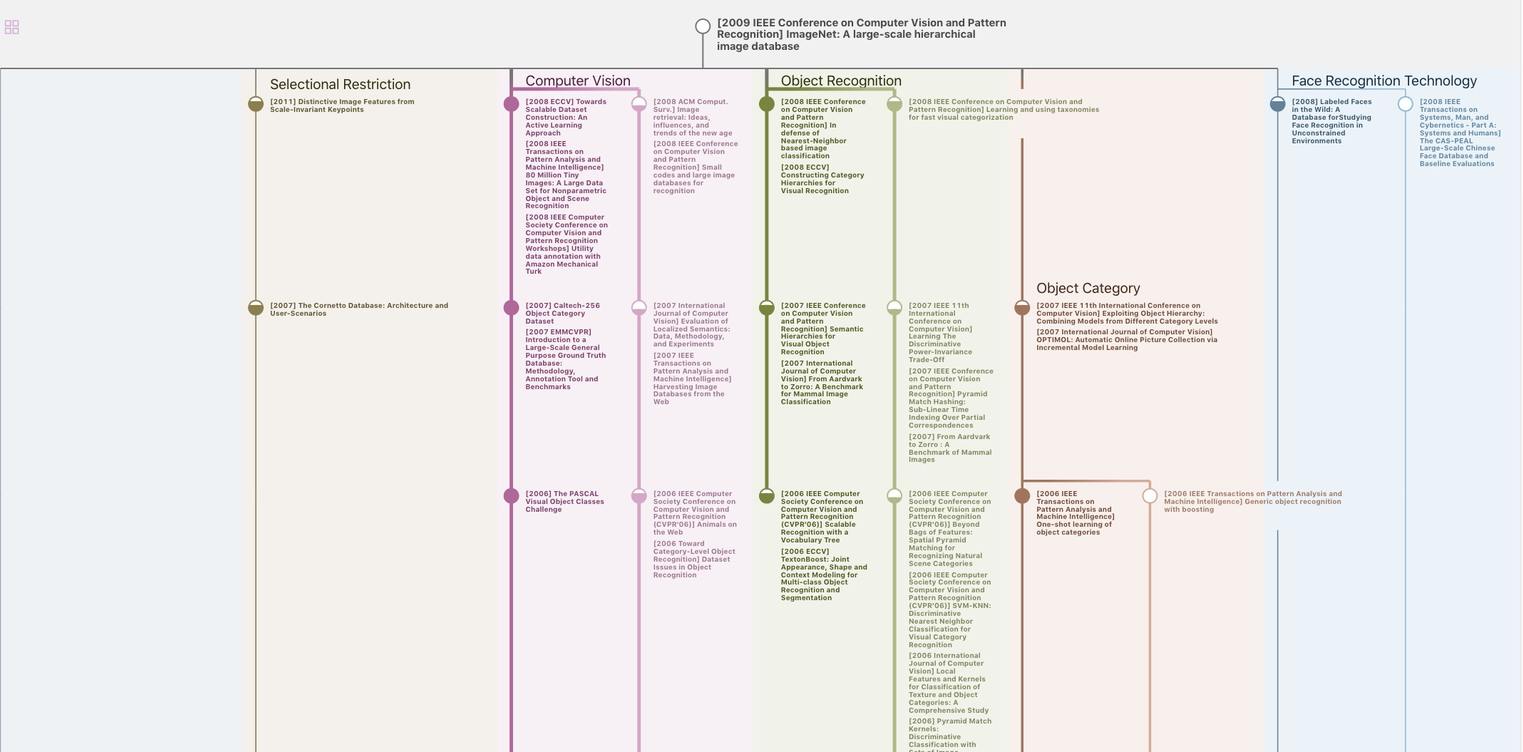
生成溯源树,研究论文发展脉络
Chat Paper
正在生成论文摘要